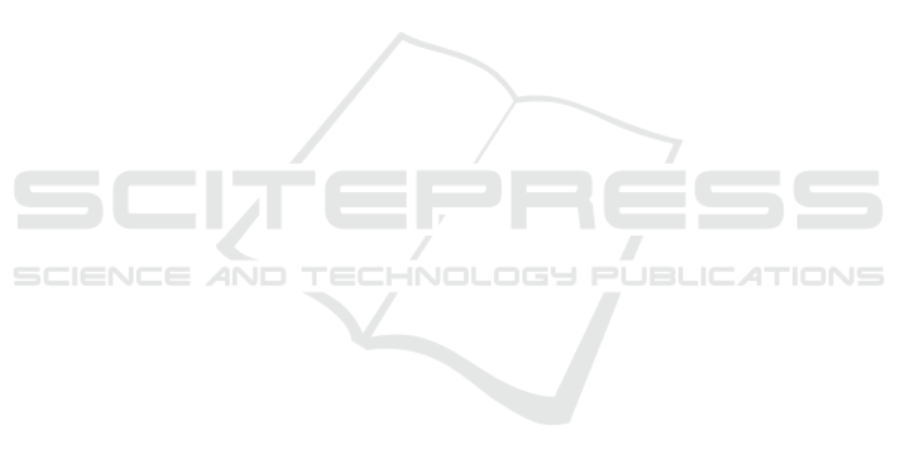
tion. The number of ML algorithms and techniques
has been discussed in terms of types of input, pur-
poses, advantages, and disadvantages. For stock price
prediction, some of ML algorithms and techniques
have been popularly selected as to their characteris-
tics, accuracy and error acquired.
In addition to the historical prices, other informa-
tion might have effect to the stock such as politics,
economic growth, financial news and social media.
Many studies have proven that the sentiment analysis
has a high impact on future prices. Thus, a mix of
technical and fundamental analyses could produce the
prediction more efficient and would be interesting to
be added in to the state-of-the-art ML as future works.
REFERENCES
Adebiyi, A. A., Adewumi, A. O., and Ayo, C. K. (2014).
Comparison of ARIMA and artificial neural networks
models for stock price prediction. Journal of Applied
Mathematics, 2014.
Aghabozorgi, S., Shirkhorshidi, A. S., and Wah, T. Y.
(2015). Time-series clustering–a decade review. In-
formation Systems, 53:16–38.
Al-Mahasneh, A. J., Anavatti, S. G., and Garratt, M. A.
(2018). Review of Applications of Generalized
Regression Neural Networks in Identification and
Control of Dynamic Systems. arXiv preprint
arXiv:1805.11236.
Alfred, R. et al. (2015). A genetic-based backpropagation
neural network for forecasting in time-series data. In
2015 International Conference on Science in Informa-
tion Technology (ICSITech), pages 158–163. IEEE.
Alpaydin, E. (2014). Introduction to machine learning.
MIT press.
Althelaya, K. A., El-Alfy, E.-S. M., and Mohammed, S.
(2018a). Evaluation of bidirectional LSTM for short-
and long-term stock market prediction. In 2018 9th
International Conference on Information and Com-
munication Systems (ICICS), pages 151–156. IEEE.
Althelaya, K. A., El-Alfy, E.-S. M., and Mohammed, S.
(2018b). Stock Market Forecast Using Multivari-
ate Analysis with Bidirectional and Stacked (LSTM,
GRU). In 2018 21st Saudi Computer Society National
Computer Conference (NCC), pages 1–7. IEEE.
Archana, S. and Elangovan, K. (2014). Survey of classifi-
cation techniques in data mining. International Jour-
nal of Computer Science and Mobile Applications,
2(2):65–71.
Attigeri, G. V., MM, M. P., Pai, R. M., and Nayak, A.
(2015). Stock market prediction: A big data approach.
In TENCON 2015-2015 IEEE Region 10 Conference,
pages 1–5. IEEE.
Bai, S., Kolter, J. Z., and Koltun, V. (2018). An empiri-
cal evaluation of generic convolutional and recurrent
networks for sequence modeling. arXiv preprint
arXiv:1803.01271.
Belgacem, S., Chatelain, C., and Paquet, T. (2017).
Gesture sequence recognition with one shot learned
CRF/HMM hybrid model. Image and Vision Comput-
ing, 61:12–21.
Beyaz, E., Tekiner, F., Zeng, X.-j., and Keane, J. (2018).
Comparing technical and fundamental indicators in
stock price forecasting. In 2018 IEEE 20th In-
ternational Conference on High Performance Com-
puting and Communications; IEEE 16th Interna-
tional Conference on Smart City; IEEE 4th Inter-
national Conference on Data Science and Systems
(HPCC/SmartCity/DSS), pages 1607–1613. IEEE.
Bodie, Z., Kane, A., and Marcus, A. J. (2013). Investments
and portfolio management. McGraw Hill Education
(India) Private Limited.
Boomija, M. and Phil, M. (2008). Comparison of partition
based clustering algorithms. Journal of Computer
Applications, 1(4):18–21.
Brown, B. (2017). The forward market in foreign exchange:
a study in market-making, arbitrage and speculation.
Routledge.
Chou, J.-S. and Nguyen, T.-K. (2018). Forward Forecast
of Stock Price Using Sliding-Window Metaheuristic-
Optimized Machine-Learning Regression. IEEE
Transactions on Industrial Informatics, 14(7):3132–
3142.
Dang, M. and Duong, D. (2016). Improvement methods for
stock market prediction using financial news articles.
In 2016 3rd National Foundation for Science and
Technology Development Conference on Information
and Computer Science (NICS), pages 125–129. IEEE.
G
¨
oc¸ken, M.,
¨
Ozc¸alıcı, M., Boru, A., and Dosdo
˘
gru, A. T.
(2016). Integrating metaheuristics and artificial neural
networks for improved stock price prediction. Expert
Systems with Applications, 44:320–331.
Grover, N. (2014). A study of various Fuzzy Clustering
Algorithms. In 3, editor, International Journal of
Engineering Research, volume 3, pages 177–181.
He, J., Cai, L., Cheng, P., and Fan, J. (2015). Opti-
mal investment for retail company in electricity mar-
ket. IEEE Transactions on Industrial Informatics,
11(5):1210–1219.
Hegazy, O., Soliman, O. S., and Salam, M. A. (2014). A
machine learning model for stock market prediction.
arXiv preprint arXiv:1402.7351.
Hyndman, R. J. and Athanasopoulos, G. (2018). Forecast-
ing: principles and practice. OTexts.
Ijegwa, A. D., Rebecca, V. O., Olusegun, F., and Isaac,
O. O. (2014). A predictive stock market technical
analysis using fuzzy logic. Computer and information
science, 7(3):1.
Jeong, Y., Kim, S., and Yoon, B. (2018). An Algorithm
for Supporting Decision Making in Stock Investment
through Opinion Mining and Machine Learning. In
2018 Portland International Conference on Man-
agement of Engineering and Technology (PICMET),
pages 1–10. IEEE.
Khare, K., Darekar, O., Gupta, P., and Attar, V. (2017).
Short term stock price prediction using deep learning.
In 2017 2nd IEEE International Conference on Recent
Trends in Electronics, Information & Communication
Technology (RTEICT), pages 482–486. IEEE.
FEMIB 2020 - 2nd International Conference on Finance, Economics, Management and IT Business
70