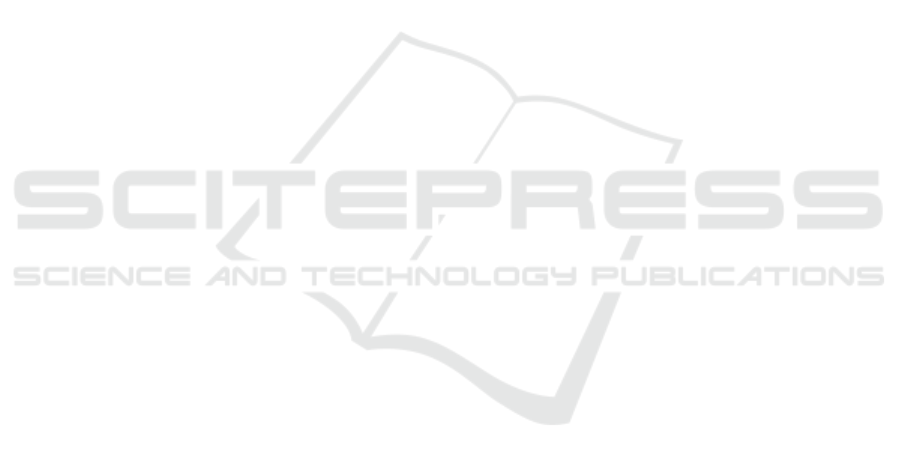
greater impact on the accuracy values compared to the
drowsy sequences, where blink intervals are longer.
Nevertheless, the overall accuracy is higher than other
results reported in related state-of-the-art methods.
Finally, the presented results suggest that in-
cluding our method in more complex blink de-
tection pipelines within Driver Monitoring Systems
(DMS) improves the overall detection accuracy with-
out adding significant computational overhead.
6 CONCLUSIONS
In this paper we have presented a method to obtain
an eyelid aperture signal, based on online amplitude
analysis, which enables driver-adaptive normalisation
of eye amplitudes obtained with face alignment meth-
ods. Its parameters have been trained using manually
labelled sequences and a proposed cost function with
minimisation mechanisms. The method has been im-
plemented within the framework of a Driver Monitor-
ing System (DMS) library. Experimental results show
real-time performance in different platforms used in
automation applications, which make it feasible for
integration in complex ADAS systems without signif-
icant computational overhead.
The method was evaluated using the proposed cost
function, which makes use of manually labelled data
samples. Comparison with simple baseline methods
was provided showing lower error cost. In addition,
the results of the classification problem for open and
closed eyes show higher accuracy and adaptability
to driver-specific visual features compared to other
state-of-the-art methods.
Our method can be incorporated into blink de-
tection pipelines to improve the estimation of blink
parameters while it also produces adaptive eyelid
aperture estimates valuable for subsequent driver’s
arousal state analysis. Future work include the valida-
tion of the method under a wide variety of use cases
and conditions, extending our current database.
ACKNOWLEDGEMENTS
This work has received funding from the European
Union’s H2020 research and innovation programme
(grant agreement n
o
690772, project VI-DAS).
REFERENCES
Asthana, A., Zafeiriou, S., Cheng, S., and Pantic, M.
(2014). Incremental face alignment in the wild. IEEE
Conference on Computer Vision and Pattern Recogni-
tion, CVPR, pages 1859–1866.
Baccour, M. H., Driewer, F., Kasneci, E., and Rosenstiel,
W. (2019). Camera-Based Eye Blink Detection Al-
gorithm for Assessing Driver Drowsiness. In IEEE
Intelligent Vehicles Symposium, pages 866–872.
Baltrusaitis, T., Robinson, P., and Morency, L. P. (2016).
OpenFace: An open source facial behavior analysis
toolkit. In 2016 IEEE Winter Conference on Applica-
tions of Computer Vision, WACV 2016.
Borghini, G., Astolfi, L., Vecchiato, G., Mattia, D., and Ba-
biloni, F. (2014). Measuring neurophysiological sig-
nals in aircraft pilots and car drivers for the assessment
of mental workload, fatigue and drowsiness. Neuro-
science and Biobehavioral Reviews, 44:58–75.
Boyle, L. N., Tippin, J., Paul, A., and Rizzo, M. (2008).
Driver performance in the moments surrounding a mi-
crosleep. Transportation Research Part F: Traffic Psy-
chology and Behaviour, 11(2):126–136.
Cabrall, C., Janssen, N., Goncalves, J., Morando, A., Sass-
man, M., and de Winter, J. (2016). Eye-based driver
state monitor of distraction, drowsiness, and cogni-
tive load for transitions of control in automated driv-
ing. 2016 IEEE International Conference on Systems,
Man, and Cybernetics (SMC), pages 001981–001982.
Daniluk, M., Rezaei, M., Nicolescu, R., and Klette, R.
(2014). Eye Status Based on Eyelid Detection : A
Driver Assistance System. In International Confer-
ence on Computer Vision and Graphics.
Danisman, T., Bilasco, I. M., Djeraba, C., and Ihaddadene,
N. (2010). Drowsy driver detection system using eye
blink patterns. In International Conference on Ma-
chine and Web Intelligence, ICMWI, pages 230–233.
Drutarovsky, T. and Fogelton, A. (2015). Eye blink detec-
tion using variance of motion vectors. In Proceed-
ings of 2th workshop on Assistive Computer Vision
and Robotics in ECCV 2014, volume 8927.
Ebrahim, P., Stolzmann, W., and Yang, B. (2013). Eye
movement detection for assessing driver drowsiness
by electrooculography. Proceedings - 2013 IEEE In-
ternational Conference on Systems, Man, and Cyber-
netics, SMC 2013, pages 4142–4148.
European Commission (2011). Roadmap to a Single Euro-
pean Transport Area–Towards a competitive and re-
source efficient transport system. Technical report,
European Commission.
Fogelton, A. and Benesova, W. (2016). Eye blink detection
based on motion vectors analysis. Computer Vision
and Image Understanding, 148:23–33.
Fridman, L., Lee, J., Reimer, B., and Victor, T. (2016).
Owl and Lizard: Patterns of Head Pose and Eye Pose
in Driver Gaze Classification. IET Computer Vision,
10(4):1–9.
Friedrichs, F. and Yang, B. (2010). Camera-based drowsi-
ness reference for driver state classification under real
driving conditions. In IEEE Intelligent Vehicles Sym-
posium, volume 4, pages 101–106.
Fuhl, W., Santini, T., and Kasneci, E. (2017). Fast & robust
eyelid outline & aperture detection in real-world sce-
User-adaptive Eyelid Aperture Estimation for Blink Detection in Driver Monitoring Systems
351