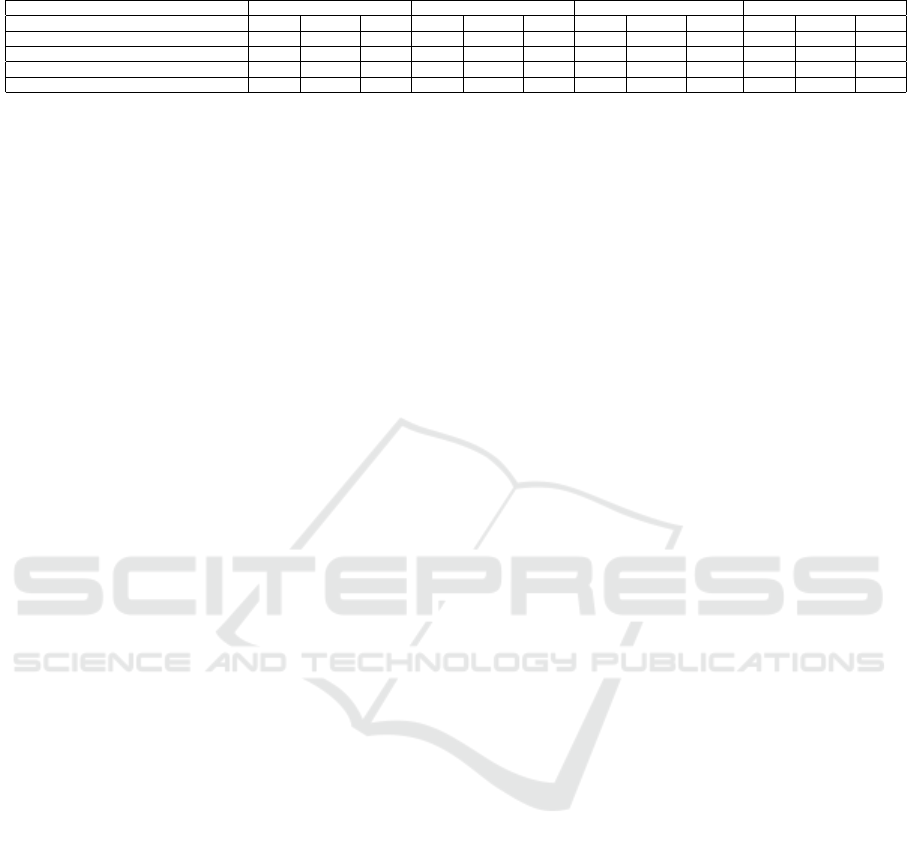
Table 2: MAPE, RMSPE and RMSE for the ILI Count Predicted Using ARIMA and Proposed Model of Region 5.
Weeks 1-week 5-week 10-week 15-week
Model MAPE RMSPE RMSE MAPE RMSPE RMSE MAPE RMSPE RMSE MAPE RMSPE RMSE
LSTM (Hochreiter and Schmidhuber, 1997) 31.95 31.95 1364 21.15 22.59 1134.9 23.92 25.02 1113.35 28.42 29.92 998.89
Proposed model 20.09 20.09 846.8 11.59 13.42 650.18 8.18 10.42 520.37 17.04 23.41 599.73
ARIMA (Choi and Thacker, 1981) 22.23 22.23 937 15.87 17.05 957.68 18.13 19.58 912.08 19.68 21.62 791.33
ARIMA+External factors 22.23 22.23 937 16.03 17.30 977.41 13.88 15.97 850.58 22.07 30.01 845.77
real-time data better than the other model. Accord-
ing to these three errors in Fig. 4 and Fig. 5, we can
say that the proposed approach is favourable for the
prediction of influenza-Like illness.
5 CONCLUSIONS
In this study, we proposed an approach to enhance in-
fluenza prediction. In our contribution, first step is the
application of LSTM as machine learning technique,
this method shows a better performance comparing
to the existing time series prediction methods. Sec-
ond step is the integration of the impacts of the exter-
nal predictors : air pollution data, climatic variables
and geographical proximity whose goal is to reduce
the error of machine learning method. We evaluated
the approach we proposed on the datasets from CDC-
HHS ILI. The proposed approach is compared with
ARIMA model. It can be seen that with the integra-
tion of external predictors in LSTM, we improved the
accuracy performance. Also, the proposed approach
may be useful for other viral illness such as Asthma,
Chickenpox and Ebola. Our future study seeks to
implement the proposed approach on Social Network
Site like Twitter and Instagram dataset.
REFERENCES
Alessa, A. and Faezipour, M. (2018). A review of in-
fluenza detection and prediction through social net-
working sites. Theoretical Biology and Medical Mod-
elling, 15(1):2.
Bradley, C. A., Rolka, H., Walker, D., and Loonsk, J.
(2005). Biosense: implementation of a national
early event detection and situational awareness sys-
tem. MMWR Morb Mortal Wkly Rep, 54(Suppl):11–
19.
Choi, K. and Thacker, S. B. (1981). An evaluation
of influenza mortality surveillance, 1962–1979: I.
time series forecasts of expected pneumonia and in-
fluenza deaths. American journal of epidemiology,
113(3):215–226.
Chowell, G., Miller, M., and Viboud, C. (2008). Seasonal
influenza in the united states, france, and australia:
transmission and prospects for control. Epidemiology
& Infection, 136(6):852–864.
Chowell, G., Nishiura, H., and Bettencourt, L. M. (2006).
Comparative estimation of the reproduction num-
ber for pandemic influenza from daily case notifica-
tion data. Journal of the Royal Society Interface,
4(12):155–166.
Donnelly, C. F., Riley, S., Ferguson, N. M., and Anderson,
R. M. (2005). Transmission dynamics and control of
the viral aetiological agent of sars. Severe Acute Res-
piratory Syndrome: A Clinical Guide, page 111.
Dugas, A. F., Jalalpour, M., Gel, Y., Levin, S., Torcaso, F.,
Igusa, T., and Rothman, R. E. (2013). Influenza fore-
casting with google flu trends. PloS one, 8(2):e56176.
Elkin, P. L., Froehling, D., Wahner-Roedler, D., Brown,
S. H., and Bailey, K. R. (2012). Comparison of nat-
ural language processing biosurveillance methods for
identifying influenza from encounter notes. Annals of
internal medicine, 156 1 Pt 1:11–8.
Gers, F. A., Schmidhuber, J., and Cummins, F. (1999).
Learning to forget: Continual prediction with lstm.
Ginsberg, J., Mohebbi, M. H., Patel, R. S., Brammer, L.,
Smolinski, M. S., and Brilliant, L. (2009). Detecting
influenza epidemics using search engine query data.
Nature, 457(7232):1012.
Hethcote, H. W. (2000). The mathematics of infectious dis-
eases. SIAM review, 42(4):599–653.
Hochreiter, S. and Schmidhuber, J. (1997). Long short-term
memory. Neural computation, 9(8):1735–1780.
Hooten, M. B., Anderson, J., and Waller, L. A. (2010). As-
sessing north american influenza dynamics with a sta-
tistical sirs model. Spatial and spatio-temporal epi-
demiology, 1(2-3):177–185.
Hu, H., Wang, H., Wang, F., Langley, D., Avram, A., and
Liu, M. (2018). Prediction of influenza-like illness
based on the improved artificial tree algorithm and ar-
tificial neural network. Scientific reports, 8(1):4895.
Keeling, M. J. and Rohani, P. (2011). Modeling infectious
diseases in humans and animals. Princeton University
Press.
Li, Q., Song, K., He, Z., Li, E., Cheng, A., and Chen, T.
(2017). The artificial tree (at) algorithm. Engineering
Applications of Artificial Intelligence, 65:99–110.
Liu, L., Han, M., Zhou, Y., and Wang, Y. (2018). Lstm
recurrent neural networks for influenza trends predic-
tion. In International Symposium on Bioinformatics
Research and Applications, pages 259–264. Springer.
Lowen, A. C., Mubareka, S., Steel, J., and Palese, P.
(2007). Influenza virus transmission is dependent on
relative humidity and temperature. PLoS pathogens,
3(10):e151.
Lowen, A. C. and Steel, J. (2014). Roles of humidity and
temperature in shaping influenza seasonality. Journal
of virology, 88(14):7692–7695.
Data-driven Model for Influenza Prediction Incorporating Environmental Effects
23