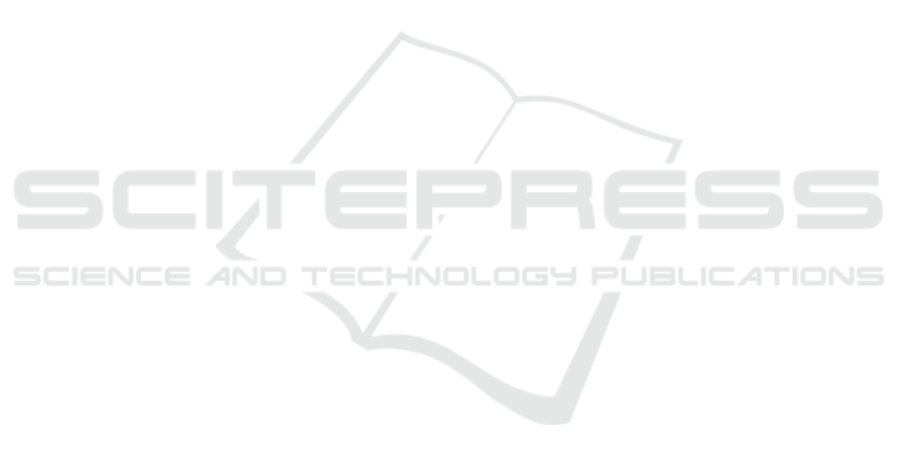
REFERENCES
Albuquerque, A., Amador, T., Ferreira, R., Veloso, A., and
Ziviani, N. (2018). Learning to rank with deep autoen-
coder features. In 2018 International Joint Conference
on Neural Networks (IJCNN), pages 1–8. IEEE.
Arima, S., Sumita, U., and Yoshii, J. (2012). Development
of sequential association rules for preventing minor-
stoppages in semi-conductor manufacturing. In Pro-
ceedings of the International Conference on Oper-
ations Research and Enterprise Systems (ICORES),
pages 349–354.
Belgiu, M. and Dr
˘
agut¸, L. (2016). Random forest in remote
sensing: A review of applications and future direc-
tions. ISPRS Journal of Photogrammetry and Remote
Sensing, 114:24–31.
Breiman, L. (2001). Random forests. Machine learning,
45(1):5–32.
Bulla, J., Orjuela-Ca
˜
n
´
on, A. D., and Fl
´
orez, O. D. (2018).
Feature extraction analysis using filter banks for faults
classification in induction motors. In 2018 Interna-
tional Joint Conference on Neural Networks (IJCNN),
pages 1–6. IEEE.
Calimeri, F., Marzullo, A., Stamile, C., and Terracina, G.
(2018). Graph based neural networks for automatic
classification of multiple sclerosis clinical courses. In
European Symposium on Artificial Neural Networks,
Computational Intelligence and Machine Learning
(ESANN).
Desa (2014). World urbanization prospects, the 2011 revi-
sion. Population Division, Department of Economic
and Social Affairs, United Nations Secretariat.
Ebeling, T. (2011). Condition monitoring for elevators–an
overview. Lift Report, 6:25–26.
Ebeling, T. and Haul, M. (2016). Results of a field trial aim-
ing at demonstrating the permanent detection of ele-
vator wear using intelligent sensors. In Proc. ELEV-
CON, pages 101–109.
Ferhatosmanoglu, N. and Macit, B. (2016). Incorporating
explanatory effects of neighbour airports in forecast-
ing models for airline passenger volumes. In Proceed-
ings of the International Conference on Operations
Research and Enterprise Systems (ICORES), pages
178–185.
Fern
´
andez-Varela, I., Athanasakis, D., Parsons, S.,
Hern
´
andez-Pereira, E., and Moret-Bonillo, V. (2018).
Sleep staging with deep learning: a convolutional
model. In Proceedings of the European Symposium
on Artificial Neural Networks, Computational Intelli-
gence and Machine Learning (ESANN).
Fogelman-Soulie, F., Robert, Y., and Tchuente, M. (1987).
Automata networks in computer science: theory and
applications. Manchester University Press and Prince-
ton University Press.
H
¨
anninen, J. and K
¨
arkk
¨
ainen, T. (2016). Comparison
of four-and six-layered configurations for deep net-
work pretraining. In European Symposium on Artifi-
cial Neural Networks, Computational Intelligence and
Machine Learning (ESANN).
Huet, R., Courty, N., and Lef
`
evre, S. (2016). A new penal-
isation term for image retrieval in clique neural net-
works. In European Symposium on Artificial Neural
Networks, Computational Intelligence and Machine
Learning (ESANN).
Huynh, T., Gao, Y., Kang, J., Wang, L., Zhang, P., Lian,
J., and Shen, D. (2016). Estimating ct image from
mri data using structured random forest and auto-
context model. IEEE transactions on medical imag-
ing, 35(1):174.
Japkowicz, N., Hanson, S. J., and Gluck, M. A. (2000).
Nonlinear autoassociation is not equivalent to pca.
Neural computation, 12(3):531–545.
Jia, F., Lei, Y., Lin, J., Zhou, X., and Lu, N. (2016). Deep
neural networks: A promising tool for fault character-
istic mining and intelligent diagnosis of rotating ma-
chinery with massive data. Mechanical Systems and
Signal Processing, 72:303–315.
Jiang, G., Xie, P., He, H., and Yan, J. (2018). Wind turbine
fault detection using a denoising autoencoder with
temporal information. IEEE/ASME Transactions on
Mechatronics, 23(1):89–100.
Jing, L., Wang, T., Zhao, M., and Wang, P. (2017). An adap-
tive multi-sensor data fusion method based on deep
convolutional neural networks for fault diagnosis of
planetary gearbox. Sensors, 17(2):414.
Lee, C.-C. (2014). Gender classification using m-estimator
based radial basis function neural network. In Signal
Processing and Multimedia Applications (SIGMAP),
2014 International Conference on, pages 302–306.
IEEE.
Liu, Z., Tang, B., He, X., Qiu, Q., and Liu, F. (2017).
Class-specific random forest with cross-correlation
constraints for spectral–spatial hyperspectral image
classification. IEEE Geoscience and Remote Sensing
Letters, 14(2):257–261.
Madani, P. and Vlajic, N. (2018). Robustness of deep
autoencoder in intrusion detection under adversarial
contamination. In Proceedings of the 5th Annual Sym-
posium and Bootcamp on Hot Topics in the Science of
Security, page 1. ACM.
Mart
´
ınez-Rego, D., Fontenla-Romero, O., and Alonso-
Betanzos, A. (2011). Power wind mill fault detection
via one-class ν-svm vibration signal analysis. In Neu-
ral Networks (IJCNN), The 2011 International Joint
Conference on, pages 511–518. IEEE.
Mendes, L. P., Dias, J., and Godinho, P. (2012). Bi-level
clustering in telecommunication fraud. In Proceed-
ings of the International Conference on Operations
Research and Enterprise Systems (ICORES), pages
126–131.
Mishra, K. M., Krogerus, T., and Huhtala, K. (2019). Fault
detection of elevator systems using deep autoencoder
feature extraction. In Research Challenges in Infor-
mation Science (RCIS), 2019 13th International Con-
ference on, pages 43–48. IEEE.
Sedlak, O., Cileg, M., and Kis, T. (2013). Decision support
system with mark-giving method. In Proceedings of
the International Conference on Operations Research
and Enterprise Systems (ICORES), pages 338–342.
ND2A 2020 - Special Session on Nonlinear Data Analysis and Applications
386