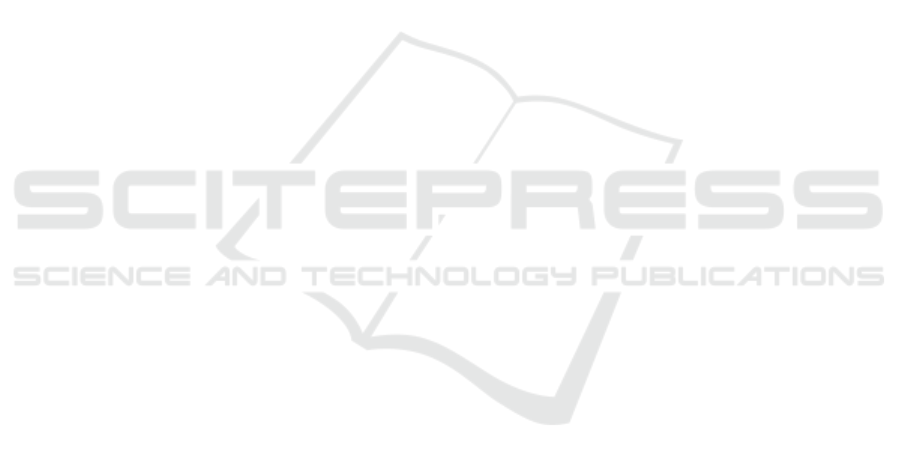
Li, Z., Hong, Y., and Zhang, Z. (2017). An Empirical Anal-
ysis of On-demand Ride-sharing and Traffic Conges-
tion. In Hawaii International Conference on System
Sciences.
Liu, W., Zhang, F., and Yang, H. (2017). Modeling and
managing morning commute with both household and
individual travels. Transportation Research Part B:
Methodological, 103:227–247.
Mahmoudi, M. and Zhou, X. (2016). Finding optimal so-
lutions for vehicle routing problem with pickup and
delivery services with time windows: A dynamic pro-
gramming approach based on state–space–time net-
work representations. Transportation Research Part
B: Methodological, 89:19–42.
Mann, H. B. and Whitney, D. R. (1947). On a Test of
Whether one of Two Random Variables is Stochasti-
cally Larger than the Other. The Annals of Mathemat-
ical Statistics, 18(1):50–60.
Mirsadikov, A., Harrison, A., and Mennecke, B. (2016).
Tales From the Wheel: An IT-Fueled Ride as an
UBER Driver. In San Diego, page 10.
Mourad, A., Puchinger, J., and Chu, C. (2019). A survey of
models and algorithms for optimizing shared mobil-
ity. Transportation Research Part B: Methodological,
123:323–346.
Neoh, J. G., Chipulu, M., and Marshall, A. (2017). What
encourages people to carpool? An evaluation of fac-
tors with meta-analysis. Transportation, 44(2):423–
447.
Neoh, J. G., Chipulu, M., Marshall, A., and Tewkesbury, A.
(2018). How commuters’ motivations to drive relate
to propensity to carpool: Evidence from the United
Kingdom and the United States. Transportation Re-
search Part A: Policy and Practice, 110:128–148.
Nielsen, J. R., Hovmøller, H., Blyth, P.-L., and Sovacool,
B. K. (2015). Of “white crows” and “cash savers:”
A qualitative study of travel behavior and perceptions
of ridesharing in Denmark. Transportation Research
Part A: Policy and Practice, 78:113–123.
Nourinejad, M. and Roorda, M. J. (2016). Agent based
model for dynamic ridesharing. Transportation Re-
search Part C: Emerging Technologies, 64:117–132.
Papenmeier, A., Englebienne, G., and Seifert, C. (2019).
How model accuracy and explanation fidelity influ-
ence user trust. In arXiv:1907.12652 [Cs].
Parmesan, C. and Yohe, G. (2003). A globally coherent
fingerprint of climate change impacts across natural
systems. Nature, 421(6918):37–42.
S
´
anchez, D., Mart
´
ınez, S., and Domingo-Ferrer, J. (2016).
Co-utile P2P ridesharing via decentralization and rep-
utation management. Transportation Research Part
C: Emerging Technologies, 73:147–166.
Schmitz, C. and Team, L. P. (2012). LimeSurvey: An Open
Source survey tool. LimeSurvey Project.
Schweitzer, S. and Brendel, J. (2018). The Segmented
Introduction of Transaction Fees in the German
Ridesharing Market. In PACIS 2018 Proceedings,
page 8, Japan.
Shared and Digital Mobility Committee (2018). Taxonomy
and Definitions for Terms Related to Shared Mobility
and Enabling Technologies. Technical report, SAE
International.
Statistisches Bundesamt (2019). 6 Prozent der EU-B
¨
urger
nutzen Mitfahrzentralen.
Stiglic, M., Agatz, N., Savelsbergh, M., and Gradisar, M.
(2015). The benefits of meeting points in ride-sharing
systems. Transportation Research Part B: Method-
ological, 82:36–53.
Sullivan, G. M. and Artino, A. R. (2013). Analyzing and
Interpreting Data From Likert-Type Scales. Journal
of Graduate Medical Education, 5(4):541–542.
Tian, L.-J., Sheu, J.-B., and Huang, H.-J. (2019). The morn-
ing commute problem with endogenous shared au-
tonomous vehicle penetration and parking space con-
straint. Transportation Research Part B: Methodolog-
ical, 123:258–278.
Tsao, H.-S. J. and Lin, D.-J. (1999). Spatial and Tempo-
ral Factors in Estimating the Potential of Ride-sharing
for Demand Reduction. California PATH Research
Report.
Vanoutrive, T., Van De Vijver, E., Van Malderen, L.,
Jourquin, B., Thomas, I., Verhetsel, A., and Witlox, F.
(2012). What determines carpooling to workplaces in
Belgium: Location, organisation, or promotion? Jour-
nal of Transport Geography, 22:77–86.
vom Brocke, J., Simons, A., Niehaves, B., Plattfaut, R.,
Cleven, A., and Reimer, K. (2009). Reconstructing
the giant: On the importance of rigour in documenting
the literature search process. European Conference on
Information Systems.
Wang, J.-P., Ban, X. J., and Huang, H.-J. (2019).
Dynamic ridesharing with variable-ratio charging-
compensation scheme for morning commute. Trans-
portation Research Part B: Methodological, 122:390–
415.
Wang, X., Agatz, N., and Erera, A. (2018). Stable Matching
for Dynamic Ride-Sharing Systems. Transportation
Science, 52(4):850–867.
Washbrook, K., Haider, W., and Jaccard, M. (2006). Es-
timating commuter mode choice: A discrete choice
analysis of the impact of road pricing and parking
charges. Transportation, 33(6):621–639.
Webster, J. and Watson, R. T. (2002). Analyzing the past
to prepare for the future: Writing a literature review.
MIS Quarterly, 26(2):13–23.
Xiao, L.-L., Liu, T.-L., and Huang, H.-J. (2016). On the
morning commute problem with carpooling behavior
under parking space constraint. Transportation Re-
search Part B: Methodological, 91:383–407.
Yan, X., Levine, J., and Zhao, X. (2019). Integrating rides-
ourcing services with public transit: An evaluation of
traveler responses combining revealed and stated pref-
erence data. Transportation Research Part C: Emerg-
ing Technologies, 105:683–696.
Yousaf, J., Li, J., Chen, L., Tang, J., and Dai, X.
(2014). Generalized multipath planning model for
ride-sharing systems. Frontiers of Computer Science,
8(1):100–118.
Zhang, N., Kien, S. S., and Lee, G.-w. (2018). The Insti-
tutional Legitimacy of Disruptive Start-ups in Sharing
Economy. In PACIS 2018 Proceedings, page 8, Japan.
VEHITS 2020 - 6th International Conference on Vehicle Technology and Intelligent Transport Systems
168