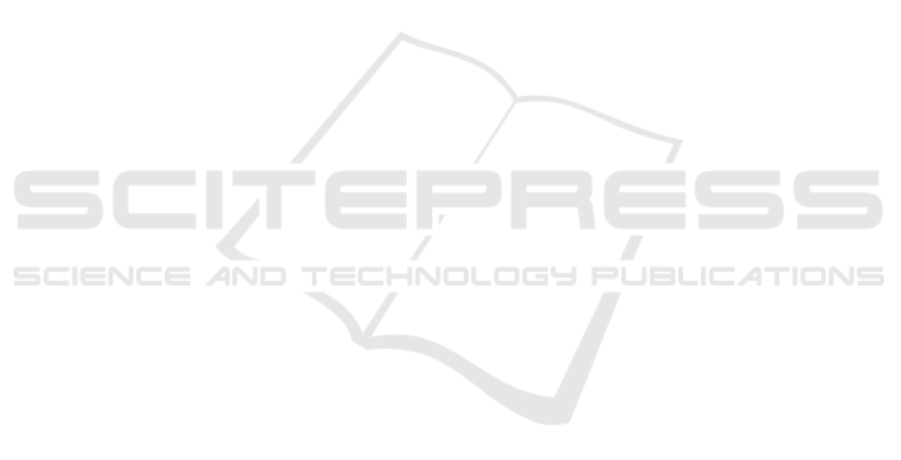
for the distributed learning process between the agent
and the human experts. This will propose a complete
concept of parallel learning. We are also planning to
optimise the learning data and dig deeper to explore
the nature of the prediction accuracy, and it is rele-
vant to the available data. Although our model intro-
duces faults tolerance and communication failure or
reduction, a comparative analysis with other systems
in (Makonin et al., 2016; Tecuci et al., 2007) using
real agents marked as future work. We will also look
at how the model and the predictions tools act in a
highly changing environment.
ACKNOWLEDGEMENTS
We appreciate the effort of Petroleum Technology
Trust Funds (PTDF) of Nigeria for sponsoring this
project.
REFERENCES
afrl rq (2019). https://github.com/afrl-rq/OpenAMASE.
afrl-rq. original-date: 2017-05-07T13:17:17Z.
Araragi, T. (2005). Fault tolerance for internet agent sys-
tems: In cases of stop failure and byzantine failure.
AAMAS ’05, page 123–124, New York, NY, USA.
ACM. event-place: The Netherlands.
Bevacqua, G., Cacace, J., Finzi, A., and Lippiello, V.
(2015). Mixed-initiative planning and execution
for multiple drones in search and rescue missions.
ICAPS’15, page 315–323. AAAI Press. event-place:
Jerusalem, Israel.
Bottou, L. (2010). Large-scale machine learning with
stochastic gradient descent. page 10.
Cacace, J., Finzi, A., and Lippiello, V. (2014). A mixed-
initiative control system for an aerial service vehi-
cle supported by force feedback. pages 1230–1235.
2014 IEEE/RSJ International Conference on Intelli-
gent Robots and Systems.
Chawla, M. and Duhan, M. (2018). Levy flights in meta-
heuristics optimization algorithms – a review. Applied
Artificial Intelligence, 32(9-10):802–821.
Dempster, A. P., Laird, N. M., and Rubin, D. B. (1977).
Maximum likelihood from incomplete data via the em
algorithm. Journal of the Royal Statistical Society. Se-
ries B (Methodological), 39(1):1–38.
Dernoncourt, F. (2013). Introduction to fuzzy logic.
page 24.
Endert, A., Hossain, M. S., Ramakrishnan, N., North, C.,
Fiaux, P., and Andrews, C. (2014). The human is the
loop: New directions for visual analytics. J. Intell. Inf.
Syst., 43(3):411–435.
Endsley, M. R. (1995). Toward a theory of situation aware-
ness in dynamic systems. [Online; accessed 2019-11-
14].
Ferguson, G. and Allen, J. (2007). Mixed-initiative sys-
tems for collaborative problem solving. AI Magazine,
28(2):23–23.
Green, T. M., Maciejewski, R., and DiPaola, S. (2010). Al-
ida: Using machine learning for intent discernment in
visual analytics interfaces. pages 223–224. 2010 IEEE
Symposium on Visual Analytics Science and Technol-
ogy. ISSN: null.
Ingle, L. B. (2011). Every day is fire day: A study of his-
toric fire towers and lookout life in the great smoky
mountains national park. page 136.
Kifor, T., Varga, L. Z., Vazquez-Salceda, J., Alvarez, S.,
Willmott, S., Miles, S., and Moreau, L. (2006). Prove-
nance in agent-mediated healthcare systems. IEEE In-
telligent Systems, 21(6):38–46.
Makonin, S., McVeigh, D., Stuerzlinger, W., Tran, K., and
Popowich, F. (2016). Mixed-initiative for big data:
The intersection of human + visual analytics + pre-
diction. pages 1427–1436. 2016 49th Hawaii Interna-
tional Conference on System Sciences (HICSS).
Rich, C. and Sidner, C. L. (2007). Diamondhelp: A generic
collaborative task guidance system. AI Magazine,
28(2):33–33.
Rich, C., Sidner, C. L., and Lesh, N. (2001). Collagen:
Applying collaborative discourse theory to human-
computer interaction. AI Magazine, 22(4):15–15.
Romanycia, M. (2019). Netica-j reference manual. page
119.
Stanton, N. A., Stewart, R., Harris, D., Houghton, R. J.,
Baber, C., McMaster, R., Salmon, P., Hoyle, G.,
Walker, G., Young, M. S., Linsell, M., Dymott, R.,
and Green, D. (2006). Distributed situation awareness
in dynamic systems: theoretical development and ap-
plication of an ergonomics methodology. Ergonomics,
49(12-13):1288–1311.
Tecuci, G., Boicu, M., and Cox, M. T. (2007). Seven as-
pects of mixed-initiative reasoning:an introduction to
this special issue on mixed-initiative assistants. AI
Magazine, 28(2):11–11.
Turpin, M., Michael, N., and Kumar, V. (2014). Capt: Con-
current assignment and planning of trajectories for
multiple robots. The International Journal of Robotics
Research, 33(1):98–112.
Yue, J., Raja, A., and Ribarsky, W. (2010). Predictive ana-
lytics using a blackboard-based reasoning agent. vol-
ume 2, pages 97–100. 2010 IEEE/WIC/ACM Interna-
tional Conference on Web Intelligence and Intelligent
Agent Technology. ISSN: null.
HAMT 2020 - Special Session on Human-centric Applications of Multi-agent Technologies
354