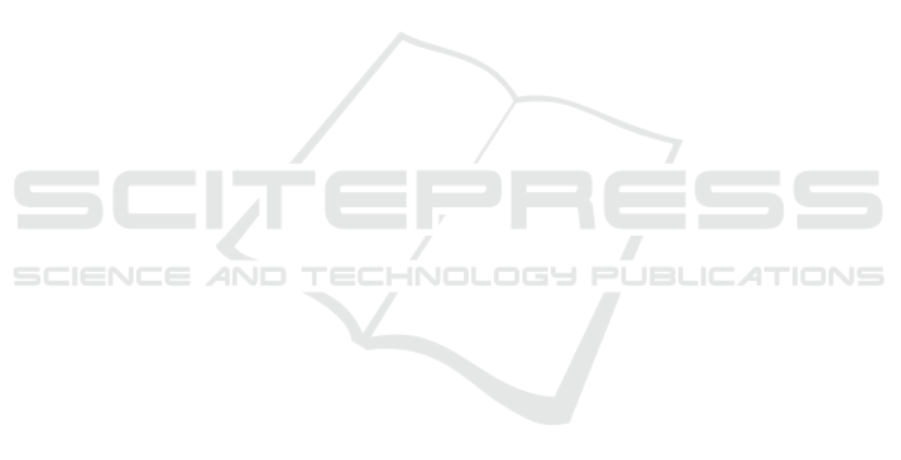
ticed that collaboration could achieve a cost reduction
of near 45% compared with individual routing, along
with different satisfaction levels. However, when per-
sonalized goals are considered, the overall saving is
comparatively reduced, but better levels of satisfac-
tion are obtained. Therefore, considering the egali-
tarian approach that guarantees an equal level of ser-
vice and satisfaction may encourage different LSPs to
work towards further collaboration on several levels.
REFERENCES
Barbucha, D. (2012). Search modes for the cooperative
multi-agent system solving the vehicle routing prob-
lem. Neurocomputing, 88:13–23.
Barbucha, D. (2014). A cooperative population learning
algorithm for vehicle routing problem with time win-
dows. Neurocomputing, 146:210–229.
Baykaso
˘
glu, A. and Kaplano
˘
glu, V. (2015). An applica-
tion oriented multi-agent based approach to dynamic
load/truck planning. Expert Systems with Applica-
tions, 42(15-16):6008–6025.
Cattaruzza, D., Absi, N., Feillet, D., and Vidal, T. (2014).
A memetic algorithm for the multi trip vehicle routing
problem. European Journal of Operational Research,
236(3):833–848.
Chatterjee, R., Greulich, C., and Edelkamp, S. (2016). Op-
timizing last mile delivery using public transport with
multi-agent based control. In 2016 IEEE 41st Confer-
ence on Local Computer Networks Workshops (LCN
Workshops), pages 205–212. IEEE.
Chinh, N. Q., Kim, H. C., Siwei, J., and NengSheng, Z.
(2016). Collaborative vehicle routing problem for ur-
ban last-mile logistics. In 2016 IEEE International
Conference on Systems, Man, and Cybernetics (SMC),
pages 001531–001536. IEEE.
Clarke, G. and Wright, J. W. (1964). Scheduling of vehicles
from a central depot to a number of delivery points.
Operations research, 12(4):568–581.
Cordeau, J.-F., Gendreau, M., and Laporte, G. (1997). A
tabu search heuristic for periodic and multi-depot ve-
hicle routing problems. Networks: An International
Journal, 30(2):105–119.
Cruijssen, F., Bräysy, O., Dullaert, W., Fleuren, H., and Sa-
lomon, M. (2007). Joint route planning under vary-
ing market conditions. International Journal of Physi-
cal Distribution & Logistics Management, 37(4):287–
304.
Czarnowski, I., J˛edrzejowicz, P., and Kacprzyk, J. (2013).
Agent-based optimization. Springer.
Dai, B. and Chen, H. (2011). A multi-agent and auction-
based framework and approach for carrier collabora-
tion. Logistics Research, 3(2-3):101–120.
Holdorf, S. and Haasis, H.-D. (2014). Last mile deliv-
ery concepts in e-commerce an empirical approach.
In The 8th International Conference on Software,
Knowledge, Information Management and Applica-
tions (SKIMA 2014), pages 1–6. IEEE.
Kalina, P., Vok
ˇ
rínek, J., and Ma
ˇ
rík, V. (2015). Agents
toward vehicle routing problem with time win-
dows. Journal of Intelligent Transportation Systems,
19(1):3–17.
Kumar, S. N. and Panneerselvam, R. (2012). A survey on
the vehicle routing problem and its variants. Intelli-
gent Information Management, 4(03):66.
Lahyani, R., Khemakhem, M., and Semet, F. (2015). Rich
vehicle routing problems: From a taxonomy to a def-
inition. European Journal of Operational Research,
241(1):1–14.
Martin, S., Ouelhadj, D., Beullens, P., Ozcan, E., Juan,
A. A., and Burke, E. K. (2016). A multi-agent based
cooperative approach to scheduling and routing. Eu-
ropean Journal of Operational Research, 254(1):169–
178.
Mingozzi, A., Roberti, R., and Toth, P. (2013). An exact
algorithm for the multitrip vehicle routing problem.
INFORMS Journal on Computing, 25(2):193–207.
Ouhader, H. and El Kyal, M. (2017). Combining facil-
ity location and routing decisions in sustainable ur-
ban freight distribution under horizontal collabora-
tion: how can shippers be benefited? Mathematical
Problems in Engineering, 2017.
Pisinger, D. and Ropke, S. (2007). A general heuristic for
vehicle routing problems. Computers & Operations
Research, 34(8):2403–2435.
Schrimpf, G., Schneider, J., Stamm-Wilbrandt, H., and
Dueck, G. (2000). Record breaking optimization re-
sults using the ruin and recreate principle. Journal of
Computational Physics, 159(2):139–171.
Schwarze, S. and Voß, S. (2013). Improved load balancing
and resource utilization for the skill vehicle routing
problem. Optimization Letters, 7(8):1805–1823.
Schwind, M., Gujo, O., and Vykoukal, J. (2009). A com-
binatorial intra-enterprise exchange for logistics ser-
vices. Information Systems and E-business Manage-
ment, 7(4):447–471.
Souza, G. R., Goldbarg, E. F., Goldbarg, M. C., and Canuto,
A. M. (2012). A multiagent approach for metaheuris-
tics hybridization applied to the traveling salesman
problem. In 2012 Brazilian Symposium on Neural
Networks, pages 208–213. IEEE.
Talukdar, S., Murthy, S., and Akkiraju, R. (2003). Asyn-
chronous teams. In Handbook of Metaheuristics,
pages 537–556. Springer.
Wang, X. and Kopfer, H. (2014). Collaborative transporta-
tion planning of less-than-truckload freight. OR Spec-
trum, 36(2):357–380.
Wang, X. and Kopfer, H. (2015). Rolling horizon planning
for a dynamic collaborative routing problem with full-
truckload pickup and delivery requests. Flexible Ser-
vices and Manufacturing Journal, 27(4):509–533.
Zhou, W., Song, T., He, F., and Liu, X. (2013). Multiobjec-
tive vehicle routing problem with route balance based
on genetic algorithm. Discrete Dynamics in Nature
and Society, 2013.
A Meta-heuristic based Multi-Agent Approach for Last Mile Delivery Problem
505