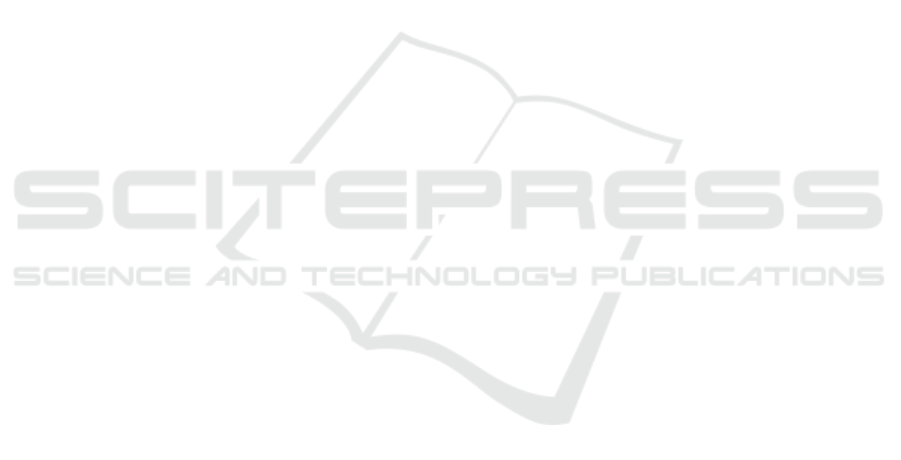
websites that integrated the connect service library.
The aim of this research was to investigate clusters
among the users who show similar patterns of engage-
ment with the learning materials across the before-
mentioned websites.
To clearly draw the line of the study, the following
research questions have been asked:
1. RQ1: Are there any recognisable engagement pat-
tern which can be used for clustering the users by
applying learning analytics?
2. RQ2: If so, what are the main differences per clus-
ter?
3. RQ3: Are these patterns distinctive by OER
repositories?
The activities of users were divided into sessions
by checking out whether or not the time passed be-
tween two visits is no more than 2 hours. Two nu-
meric factors indicating the feature of the engage-
ment, number of materials visited in a session and
number of visits made in a session, are used.
In order to answer RQ1, k-means clustering
method has been used. Five clusters have been de-
tected based on number of materials visited in a ses-
sion and number of clicks made in a session (see Fig-
ure 4).
The main distinctive differences between the clus-
ters are i) number of repositories seen in a cluster and
ii) drop in single page views after Cluster 1, and iii)
increasing longer and sequential view of OER mate-
rials from Cluster 1 to Cluster 5.
It is observed that the pattern of engagement varies
by clusters. The users on the online learning platform
of the Universitat Polit
´
ecnica de Val
´
encia (UPV) have
been seen in only Clusters 1 to 3. The users on Vide-
olectures.NET (VL) and eU
ˇ
cbeniki were detected in
every single cluster while the users on VL were
mostly in the first two clusters, users on eU
ˇ
cbeniki
were dominant in Clusters 3 to 5. This results answer
RQ3, even though we believe that there is a room for
a detail investigation on each platform.
In conclusion, the contributions of our paper can
be summarised as follows:
• Users can be grouped, in our case it was into five
clusters, based on the number of materials they
interacted with and the number of transitions they
made within a certain time period.
• Users on the same OER provider usually show
similar patterns of engagement. For example,
users on UPV have only be seen in the first three
clusters so that they never showed a sequential en-
gagement with the materials.
• The design of materials might have an effect on
the pattern of engagement. For example, users on
eU
ˇ
cbeniki are usually clustered in the last three
clusters where there is a sequential paths extracted
from the users’ transitions amongst many mate-
rials. eU
ˇ
cbeniki is also designed as a sequential
lecture models directing users to the next page
after study the current page. Even though same
users on Videolectures.NET showed the same pat-
tern, they are usually seen in the first two clusters
where single page views or shorter paths occurred
as relatively longer videos are available on Vide-
olectures.NET.
The future direction of this research is to com-
plete the analysis by using the semantic relationship
between the OER materials to more meaningfully ad-
dress the users’ learning pathways across the clusters.
A final direction of this research would be to use the
gathered information by learning analytics in improv-
ing our existing recommendation system and encour-
age other OER repositories to integrate the system
into their website for their visitors. We also intend to
share our final model for cross-site engagement pat-
tern detection so that other OER repositories can re-
use it and integrate in their system as we believe open
cross-site systems will be more demanded in the fol-
lowing decades.
ACKNOWLEDGEMENTS
This work was supported by the Slovenian Research
Agency and X5GON European Unions Horizon 2020
project under grant agreement No: 761758.
REFERENCES
de Barba, P. G., Malekian, D., Oliveira, E. A., Bailey, J.,
Ryan, T., and Kennedy, G. (2019). The importance
and meaning of session behaviour in a massive open
online course. Computers & Education, page 103772.
Dernoncourt, F., Taylor, C., O’Reilly, U.-M., Veeramacha-
neni, K., Wu, S., Do, C., and Halawa, S. (2013).
Moocviz: A large scale, open access, collaborative,
data analytics platform for moocs. In NIPS work-
shop on data-driven education, Lake Tahoe, Nevada.
Retrieved from http://groups. csail. mit. edu/EVO-
DesignOpt/groupWebSite/uploads/Site/MoocViz. pdf.
Drachsler, H. and Kalz, M. (2016). The mooc and learning
analytics innovation cycle (molac): a reflective sum-
mary of ongoing research and its challenges. Journal
of Computer Assisted Learning, 32(3):281–290.
Huang, H., Zhao, B., Zhao, H., Zhuang, Z., Wang, Z.,
Yao, X., Wang, X., Jin, H., and Fu, X. (2018). A
cross-platform consumer behavior analysis of large-
scale mobile shopping data. In Proceedings of the
CSEDU 2020 - 12th International Conference on Computer Supported Education
94