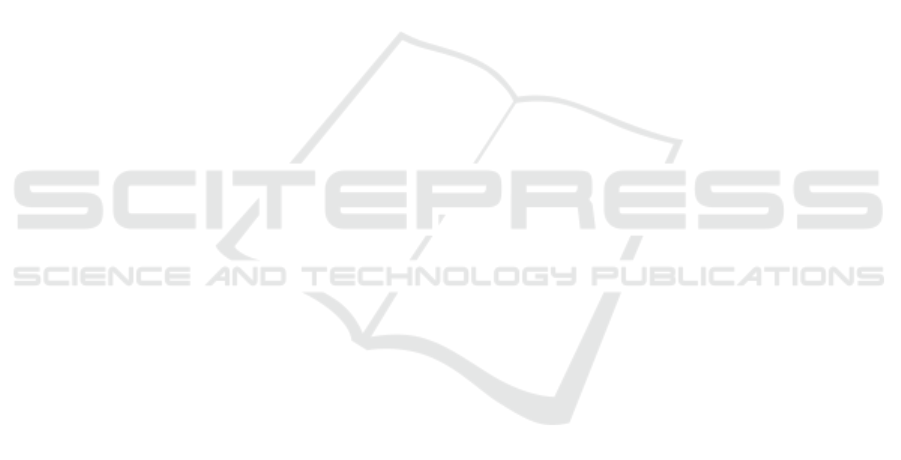
for discrimination prevention. In Advances in Neural
Information Processing Systems, pages 3992–4001.
Chen, Y., Zheng, Q., Ji, S., Tian, F., Zhu, H., and Liu, M.
(2019). Identifying at-risk students based on the phased
prediction model. Knowledge and Information Systems,
pages 1–17.
Chouldechova, A., Benavides-Prado, D., Fialko, O., and
Vaithianathan, R. (2018). A case study of algorithm-
assisted decision making in child maltreatment hotline
screening decisions. In Conference on Fairness, Ac-
countability and Transparency, pages 134–148.
Cohen, L., Manion, L., and Morrison, K. (2002). Research
methods in education. routledge.
Davila, A. and Bohara, A. K. (1994). Equal employment
opportunity across states: The eeoc 1979-1989. Public
Choice, 80(3/4):223–243.
Drachsler, H. and Greller, W. (2016). Privacy and analytics:
it’s a delicate issue a checklist for trusted learning
analytics. In Proceedings of the sixth international
conference on learning analytics & knowledge, pages
89–98. ACM.
Dwork, C., Hardt, M., Pitassi, T., Reingold, O., and Zemel,
R. (2012). Fairness through awareness. In Proceedings
of the 3rd innovations in theoretical computer science
conference, pages 214–226. ACM.
Ebner, M. and Ebner, M. (2018). Learning analytics an
schulen–hintergrund und beispiele. Medienimpulse,
56(1).
Ellis, E. and Watson, P. (2012). EU anti-discrimination law.
OUP Oxford.
Feldman, M., Friedler, S. A., Moeller, J., Scheidegger, C.,
and Venkatasubramanian, S. (2015). Certifying and
removing disparate impact. In Proceedings of the 21th
ACM SIGKDD International Conference on Knowl-
edge Discovery and Data Mining, pages 259–268.
ACM.
Ferguson, R., Hoel, T., Scheffel, M., and Drachsler, H.
(2016). Guest editorial: Ethics and privacy in learning
analytics. SoLAR.
Friedler, S. A., Scheidegger, C., Venkatasubramanian, S.,
Choudhary, S., Hamilton, E. P., and Roth, D. (2019). A
comparative study of fairness-enhancing interventions
in machine learning. In Proceedings of the Conference
on Fairness, Accountability, and Transparency, pages
329–338. ACM.
Gardner, J., Brooks, C., and Baker, R. (2019). Evaluating the
fairness of predictive student models through slicing
analysis. In Proceedings of the 9th International Con-
ference on Learning Analytics & Knowledge, pages
225–234. ACM.
Gilpin, L. H., Bau, D., Yuan, B. Z., Bajwa, A., Specter,
M., and Kagal, L. (2018). Explaining explanations:
An overview of interpretability of machine learning.
In 2018 IEEE 5th International Conference on Data
Science and Advanced Analytics (DSAA), pages 80–89.
IEEE.
Grandl, M., Taraghi, B., Ebner, M., Leitner, P., and Ebner, M.
(2017). Learning analytics. Handbuch E-Learning: Ex-
pertenwissen aus Wissenschaft und Praxis-Strategien,
pages 1–16.
Hardt, M., Price, E., Srebro, N., et al. (2016). Equality of
opportunity in supervised learning. In Advances in
neural information processing systems, pages 3315–
3323.
Kamiran, F. and Calders, T. (2012). Data preprocessing
techniques for classification without discrimination.
Knowledge and Information Systems, 33(1):1–33.
Kamishima, T., Akaho, S., Asoh, H., and Sakuma, J. (2012).
Fairness-aware classifier with prejudice remover regu-
larizer. In Joint European Conference on Machine
Learning and Knowledge Discovery in Databases,
pages 35–50. Springer.
Komiyama, J. and Shimao, H. (2017). Two-stage algorithm
for fairness-aware machine learning. arXiv preprint
arXiv:1710.04924.
Komiyama, J., Takeda, A., Honda, J., and Shimao, H. (2018).
Nonconvex optimization for regression with fairness
constraints. In International Conference on Machine
Learning, pages 2742–2751.
Kusner, M. J., Loftus, J., Russell, C., and Silva, R. (2017).
Counterfactual fairness. In Advances in Neural Infor-
mation Processing Systems, pages 4066–4076.
Kuzilek, J., Hlosta, M., and Zdrahal, Z. (2017). Open
university learning analytics dataset. Scientific data,
4:170171.
Larson, J., Mattu, S., Kirchner, L., and Angwin, J. (2016).
How we analyzed the compas recidivism algorithm.
ProPublica (5 2016), 9.
Learning Analytics and Knowledge (2019). Proceedings of
the 9th international conference on learning analytics
& knowledge. New York, NY, USA. ACM.
Menon, A. K. and Williamson, R. C. (2018). The cost of
fairness in binary classification. In Friedler, S. A. and
Wilson, C., editors, Proceedings of the 1st Conference
on Fairness, Accountability and Transparency, vol-
ume 81 of Proceedings of Machine Learning Research,
pages 107–118, New York, NY, USA. PMLR.
Michinov, N., Brunot, S., Le Bohec, O., Juhel, J., and
Delaval, M. (2011). Procrastination, participation, and
performance in online learning environments. Comput-
ers & Education, 56(1):243–252.
Migu
´
eis, V. L., Freitas, A., Garcia, P. J., and Silva, A. (2018).
Early segmentation of students according to their aca-
demic performance: A predictive modelling approach.
Decision Support Systems, 115:36–51.
Morris, L. V., Finnegan, C., and Wu, S.-S. (2005). Track-
ing student behavior, persistence, and achievement in
online courses. The Internet and Higher Education,
8(3):221–231.
Ochoa, X. and Merceron, A. (2018). Quantitative and qual-
itative analysis of the learning analytics and knowl-
edge conference 2018. Journal of Learning Analytics,
5(3):154–166.
Oladokun, V., Adebanjo, A., and Charles-Owaba, O. (2008).
Predicting students academic performance using arti-
ficial neural network: A case study of an engineering
course.
O’Neill, C. (2016). Weapons of math destruction: How
big data increases inequality and threatens democracy.
Nueva York, NY: Crown Publishing Group.
CSEDU 2020 - 12th International Conference on Computer Supported Education
24