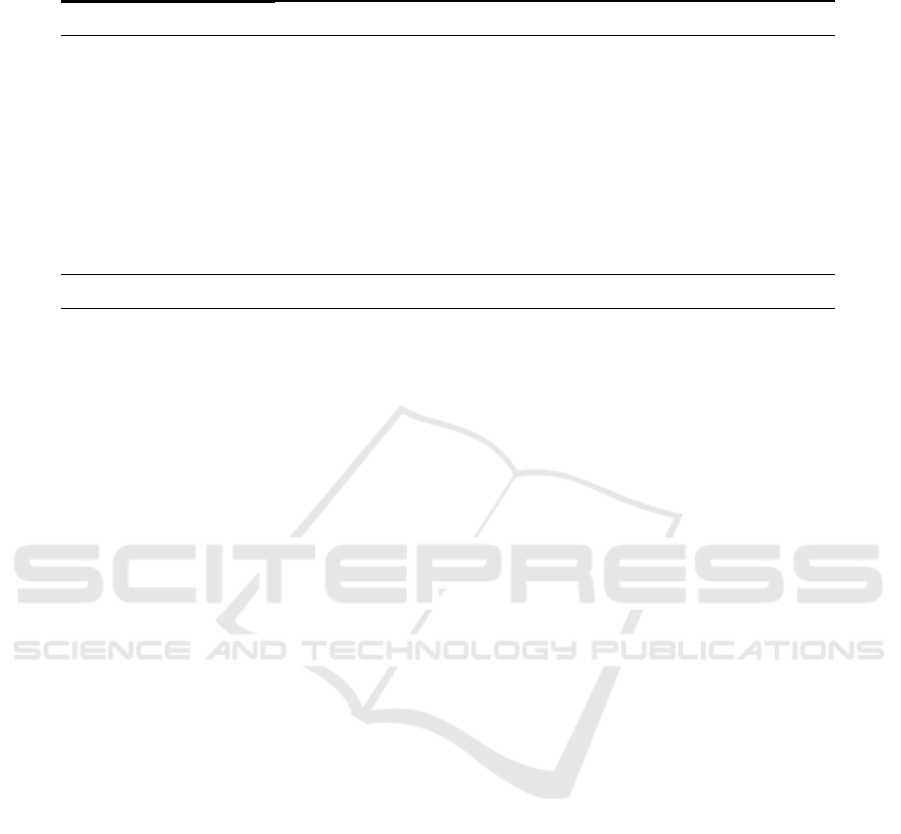
Table 3: Average rotation parameter values across classes of ModelNet10. The values are reformatted to be positive angles
between 0 and 360.
Class r
1
r
2
r
3
r
4
r
5
r
6
r
7
r
8
r
9
Bathtub Bathtub 319.2 100.5 57.8 185.2 223.4 98.3 350.6 167.4 14.2
Bed 264.3 196.3 103.7 208.5 186.2 194.4 267.9 246.3 81.2
Chair 198.6 91.2 243.7 47.4 161.2 87.9 240.5 47.3 203.4
Desk 88.4 80.2 130.9 206.6 86.5 112.8 291.7 233.2 351.4
Dresser 58.0 145.7 353.1 148.4 346.4 125.3 47.0 2.2 35.4
Monitor 218.9 279.0 58.1 10.4 30.3 331.4 90.7 285.6 346.1
Night stand 85.3 336.1 175.9 246.4 169.4 278.7 317.0 137.6 302.9
Sofa 306.1 86.9 109.2 311.1 22.5 321.4 96.9 47.0 76.2
Table 299.8 85.2 126.5 215.1 221.9 245.5 237.1 50.6 128.4
Toilet 277.0 325.3 215.5 255.6 192.2 19.8 278.4 193.4 348.2
Average 211.6 172.6 157.4 183.4 164.1 182.4 221.8 141.1 189.7
6 CONCLUSION
In this work, we present a novel experimental archi-
tecture, which can learn feature representations in
B
3
.
We utilize the underlying theoretical foundations for
volumetric convolution and demonstrate how it can
be efficiently computed and implemented using low-
cost matrix multiplications. Moreover, we show that
our experimental architecture gives competitive results
to state-of-the-art with a relatively shallow design, in
3D object recognition task. Finally, we empirically
demonstrate that fusing learned and hand-crafted fea-
tures results in improved performance, as they provide
complementary information.
REFERENCES
Bai, S., Bai, X., Zhou, Z., Zhang, Z., and Latecki, L. J.
(2016). Gift: A real-time and scalable 3d shape search
engine. In Computer Vision and Pattern Recognition
(CVPR), 2016 IEEE Conference on, pages 5023–5032.
IEEE.
Boomsma, W. and Frellsen, J. (2017). Spherical convolu-
tions and their application in molecular modelling. In
Advances in Neural Information Processing Systems,
pages 3436–3446.
Brock, A., Lim, T., Ritchie, J. M., and Weston, N.
(2016). Generative and discriminative voxel modeling
with convolutional neural networks. arXiv preprint
arXiv:1608.04236.
Canterakis, N. (1999). 3d zernike moments and zernike
affine invariants for 3d image analysis and recogni-
tion. In In 11th Scandinavian Conf. on Image Analysis.
Citeseer.
Cohen, T. S., Geiger, M., Koehler, J., and Welling, M. (2018).
Spherical cnns. International Conference on Learning
representations (ICLR).
Gao, Y., Beijbom, O., Zhang, N., and Darrell, T. (2016).
Compact bilinear pooling. In Proceedings of the IEEE
Conference on Computer Vision and Pattern Recogni-
tion, pages 317–326.
Garcia-Garcia, A., Gomez-Donoso, F., Garcia-Rodriguez, J.,
Orts-Escolano, S., Cazorla, M., and Azorin-Lopez, J.
(2016). Pointnet: A 3d convolutional neural network
for real-time object class recognition. In Neural Net-
works (IJCNN), 2016 International Joint Conference
on, pages 1578–1584. IEEE.
He, K., Zhang, X., Ren, S., and Sun, J. (2016). Deep resid-
ual learning for image recognition. In Proceedings of
the IEEE conference on computer vision and pattern
recognition, pages 770–778.
Johns, E., Leutenegger, S., and Davison, A. J. (2016). Pair-
wise decomposition of image sequences for active
multi-view recognition. In Computer Vision and Pat-
tern Recognition (CVPR), 2016 IEEE Conference on,
pages 3813–3822. IEEE.
Kanezaki, A., Matsushita, Y., and Nishida, Y. (2016). Rota-
tionnet: Joint object categorization and pose estimation
using multiviews from unsupervised viewpoints. arXiv
preprint arXiv:1603.06208.
Klokov, R. and Lempitsky, V. (2017). Escape from cells:
Deep kd-networks for the recognition of 3d point cloud
models. In 2017 IEEE International Conference on
Computer Vision (ICCV), pages 863–872. IEEE.
Krizhevsky, A., Sutskever, I., and Hinton, G. E. (2012). Im-
agenet classification with deep convolutional neural
networks. In Advances in neural information process-
ing systems, pages 1097–1105.
Li, J., Chen, B. M., and Lee, G. H. (2018). So-net: Self-
organizing network for point cloud analysis. arXiv
preprint arXiv:1803.04249.
Maturana, D. and Scherer, S. (2015). Voxnet: A 3d convo-
lutional neural network for real-time object recogni-
tion. In Intelligent Robots and Systems (IROS), 2015
IEEE/RSJ International Conference on, pages 922–928.
IEEE.
Qi, C. R., Su, H., Mo, K., and Guibas, L. J. (2017). Point-
ICPRAM 2020 - 9th International Conference on Pattern Recognition Applications and Methods
122