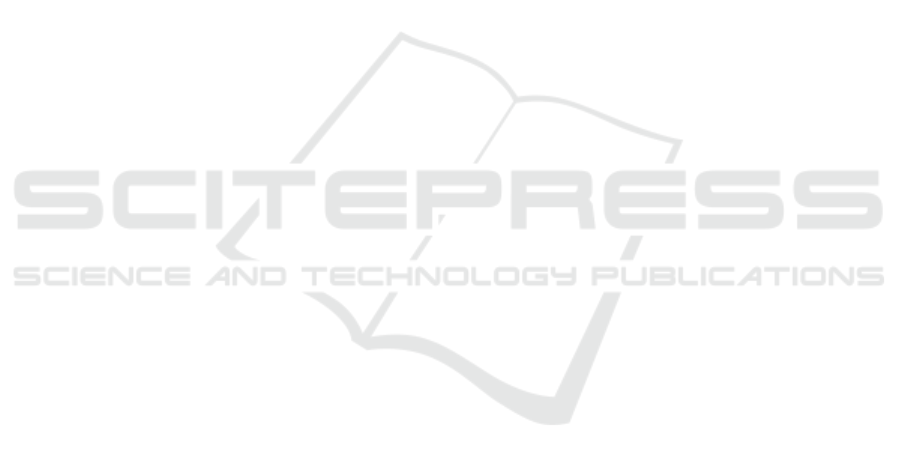
This approach can be used to generate synthetic in-
formation on the amount of HHC-demanding peo-
ple and potential service requests (order data) and al-
lows for the transformation of individual care demand
into specific service requests for HHC providers. For
the prediction of care demand, two perspectives need
to be considered: The forecast of individual care-
dependency, i.e., the prediction of the amount and lo-
cation of all HHC-demanding people in the investi-
gated area, and forecasts of the demand for specific
care services, i.e., the prediction of potentially re-
quested services. The combination of both perspec-
tives allows for the determination of the individual
care demand.
Forecasting Individual Care-dependency. This per-
spective allows for determining the number of care
recipients that choose HHC services for their care
support at an arbitrary (future) point in time. Here,
ABMS generates location, level of care, and further
information about the care recipient’s family situa-
tion. ABMS is an iterative approach and consists
of three steps. The first step is the generation of
an artificial population. Sociodemographic and care
statistic data are used to generate an artificial popu-
lation that matches all required structural characteris-
tics of the real population, e.g., age structure. Further-
more, the combination of map data, census data, and
geo-referencing approaches allows for the allocation
of the artificial population to real-world households
(Lebherz et al., 2018). In the second step, DM is used
to predict the stepwise development of this popula-
tion into the future (Li and O’Donoghue, 2013). This
allows for the investigation of the demographic de-
velopment as well as of each individuals’ care status,
level of care, and family situation. In the third step,
ABSS is used to simulate individual care decisions
and the preferred type of care for every care depen-
dent person. A simulative decision-making process
based on objective and subjective criteria is used for
analyzing care decisions. Influences that potentially
affect this decision are implemented as different func-
tions, e.g., saving of cost or social steadiness, which
are objectively interpreted depending on each individ-
uals’ situation. By this means, data about the individ-
ual care level or living situation can be integrated into
the decision-making process. Finally, an individual
subjective assessment of these results allows for de-
riving unique decisions based on the individual per-
son’s characteristics (Lebherz et al., 2018). After a
determined number of iterations, the output is a set
containing information on every care recipient, its de-
mographic characteristics, and its choice of care. For
the application in the presented framework only those
recipients choosing HHC are considered.
Forecasting the Demand for Specific Care Ser-
vices. The second perspective pursues two different
goals. The first one is a realistic assessment of spe-
cific services that are offered by HHC providers based
on the care recipient’s level of care. To this end, data
of service providers is required about the types of ser-
vices that are requested by care recipients depending
on their level of care. In a following step, quantitative
forecasting methods are used for the prediction of fu-
ture service requests. Based on this forecast, methods
for probability distribution fitting can be used to cal-
culate probabilities of the demand for every specific
care service (n) combined with every possible level
of care (m). Hence, the first result is a n × m matrix
consisting of probabilities for requesting a service.
The second goal is to determine which services
are usually provided by family members or friends
based on the assumption that even if a care recipi-
ent chooses HHC, some tasks are performed by fam-
ily members or friends. Such information must be
gathered through empirical studies, e.g., interviews.
Hence, as a second result of this perspective, prob-
abilities about the professional and non-professional
provision of services must be collected. In summary,
this perspective provides two different results, which
can be combined as probability matrices.
Forecasting Individual Care Demand and Order
Data. The set of HHC recipients combined with
the calculated probability matrices enables the fore-
cast of individual care demands. A random process
is used to assign possible services to the recipients
based on the provided probabilities. The probabilities
regarding the professional or non-professional provi-
sion of services are used in a second random process,
which transforms the demand forecast into realistic
order data for potential HHC providers. Here, ran-
domly chosen services are requested from the service
provider. Final order data consists of a list of HHC re-
cipients with all data provided by ABMS and two in-
dividual sets of services: HHC provider requests and
services provided by family or friends.
3.3 Modern Home Health Care
Logistics Management
Some management tasks in HHC are highly com-
plex or require great coordination effort. The idea of
modern HHC logistics management is to reduce the
range of such tasks by means of innovative informa-
tion systems, to create more space for social-related
tasks concerning customers and employees, like sen-
sitive human-to-human interaction in leading a team.
The vision comprises an Intelligent Assistance Sys-
tem for decision support and a Multiagent Control
ICT4AWE 2020 - 6th International Conference on Information and Communication Technologies for Ageing Well and e-Health
230