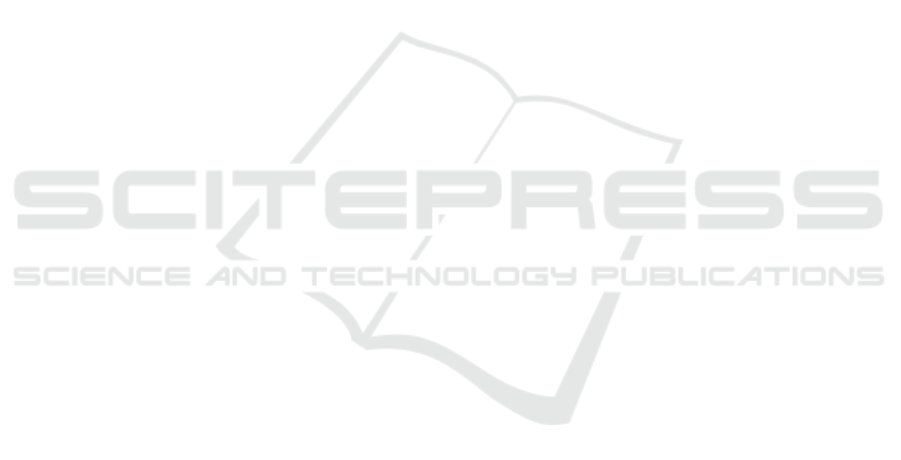
7 CONCLUSION
In conclusion, the persistence, as well as the repartic-
ipation of students in the “Mathe im Advent” calen-
dar strongly depended on prior mathematical knowl-
edge and command of German language. This ef-
fect was especially visible when the language of the
tasks was difficult and their readability was low. As
an outlook, we would like to further investigate the
connection between the perceived level of difficulty
of a task and their reading ease scores. This evalu-
ation, in a task-specific setting, would allow to au-
tomtically pre-assess tasks with regards to difficulty.
Future work will include the prediction of task dif-
ficulty using more sophisticated (machine learning)
methods.
ACKNOWLEDGEMENTS
This research has been funded by the Federal Ministry
of Education and Research of Germany in the frame-
work “Digitalisierung im Bildungsbereich” (project
number 01JD1812A).
REFERENCES
Abeer, W. and Miri, B. (2014). Students’ preferences and
views about learning in a MOOC. Procedia-Social
and Behavioral Sciences, 152:318–323.
Amstad, T. (1978). Wie verst
¨
andlich sind unsere Zeitungen?
Z
¨
urich: Studenten-Schreib-Service.
Bamberger, R. and Vanecek, E. (1984). Lesen, verstehen,
lernen, schreiben: die Schwierigkeitsstufen von Texten
in deutscher Sprache. Jugend und Volk.
Borba, M. C., Askar, P., Engelbrecht, J., Gadanidis, G.,
Llinares, S., and Aguilar, M. S. (2016). Blended learn-
ing, e-learning and mobile learning in mathematics
education. ZDM, 48(5):589–610.
Burrill, G. and Ben-Zvi, D. (2019). Topics and Trends in
Current Statistics Education Research: International
Perspectives. ICME-13 Monographs. Springer Inter-
national Publishing.
Crossley, S., McNamara, D. S., Baker, R., Wang, Y., Paque-
tte, L., Barnes, T., and Bergner, Y. (2015). Language
to completion: Success in an educational data mining
massive open online class. International Educational
Data Mining Society.
Crossley, S., Paquette, L., Dascalu, M., McNamara, D. S.,
and Baker, R. S. (2016). Combining click-stream data
with NLP tools to better understand MOOC comple-
tion. In Proceedings of the sixth international confer-
ence on learning analytics & knowledge, pages 6–14.
ACM.
Daza, V., Makriyannis, N., and Rovira Riera, C. (2013).
MOOC attack: closing the gap between pre-
university and university mathematics. Open Learn-
ing: The Journal of Open, Distance and e-Learning,
28(3):227–238.
Flesch, R. (1948). A new readability yardstick. Journal of
applied psychology, 32(3):221.
Gardner, J. and Brooks, C. (2018). Student success predic-
tion in MOOCs. User Modeling and User-Adapted
Interaction, 28(2):127–203.
Garman, G. (2010). A logistic approach to predicting stu-
dent success in online database courses. American
Journal of Business Education (AJBE), 3(12):1–6.
Hellas, A., Ihantola, P., Petersen, A., Ajanovski, V. V.,
Gutica, M., Hynninen, T., Knutas, A., Leinonen, J.,
Messom, C., and Liao, S. N. (2018). Predicting aca-
demic performance: a systematic literature review.
In Proceedings Companion of the 23rd Annual ACM
Conference on Innovation and Technology in Com-
puter Science Education, pages 175–199. ACM.
Jiang, S., Schenke, K., Eccles, J. S., Xu, D., and
Warschauer, M. (2016). Females’ enrollment and
completion in science, technology, engineering, and
mathematics massive open online courses. arXiv
preprint arXiv:1608.05131.
Kennedy, G., Coffrin, C., De Barba, P., and Corrin, L.
(2015). Predicting success: how learners’ prior
knowledge, skills and activities predict MOOC perfor-
mance. In Proceedings of the fifth international con-
ference on learning analytics and knowledge, pages
136–140. ACM.
Kincaid, J. P., Fishburne Jr, R. P., Rogers, R. L., and
Chissom, B. S. (1975). Derivation of new readability
formulas (automated readability index, fog count and
flesch reading ease formula) for navy enlisted person-
nel.
Lopes, A. P., Soares, F., and Vieira, I. (2015). Challenges
in the creation and development of a mathematics
MOOC. In Proceedings of the International Confer-
ence of Education, Research and Innovation (ICERI),
8th, pages 1862–1867. IATED.
Meaney, M. J. and Fikes, T. (2019). Early–adopter itera-
tion bias and research–praxis bias in the learning an-
alytics ecosystem. In Companion Proceedings of the
9th International Learning Analytics and Knowledge
Conference (LAK’19), pages 513 – 519.
Najafi, H., Evans, R., and Federico, C. (2014). MOOC in-
tegration into secondary school courses. The Inter-
national Review of Research in Open and Distributed
Learning, 15(5).
Ros
´
e, C. P., Carlson, R., Yang, D., Wen, M., Resnick, L.,
Goldman, P., and Sherer, J. (2014). Social factors that
contribute to attrition in MOOCs. In Proceedings of
the first ACM conference on Learning@ scale confer-
ence, pages 197–198. ACM.
Ruip
´
erez-Valiente, J. A., Halawa, S., and Reich, J. (2019).
Multiplatform MOOC analytics: Comparing global
and regional patterns in edX and Edraak.
Sclater, N., Peasgood, A., and Mullan, J. (2016). Learning
Prior Knowledge as a Predictor for Persistence
143