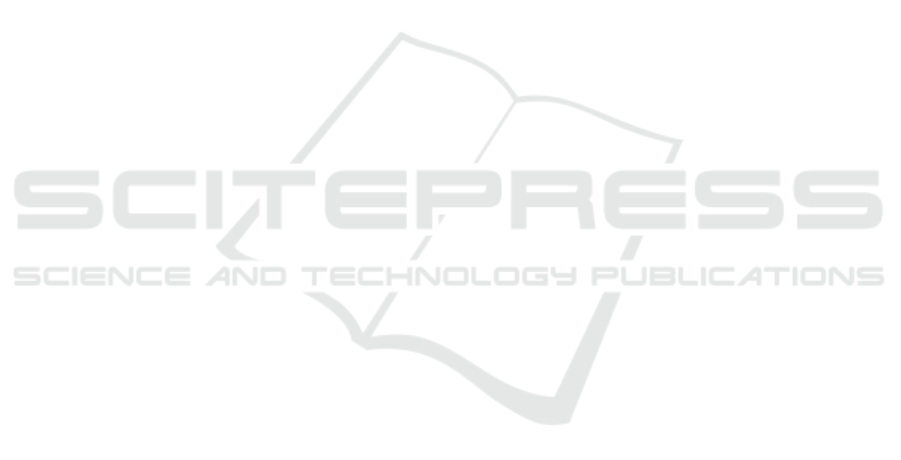
Bruno, E., Iacoviello, G., and Lazzini, A. (2015). On the
possible tools for the prevention of non-performing
loans. a case study of an italian bank. Corporate Own-
ership and Control, 5(1):7–19.
Canfora, G., Martinelli, F., Mercaldo, F., Nardone, V., San-
tone, A., and Visaggio, C. A. (2018). Leila: formal
tool for identifying mobile malicious behaviour. IEEE
Transactions on Software Engineering.
Ceccarelli, M., Cerulo, L., and Santone, A. (2014). De
novo reconstruction of gene regulatory networks from
time series data, an approach based on formal meth-
ods. Methods, 69(3):298–305.
Chitra, K. and Subashini, B. (2013). Data mining tech-
niques and its applications in banking sector. Interna-
tional Journal of Emerging Technology and Advanced
Engineering, 3(8):219–226.
Cimitile, A., Martinelli, F., and Mercaldo, F. (2017a). Ma-
chine learning meets ios malware: Identifying mali-
cious applications on apple environment. In ICISSP,
pages 487–492.
Cimitile, A., Martinelli, F., Mercaldo, F., Nardone, V.,
and Santone, A. (2017b). Formal methods meet mo-
bile code obfuscation identification of code reorder-
ing technique. In Enabling Technologies: Infrastruc-
ture for Collaborative Enterprises (WETICE), 2017
IEEE 26th International Conference on, pages 263–
268. IEEE.
EBA (2011). European banking authority,eba guidelines on
internal governance, london. http://webhome.cs.uvic.
ca/
∼
bmkapron/ccs.html.
FBS (2017). Financial stability board , artificial intelligence
and machine learning in financial services. available
at: http://www. fsb. org/2017/11/artificialintelligence-
and-machine-learning-in-financialservice/.
Francesco, N. d., Lettieri, G., Santone, A., and Vaglini, G.
(2014). Grease: a tool for efficient “nonequivalence”
checking. ACM Transactions on Software Engineer-
ing and Methodology (TOSEM), 23(3):24.
Frawley, W. J., Piatetsky-Shapiro, G., and Matheus, C. J.
(1992). Knowledge discovery in databases: An
overview. AI magazine, 13(3):57–57.
Giovannoni, E., Quarchioni, S., and Riccaboni, A. (2016).
The role of roles in risk management change: The
case of an italian bank. European Accounting Review,
25(1):109–129.
Goldstein, I., Jiang, W., and Karolyi, G. A. (2019). To fin-
tech and beyond. The Review of Financial Studies,
32(5):1647–1661.
Gradara, S., Santone, A., and Villani, M. L. (2005). Using
heuristic search for finding deadlocks in concurrent
systems. Information and Computation, 202(2):191–
226.
Jordan, M. I. and Mitchell, T. M. (2015). Machine learn-
ing: Trends, perspectives, and prospects. Science,
349(6245):255–260.
Kruppa, J., Schwarz, A., Arminger, G., and Ziegler, A.
(2013). Consumer credit risk: Individual probabil-
ity estimates using machine learning. Expert Systems
with Applications, 40(13):5125–5131.
Lessmann, S., Baesens, B., Seow, H.-V., and Thomas,
L. C. (2015). Benchmarking state-of-the-art classi-
fication algorithms for credit scoring: An update of
research. European Journal of Operational Research,
247(1):124–136.
Mackinnon, M. J. and Glick, N. (1999). Applications:
Data mining and knowledge discovery in databases–
an overview. Australian & New Zealand Journal of
Statistics, 41(3):255–275.
Maiorca, D., Mercaldo, F., Giacinto, G., Visaggio, C. A.,
and Martinelli, F. (2017). R-packdroid: Api package-
based characterization and detection of mobile ran-
somware. In Proceedings of the Symposium on Ap-
plied Computing, pages 1718–1723. ACM.
Martinelli, F., Marulli, F., and Mercaldo, F. (2017a). Eval-
uating convolutional neural network for effective mo-
bile malware detection. Procedia Computer Science,
112:2372–2381.
Martinelli, F., Mercaldo, F., and Saracino, A. (2017b).
Bridemaid: An hybrid tool for accurate detection of
android malware. In Proceedings of the 2017 ACM on
Asia Conference on Computer and Communications
Security, pages 899–901. ACM.
Mercaldo, F., Nardone, V., and Santone, A. (2017). Dia-
betes mellitus affected patients classification and diag-
nosis through machine learning techniques. Procedia
computer science, 112:2519–2528.
Mercaldo, F., Nardone, V., Santone, A., and Visaggio, C. A.
(2016). Hey malware, i can find you! In Enabling
Technologies: Infrastructure for Collaborative Enter-
prises (WETICE), 2016 IEEE 25th International Con-
ference on, pages 261–262. IEEE.
Mitchell, T. M. (1999). Machine learning and data mining.
Communications of the ACM, 42(11).
Onay, C. and
¨
Ozt
¨
urk, E. (2018). A review of credit scoring
research in the age of big data. Journal of Financial
Regulation and Compliance, 26(3):382–405.
Santone, A. (2002). Automatic verification of concur-
rent systems using a formula-based compositional ap-
proach. Acta Informatica, 38(8):531–564.
Santone, A. (2011). Clone detection through process alge-
bras and java bytecode. In IWSC, pages 73–74. Cite-
seer.
Saunders, A. and Allen, L. (2010). Credit risk management
in and out of the financial crisis: new approaches to
value at risk and other paradigms, volume 528. John
Wiley & Sons.
Tsai, C.-F. and Chen, M.-L. (2010). Credit rating by hybrid
machine learning techniques. Applied soft computing,
10(2):374–380.
Van Gestel, T. and Baesens, B. (2008). Credit Risk Man-
agement: Basic concepts: Financial risk components,
Rating analysis, models, economic and regulatory
capital. OUP Oxford.
Wilson, J. O., Casu, B., Girardone, C., and Molyneux, P.
(2010). Emerging themes in banking: Recent liter-
ature and directions for future research. The British
Accounting Review, 42(3):153–169.
Bank Credit Risk Management based on Data Mining Techniques
843