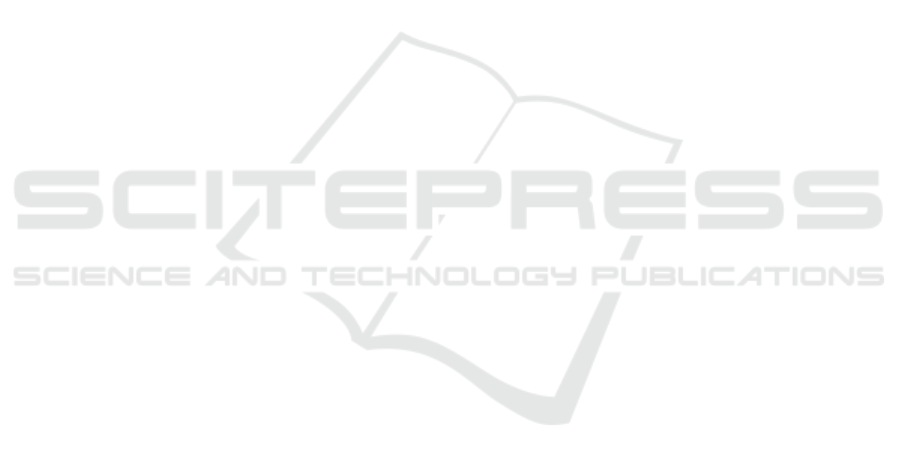
Journal of Information & Knowledge Management,
13(3).
Abdellatif, S., Ben Hassine, M. A., and Ben Yahia, S.
(2019). Novel interestingness measures for mining
significant association rules from imbalanced data. In
Web, Artificial Intelligence and Network Applications,
pages 172–182.
Abdellatif, S., Ben Hassine, M. A., Ben Yahia, S., and
Bouzeghoub, A. (2018a). ARCID: A new approach
to deal with imbalanced datasets classification. In
SOFSEM: Theory and Practice of Computer Science,
pages 569–580.
Abdellatif, S., Yahia, S. B., Hassine, M. A. B., and
Bouzeghoub, A. (2018b). Fuzzy aggregation for rule
selection in imbalanced datasets classification using
choquet integral. In IEEE International Conference
on Fuzzy Systems, page 7p.
Alwidian, J., Hammo, B. H., and Obeid, N. (2018). WCBA:
Weighted classification based on association rules al-
gorithm for breast cancer disease. Applied Soft Com-
puting, 62:536–549.
Azevedo, P. J. and Jorge, A. M. (2007). Comparing rule
measures for predictive association rules. In Machine
Learning: ECML, pages 510–517.
Bong, K. K., Joest, M., Quix, C., Anwar, T., and Manickam,
S. (2014). Selection and aggregation of interestingnes
measures: A review. Journal of Theoretical and Ap-
plied Information Technology, 59(1):146–166.
Bouker, S., Saidi, R., Yahia, S. B., and Nguifo, E. M.
(2014). Mining undominated association rules
through interestingness measures. International Jour-
nal on Artificial Intelligence Tools, 23(4):22p.
Coenen, F. (2004). LUCS KDD implementation of
CBA. http://cgi.csc.liv.ac.uk/
∼
frans/KDD/Software/
CBA/cba.html.
Dahbi, A., Jabri, S., Balouki, Y., and Gadi, T. (2016). A
new method for ranking association rules with multi-
ple criteria based on dominance relation. In ACS/IEEE
13th International Conference of Computer Systems
and Applications, page 7p.
Fayyad, U. M. and Irani, K. B. (1993). Multi-interval dis-
cretization of continuous-valued attributes for classifi-
cation learning. In International Joint Conference on
Artificial Intelligence, pages 1022–1029.
Jalali-Heravi, M. and Za
¨
ıane, O. R. (2010). A study on
interestingness measures for associative classifiers. In
Proceedings of the 2010 ACM Symposium on Applied
Computing, pages 1039–1046.
Kannan, S. (2010). An Integration of Association Rules and
Classification: An Empirical Analysis. PhD thesis,
Madurai Kamaraj University.
Liu, B., Hsu, W., and Ma, Y. (1998). Integrating classifi-
cation and association rule mining. In Proceedings of
the 4th International Conference on Knowledge Dis-
covery and Data Mining, pages 80–86.
Moreno, M. N., Segrera, S., L
´
opez, V. F., Mu
˜
noz, M. D.,
and S
´
anchez, A. L. (2016). Web mining based frame-
work for solving usual problems in recommender sys-
tems. A case study for movies’ recommendation. Neu-
rocomputing, 176:72–80.
Nandhini, M., Sivanandam, S. N., Rajalakshmi, M., and
Sidheswaran, D. (2015). Enhancing the spam email
classification accuracy using post processing tech-
niques. International Journal of Applied Engineering
Research, 10(15):35125–35130.
Nguyen Le, T. T., Huynh, H. X., and Guillet, F. (2009).
Finding the most interesting association rules by
aggregating objective interestingness measures. In
Knowledge Acquisition: Approaches, Algorithms and
Applications, pages 40–49.
Shao, Y., Liu, B., Li, G., and Wang, S. (2017). Software
defect prediction based on class-association rules. In
2nd International Conference on Reliability Systems
Engineering, page 5p.
Silva, M. F. and Carvalho, V. O. (2018). Aggregating inter-
estingness measures in associative classifiers. In Anais
do XV Encontro Nacional de Intelig
ˆ
encia Artificial e
Computacional, pages 70–81.
Singh, J., Kamra, A., and Singh, H. (2016). Prediction of
heart diseases using associative classification. In 5th
International Conference on Wireless Networks and
Embedded Systems, page 7p.
Tew, C., Giraud-Carrier, C., Tanner, K., and Burton, S.
(2014). Behavior-based clustering and analysis of
interestingness measures for association rule mining.
Data Mining and Knowledge Discovery, 28(4):1004–
1045.
Thabtah, F. (2007). A review of associative classification
mining. Knowledge Engineering Review, 22(1):37–
65.
Yang, G. and Cui, X. (2015). A study of interestingness
measures for associative classification on imbalanced
data. In Trends and Applications in Knowledge Dis-
covery and Data Mining, pages 141–151.
Yang, G., Shimada, K., Mabu, S., and Hirasawa, K. (2009).
A nonlinear model to rank association rules based on
semantic similarity and genetic network programing.
IEEJ Transactions on Electrical and Electronic Engi-
neering, 4(2):248–256.
Yin, C., Guo, Y., Yang, J., and Ren, X. (2018). A new
recommendation system on the basis of consumer ini-
tiative decision based on an associative classification
approach. Industrial Management and Data Systems,
118(1):188–203.
ICEIS 2020 - 22nd International Conference on Enterprise Information Systems
90