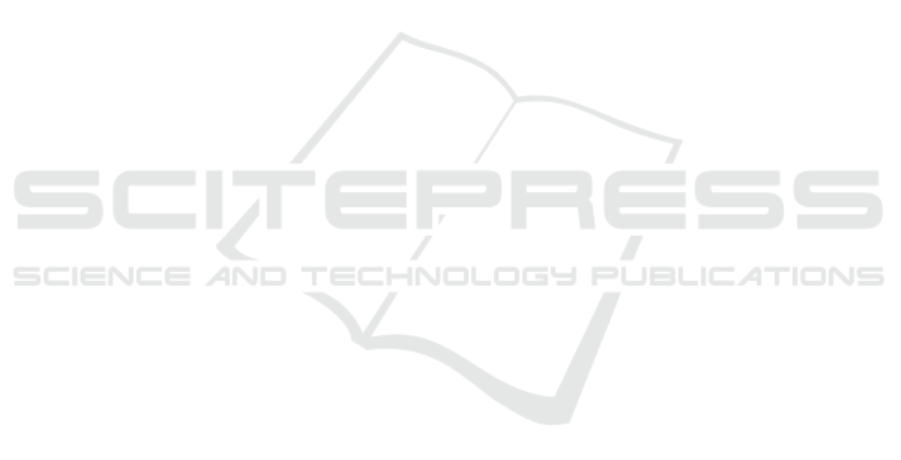
REFERENCES
Basugi, A., Ohira, Y., and Ikusaka, M. (2011). Comparison
of the outpatient diagnostic process between novice
and trained residents. General medicine, 12(1):35–41.
Beam, A. L. and Kohane, I. S. (2018). Big data and machine
learning in health care. Jama, 319(13):1317–1318.
Chen, M., Hao, Y., Hwang, K., Wang, L., and Wang, L.
(2017). Disease prediction by machine learning over
big data from healthcare communities. Ieee Access,
5:8869–8879.
Choi, E., Bahadori, M. T., Schuetz, A., Stewart, W. F., and
Sun, J. (2016a). Doctor ai: Predicting clinical events
via recurrent neural networks. In Machine Learning
for Healthcare Conference, pages 301–318.
Choi, E., Schuetz, A., Stewart, W. F., and Sun, J. (2016b).
Using recurrent neural network models for early de-
tection of heart failure onset. Journal of the American
Medical Informatics Association, 24(2):361–370.
Cuthbert, L., Duboulay, B., Teather, D., Teather, B.,
Sharples, M., and Duboulay, G. (1999). Expert/novice
differences in diagnostic medical cognition-a review
of the literature.
Detrano, R., Janosi, A., Steinbrunn, W., Pfisterer, M.,
Schmid, J.-J., Sandhu, S., Guppy, K. H., Lee, S., and
Froelicher, V. (1989). International application of a
new probability algorithm for the diagnosis of coro-
nary artery disease. The American journal of cardiol-
ogy, 64(5):304–310.
Guo, T., Xu, Z., Yao, X., Chen, H., Aberer, K., and Funaya,
K. (2016). Robust online time series prediction with
recurrent neural networks. In 2016 IEEE International
Conference on Data Science and Advanced Analytics
(DSAA), pages 816–825. Ieee.
Haq, A. U., Li, J. P., Memon, M. H., Nazir, S., and Sun,
R. (2018). A hybrid intelligent system framework for
the prediction of heart disease using machine learning
algorithms. Mobile Information Systems, 2018.
Kannel, W. B., Castelli, W. P., Gordon, T., and McNamara,
P. M. (1971). Serum cholesterol, lipoproteins, and the
risk of coronary heart disease: the framingham study.
Annals of internal medicine, 74(1):1–12.
Lemaire, J. B., Miller, E. N., Polachek, A. J., and Wong, H.
(2019). Stakeholder groups unique perspectives about
the attending physician preceptor role: A qualitative
study. Journal of general internal medicine, pages 1–
9.
Liu, J., Zhang, Z., and Razavian, N. (2018). Deep ehr:
Chronic disease prediction using medical notes. arXiv
preprint arXiv:1808.04928.
Meyer, A., Zverinski, D., Pfahringer, B., Kempfert, J.,
Kuehne, T., S
¨
undermann, S. H., Stamm, C., Hofmann,
T., Falk, V., and Eickhoff, C. (2018). Machine learn-
ing for real-time prediction of complications in criti-
cal care: a retrospective study. The Lancet Respiratory
Medicine, 6(12):905–914.
Michtalik, H. J., Yeh, H.-C., Pronovost, P. J., and Brotman,
D. J. (2013). Impact of attending physician workload
on patient care: a survey of hospitalists. JAMA inter-
nal medicine, 173(5):375–377.
Miotto, R., Li, L., Kidd, B. A., and Dudley, J. T. (2016).
Deep patient: an unsupervised representation to pre-
dict the future of patients from the electronic health
records. Scientific reports, 6:26094.
Shickel, B., Tighe, P. J., Bihorac, A., and Rashidi, P. (2017).
Deep ehr: a survey of recent advances in deep learning
techniques for electronic health record (ehr) analysis.
IEEE journal of biomedical and health informatics,
22(5):1589–1604.
Street, W. N., Mangasarian, O. L., and Wolberg, W. H.
(1995). An inductive learning approach to prognostic
prediction. In Machine Learning Proceedings 1995,
pages 522–530. Elsevier.
Sun, D., Wang, M., and Li, A. (2019). A multimodal
deep neural network for human breast cancer progno-
sis prediction by integrating multi-dimensional data.
IEEE/ACM Transactions on Computational Biology
and Bioinformatics (TCBB), 16(3):841–850.
Zhang, S., Xie, P., Wang, D., and Xing, E. P. (2017). Medi-
cal diagnosis from laboratory tests by combining gen-
erative and discriminative learning. arXiv preprint
arXiv:1711.04329.
Zhu, Z., Zheng, Z., Zhang, F., Wu, Y., Trzaskowski, M.,
Maier, R., Robinson, M. R., McGrath, J. J., Visscher,
P. M., Wray, N. R., et al. (2018). Causal associations
between risk factors and common diseases inferred
from gwas summary data. Nature communications,
9(1):224.
ICPRAM 2020 - 9th International Conference on Pattern Recognition Applications and Methods
620