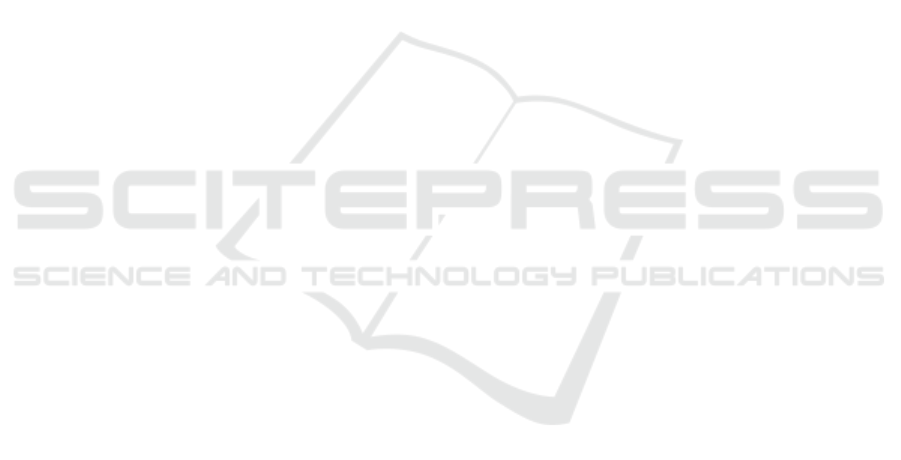
Future work might address the extension of the
method to other traffic situations, e. g., intersections
or inbound roundabout arms. The results of this work
can be helpful for behavior planning of automated ve-
hicles or advanced driver assistance systems.
ACKNOWLEDGMENTS
A big thank you goes to Richard Schneidt for captur-
ing the trajectory dataset and to Moritz Oertel for his
support with the implementation of the optimization-
based trajectory generation.
The German Federal Ministry of Economics and
Energy funded this research within the project @City:
Automated Cars and Intelligent Traffic in the City.
This work was supported by AUDI AG.
REFERENCES
Bock, J., Krajewski, R., Moers, T., Runde, S., Vater, L.,
and Eckstein, L. (2019). The inD Dataset: A Drone
Dataset of Naturalistic Road User Trajectories at Ger-
man Intersections. arXiv preprint arXiv:1911.07602.
Deo, N., Rangesh, A., and Trivedi, M. M. (2018). How
Would Surround Vehicles Move? A Unified Frame-
work for Maneuver Classification and Motion Pre-
diction. IEEE Transactions on Intelligent Vehicles,
3(2):129–140.
Gao, F. and Han, L. (2012). Implementing the Nelder-Mead
simplex algorithm with adaptive parameters. Com-
putational Optimization and Applications, 51(1):259–
277.
Gindele, T., Brechtel, S., and Dillmann, R. (2015). Learn-
ing Driver Behavior Models from Traffic Observa-
tions for Decision Making and Planning. IEEE Intel-
ligent Transportation Systems Magazine, 7(1):69–79.
K
¨
afer, E., Hermes, C., W
¨
ohler, C., Ritter, H., and Kum-
mert, F. (2010). Recognition of situation classes at
road intersections. In IEEE International Conference
on Robotics and Automation, pages 3960–3965, An-
chorage, AK.
Lef
`
evre, S., Laugier, C., and Ibanez-Guzman, J. (2012).
Risk assessment at road intersections: Comparing in-
tention and expectation. In IEEE Intelligent Vehi-
cles Symposium, pages 165–171, Alcal de Henares ,
Madrid, Spain. IEEE.
Lef
`
evre, S., Vasquez, D., and Laugier, C. (2014). A survey
on motion prediction and risk assessment for intelli-
gent vehicles. ROBOMECH Journal, 1(1).
Liebner, M., Baumann, M., Klanner, F., and Stiller, C.
(2012). Driver intent inference at urban intersections
using the intelligent driver model. In IEEE Intelli-
gent Vehicles Symposium, pages 1162–1167, Alcal de
Henares , Madrid, Spain.
Meyer-Delius, D., Plagemann, C., von Wichert, G., Feiten,
W., Lawitzky, G., and Burgard, W. (2008). A Proba-
bilistic Relational Model for Characterizing Situations
in Dynamic Multi-Agent Systems. In Preisach, C.,
Burkhardt, H., Schmidt-Thieme, L., and Decker, R.,
editors, Data Analysis, Machine Learning and Appli-
cations, pages 269–276. Springer Berlin Heidelberg.
Morris, B. T. and Trivedi, M. M. (2011). Trajectory Learn-
ing for Activity Understanding: Unsupervised, Mul-
tilevel, and Long-Term Adaptive Approach. IEEE
Transactions on Pattern Analysis and Machine Intel-
ligence, 33(11):2287–2301.
Murphy, K. P. (2012). Machine Learning – A Probabilistic
Perspective. MIT Press.
Phillips, D. J., Wheeler, T. A., and Kochenderfer, M. J.
(2017). Generalizable intention prediction of human
drivers at intersections. In IEEE Intelligent Vehi-
cles Symposium, pages 1665–1670, Los Angeles, CA,
USA.
Quehl, J., Hu, H., Tas, O. S., Rehder, E., and Lauer, M.
(2017). How good is my prediction? Finding a simi-
larity measure for trajectory prediction evaluation. In
IEEE International Conference on Intelligent Trans-
portation Systems, pages 1–6, Yokohama.
Salvador, S. and Chan, P. (2007). FastDTW: Toward Ac-
curate Dynamic Time Warping in Linear Time and
Space. Intelligent Data Analysis, pages 561–580.
Schreier, M. (2015). Bayesian environment representation,
prediction, and criticality assessment for driver assis-
tance systems. PhD thesis, TU Darmstadt.
Schubert, R., Richter, E., and Wanielik, G. (2008). Com-
parison and Evaluation of Advanced Motion Models
for Vehicle Tracking. In International Conference on
Information Fusion, pages 1–6, Cologne.
Schulz, J., Hubmann, C., L
¨
ochner, J., and Burschka, D.
(2018). Multiple Model Unscented Kalman Filter-
ing in Dynamic Bayesian Networks for Intention Es-
timation and Trajectory Prediction. In IEEE Interna-
tional Conference on Intelligent Transportation Sys-
tems, pages 1467–1474, Maui, HI.
Schulz, J., Hubmann, C., Morin, N., L
¨
ochner, J., and
Burschka, D. (2019). Learning Interaction-Aware
Probabilistic Driver Behavior Models from Urban
Scenarios. In IEEE Intelligent Vehicles Symposium,
pages 1326–1333, Paris, France.
Streubel, T. and Hoffmann, K. H. (2014). Prediction of
driver intended path at intersections. In IEEE Intel-
ligent Vehicles Symposium, pages 134–139, MI, USA.
IEEE.
Tran, Q. and Firl, J. (2013). Modelling of traffic situations at
urban intersections with probabilistic non-parametric
regression. In IEEE Intelligent Vehicles Symposium,
pages 334–339, Gold Coast City, Australia.
Vasquez, D., Fraichard, T., and Laugier, C. (2009). In-
cremental learning of statistical motion patterns with
growing hidden Markov models. In IEEE Trans-
actions on Intelligent Transportation Systems 10(3),
pages 403–416.
Vintsyuk, T. K. (1972). Speech discrimination by dynamic
programming. Cybernetics, 4(1):52–57.
VEHITS 2020 - 6th International Conference on Vehicle Technology and Intelligent Transport Systems
310