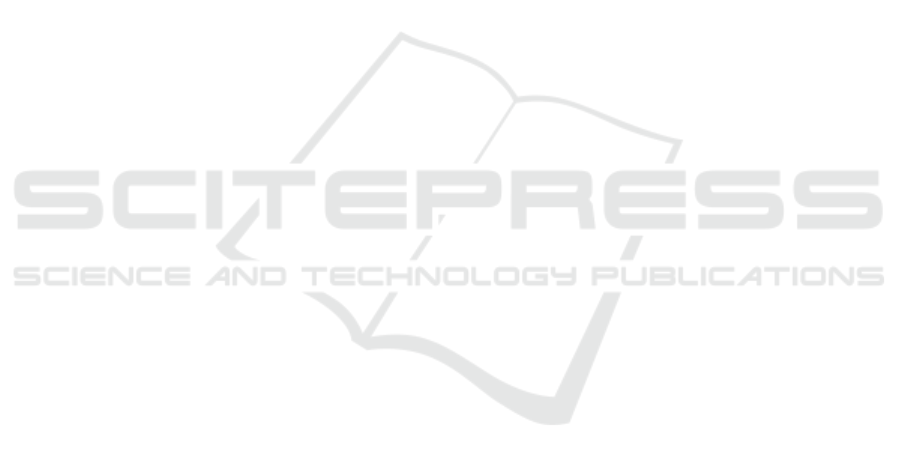
REFERENCES
Archambault, I., Janosz, M., Fallu, J.-S., and Pagani, L. S.
(2009). Student engagement and its relationship with
early high school dropout. Journal of adolescence,
32(3):651–670.
B
´
eres, F., Kelen, D. M., and Bencz
´
ur, A. A. (2019). Se-
quential skip prediction using deep learning and en-
sembles.
Chen, Q. and Yan, Z. (2016). Does multitasking with mo-
bile phones affect learning? a review. Computers in
Human Behavior, 54:34–42.
Chen, Y., Xie, X., Lin, S.-D., and Chiu, A. (2018). Wsdm
cup 2018: Music recommendation and churn predic-
tion. In Proceedings of the Eleventh ACM Interna-
tional Conference on Web Search and Data Mining,
pages 8–9. ACM.
Choi, Y., Lee, Y., Shin, D., Cho, J., Park, S., Lee, S., Baek,
J., Kim, B., and Jang, Y. (2019). Ednet: A large-
scale hierarchical dataset in education. arXiv preprint
arXiv:1912.03072.
Dar
´
oczy, B., Vaderna, P., and Bencz
´
ur, A. (2015). Machine
learning based session drop prediction in lte networks
and its son aspects. In 2015 IEEE 81st Vehicular Tech-
nology Conference (VTC Spring), pages 1–5. IEEE.
Feng, W., Tang, J., and Liu, T. X. (2019). Understanding
dropouts in moocs.
Gehring, J., Auli, M., Grangier, D., Yarats, D., and
Dauphin, Y. N. (2017). Convolutional sequence to se-
quence learning. In Proceedings of the 34th Interna-
tional Conference on Machine Learning-Volume 70,
pages 1243–1252. JMLR. org.
Glorot, X. and Bengio, Y. (2010). Understanding the diffi-
culty of training deep feedforward neural networks.
In Proceedings of the thirteenth international con-
ference on artificial intelligence and statistics, pages
249–256.
Halawa, S., Greene, D., and Mitchell, J. (2014). Dropout
prediction in moocs using learner activity features.
Proceedings of the second European MOOC stake-
holder summit, 37(1):58–65.
Halfaker, A., Keyes, O., Kluver, D., Thebault-Spieker, J.,
Nguyen, T., Shores, K., Uduwage, A., and Warncke-
Wang, M. (2015). User session identification based on
strong regularities in inter-activity time. In Proceed-
ings of the 24th International Conference on World
Wide Web, pages 410–418. International World Wide
Web Conferences Steering Committee.
Hansen, C., Hansen, C., Alstrup, S., Simonsen, J. G., and
Lioma, C. (2019). Modelling sequential music track
skips using a multi-rnn approach. arXiv preprint
arXiv:1903.08408.
Harman, B. A. and Sato, T. (2011). Cell phone use and
grade point average among undergraduate university
students. College Student Journal, 45(3):544–550.
Huang, B., Kechadi, M. T., and Buckley, B. (2012). Cus-
tomer churn prediction in telecommunications. Expert
Systems with Applications, 39(1):1414–1425.
Hussain, M., Zhu, W., Zhang, W., Abidi, S. M. R., and Ali,
S. (2019). Using machine learning to predict student
difficulties from learning session data. Artificial Intel-
ligence Review, 52(1):381–407.
Junco, R. (2012). Too much face and not enough books:
The relationship between multiple indices of facebook
use and academic performance. Computers in human
behavior, 28(1):187–198.
Kawale, J., Pal, A., and Srivastava, J. (2009). Churn predic-
tion in mmorpgs: A social influence based approach.
In 2009 International Conference on Computational
Science and Engineering, volume 4, pages 423–428.
IEEE.
Kingma, D. P. and Ba, J. (2014). Adam: A
method for stochastic optimization. arXiv preprint
arXiv:1412.6980.
Lee, Y., Choi, Y., Cho, J., Fabbri, A. R., Loh, H., Hwang, C.,
Lee, Y., Kim, S.-W., and Radev, D. (2019). Creating a
neural pedagogical agent by jointly learning to review
and assess.
Liang, J., Li, C., and Zheng, L. (2016a). Machine learning
application in moocs: Dropout prediction. In 2016
11th International Conference on Computer Science
& Education (ICCSE), pages 52–57. IEEE.
Liang, J., Yang, J., Wu, Y., Li, C., and Zheng, L. (2016b).
Big data application in education: dropout prediction
in edx moocs. In 2016 IEEE Second International
Conference on Multimedia Big Data (BigMM), pages
440–443. IEEE.
Liu, Q., Tong, S., Liu, C., Zhao, H., Chen, E., Ma, H.,
and Wang, S. (2019). Exploiting cognitive structure
for adaptive learning. In Proceedings of the 25th
ACM SIGKDD International Conference on Knowl-
edge Discovery & Data Mining, KDD ’19, pages 627–
635, New York, NY, USA. ACM.
Liu, Z., Xiong, F., Zou, K., and Wang, H. (2018). Predicting
learning status in moocs using lstm. arXiv preprint
arXiv:1808.01616.
Lykourentzou, I., Giannoukos, I., Nikolopoulos, V., Mpar-
dis, G., and Loumos, V. (2009). Dropout prediction
in e-learning courses through the combination of ma-
chine learning techniques. Computers & Education,
53(3):950–965.
M
´
arquez-Vera, C., Cano, A., Romero, C., Noaman, A.
Y. M., Mousa Fardoun, H., and Ventura, S. (2016).
Early dropout prediction using data mining: a case
study with high school students. Expert Systems,
33(1):107–124.
Pandey, S. and Karypis, G. (2019). A self-attentive
model for knowledge tracing. arXiv preprint
arXiv:1907.06837.
Pappada, S. M., Cameron, B. D., Rosman, P. M., Bourey,
R. E., Papadimos, T. J., Olorunto, W., and Borst, M. J.
(2011). Neural network-based real-time prediction of
glucose in patients with insulin-dependent diabetes.
Diabetes technology & therapeutics, 13(2):135–141.
Reddy, S., Levine, S., and Dragan, A. (2017). Accelerating
human learning with deep reinforcement learning. In
NIPS’17 Workshop: Teaching Machines, Robots, and
Humans.
Reich, J. and Ruip
´
erez-Valiente, J. A. (2019). The mooc
pivot. Science, 363(6423):130–131.
CSEDU 2020 - 12th International Conference on Computer Supported Education
34