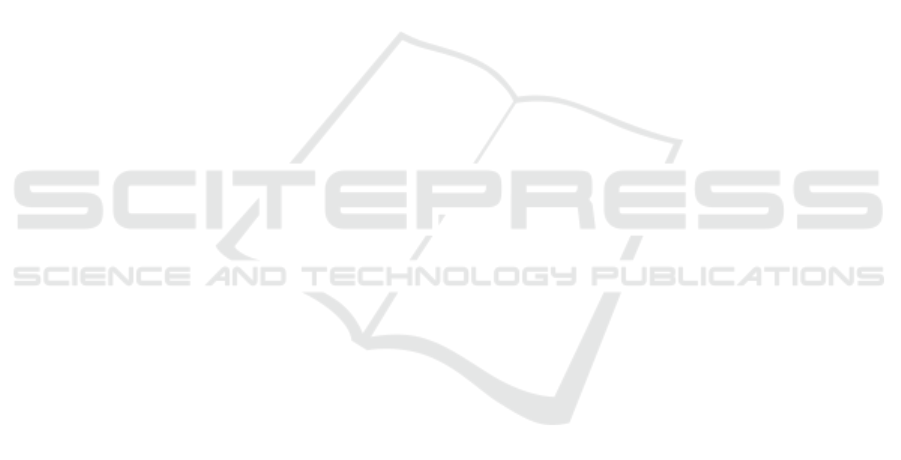
6 CONCLUSION
This paper presents a traffic light detection and recog-
nition system based on convolutional neural networks
for Taiwan road scenes. A two-stage approach is pro-
posed with first detecting the traffic light position, fol-
lowed by the light state recognition. It is specifically
designed to handle the arrow signal lights. In the traf-
fic light detection stage, the map information is used
to facilitate the detection by restricting the ROI. Two
cameras with different focal lengths are used to cap-
ture the near and far scenes. In the recognition stage,
a method combining the object detection and clas-
sification is presented. It is used to cope with the
problem of multiple light state classes in many urban
traffic scenes. The proposed end-to-end unified net-
work with shared feature maps has greatly reduced
the training and inference computation. The experi-
ments carried out using LISA dataset and our dataset
have demonstrated the effectiveness of the proposed
technique.
ACKNOWLEDGMENTS
The support of this work in part by the Ministry
of Science and Technology of Taiwan under Grant
MOST 106-2221-E-194-004 and the Advanced In-
stitute of Manufacturing with High-tech Innovations
(AIM-HI) from The Featured Areas Research Cen-
ter Program within the framework of the Higher Ed-
ucation Sprout Project by the Ministry of Education
(MOE) in Taiwan is gratefully acknowledged.
REFERENCES
Abboud, K., Omar, H. A., and Zhuang, W. (2016). Inter-
working of dsrc and cellular network technologies for
v2x communications: A survey. IEEE Transactions
on Vehicular Technology, 65:9457–9470.
Bach, M., Stumper, D., and Dietmayer, K. C. J. (2018).
Deep convolutional traffic light recognition for auto-
mated driving. 2018 21st International Conference on
Intelligent Transportation Systems (ITSC), pages 851–
858.
Behrendt, K., Novak, L., and Botros, R. (2017). A deep
learning approach to traffic lights: Detection, track-
ing, and classification. 2017 IEEE International Con-
ference on Robotics and Automation (ICRA), pages
1370–1377.
Caesar, H., Bankiti, V., Lang, A. H., Vora, S., Li-
ong, V. E., Xu, Q., Krishnan, A., Pan, Y., Baldan,
G., and Beijbom, O. (2019). nuscenes: A multi-
modal dataset for autonomous driving. arXiv preprint
arXiv:1903.11027.
Fairfield, N. and Urmson, C. (2011). Traffic light mapping
and detection. 2011 IEEE International Conference
on Robotics and Automation, pages 5421–5426.
Fregin, A., M
¨
uller, J. M., and Dietmayer, K. C. J. (2017a).
Feature detectors for traffic light recognition. 2017
IEEE 20th International Conference on Intelligent
Transportation Systems (ITSC), pages 339–346.
Fregin, A., M
¨
uller, J. M., and Dietmayer, K. C. J. (2017b).
Three ways of using stereo vision for traffic light
recognition. 2017 IEEE Intelligent Vehicles Sympo-
sium (IV), pages 430–436.
Hirabayashi, M., Sujiwo, A., Monrroy, A., Kato, S., and
Edahiro, M. (2019). Traffic light recognition us-
ing high-definition map features. Robotics and Au-
tonomous Systems, 111:62–72.
Jensen, M. B., Philipsen, M. P., Møgelmose, A., Moeslund,
T. B., and Trivedi, M. M. (2016). Vision for look-
ing at traffic lights: Issues, survey, and perspectives.
IEEE Transactions on Intelligent Transportation Sys-
tems, 17:1800–1815.
Kim, H.-K., Park, J. H., and Jung, H.-Y. (2011). Effective
traffic lights recognition method for real time driving
assistance systemin the daytime.
Krizhevsky, A., Sutskever, I., and Hinton, G. E. (2012). Im-
agenet classification with deep convolutional neural
networks. Commun. ACM, 60:84–90.
LeCun, Y., Bottou, L., Bengio, Y., Haffner, P., et al. (1998).
Gradient-based learning applied to document recogni-
tion. Proceedings of the IEEE, 86(11):2278–2324.
Li, X., Ma, H., Wang, X., and Zhang, X. (2018). Traffic
light recognition for complex scene with fusion detec-
tions. IEEE Transactions on Intelligent Transporta-
tion Systems, 19:199–208.
Liu, W., Anguelov, D., Erhan, D., Szegedy, C., Reed, S. E.,
Fu, C.-Y., and Berg, A. C. (2016). Ssd: Single shot
multibox detector. In ECCV.
M
¨
uller, J. M. and Dietmayer, K. C. J. (2018). Detecting
traffic lights by single shot detection. 2018 21st In-
ternational Conference on Intelligent Transportation
Systems (ITSC), pages 266–273.
M
¨
uller, J. M., Fregin, A., and Dietmayer, K. C. J. (2017).
Multi-camera system for traffic light detection: About
camera setup and mapping of detections. 2017 IEEE
20th International Conference on Intelligent Trans-
portation Systems (ITSC), pages 165–172.
Ramanishka, V., Chen, Y.-T., Misu, T., and Saenko, K.
(2018). Toward driving scene understanding: A
dataset for learning driver behavior and causal reason-
ing. In Conference on Computer Vision and Pattern
Recognition.
Redmon, J., Divvala, S. K., Girshick, R. B., and Farhadi, A.
(2016). You only look once: Unified, real-time object
detection. 2016 IEEE Conference on Computer Vision
and Pattern Recognition (CVPR), pages 779–788.
Redmon, J. and Farhadi, A. (2018). Yolov3: An incremental
improvement. CoRR, abs/1804.02767.
Ren, S., He, K., Girshick, R. B., and Sun, J. (2015). Faster
r-cnn: Towards real-time object detection with region
proposal networks. IEEE Transactions on Pattern
Analysis and Machine Intelligence, 39:1137–1149.
Detection and Recognition of Arrow Traffic Signals using a Two-stage Neural Network Structure
329