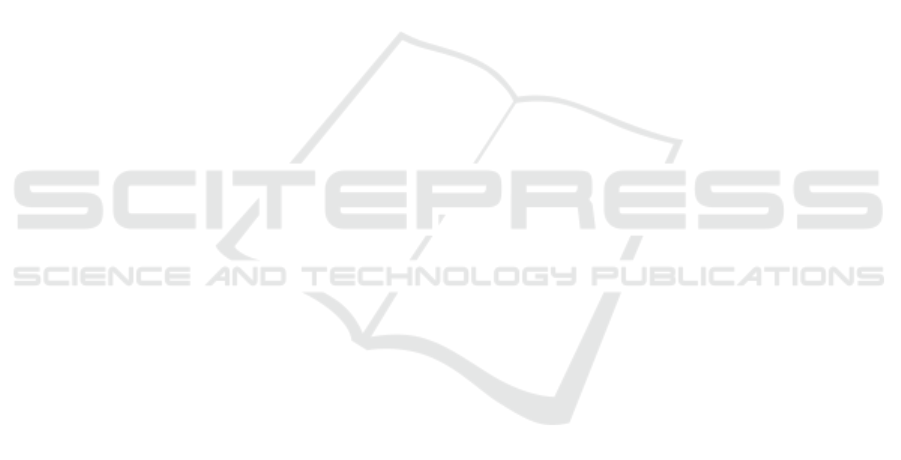
FCA and interventions were directed to the same di-
rections, this means that both approaches produced
the same causal DAG. Thus, it is possible to observe
that the interventions validate the results obtained us-
ing FCA.
The DAG shown in Fig. 6 is expected to be the
true causal network. In this sense, this DAG was pre-
sented to a specialist in order to validate its correct-
ness.
According to the expert, in a causal interpretation,
global infection does not cause local infection, be-
cause it is matter of temporal order. First come the
local infection and after global infection. Therefore,
the direction of the edge between nodes 10 and 11,
can only be 11 → 10.
Considering the bi-directed edge nodes 7 (Emer-
gency) and 8 (ASA), ASA is a classification, from 1 to
6, for assessing the health of the patient. The higher is
the number, worse is his health stands. Thus, there is a
relationship between these two attributes, which may
have a common cause or a relationship of causality
between them, once that how worse is patient’s con-
dition, more urgent became the surgery. For example,
according to (Aronson WL, 2003), in the original ver-
sion of ASA from 1941, ASA class 5 indicates ”Emer-
gencies that would otherwise be graded in Class 1 or
Class 2.”. Nowadays in each class of ASA is added a
letter E indicating if it is an emergency surgery or not.
Therefore, it is reasonable that the direction of the
edge between ASA and Emergency goes from ASA
to Emergency, not the opposite, once that ASA may
have direct effect on the emergency of the surgery, but
it is important to highlight that it is not the only factor
that influences the urgency of the surgery.
The relationship between attributes In-
fected.surgery (5) and general.anesthesia (6) is
correlated, according to the specialist, but it is not
possible to say that one causes another.
4 CONCLUSIONS
The main goal of this article was combining Causal
Inference and Formal Concept Analysis to establish
causality relationship between random variables. In
this sense we can conclude that, once causality re-
quires interventions or background knowledge to de-
fine the true DAG, FCA seems an alternative to help
in identifying the causal relationship.
Even if the implication rule does not necessarily
mean causality, it is useful in identifying relationships
among random variables through attribute implica-
tions. Therefore, the FCA can be used as a heuristic to
direct edges when the Bayesian learners’ algorithms
were unable to orient the edges between the vertices.
As future work, one should apply this heuristic in
other real applications using different type of data,
numerical for example, and create an algorithm that
combine these two theories, Causal Inference and
FCA. The researcher can also compare the results ob-
tained with others approaches of directing edges when
the true graph is unknown.
REFERENCES
Aronson WL, McAuliffe MS, M. K. (2003). Variability in
the american society of anesthesiologists physical sta-
tus classification scale. AANA Journal, 71(4):265–74.
Carpineto, C. and Romano, G. (2004). Concept Data Anal-
ysis: Theory and Applications. John Wiley &
Sons, Inc., USA.
de Carvalho, W. F. and Zarate, L. E. (2019). Causality re-
lationship among attributes applied in an educational
data set. In Proceedings of the 34th ACM/SIGAPP
Symposium on Applied Computing, SAC ’19, pages
1271–1277, New York, NY, USA. ACM.
Flesch, I. and Lucas, P. J. (2007). Markov Equivalence in
Bayesian Networks, pages 3–38. Springer Berlin Hei-
delberg, Berlin, Heidelberg.
Guyon, I. and Aliferis, C. F. (2007). Causal feature selec-
tion.
Hyttinen, A., Eberhardt, F., and J
¨
arvisalo, M. (2015). Do-
calculus when the true graph is unknown. In Proceed-
ings of the Thirty-First Conference on Uncertainty in
Artificial Intelligence, UAI’15, pages 395–404, Ar-
lington, Virginia, United States. AUAI Press.
Kalisch, M., M
¨
achler, M., Colombo, D., Maathuis, M., and
B
¨
uhlmann, P. (2012). Causal inference using graphi-
cal models with the r package pcalg. Journal of Sta-
tistical Software, Articles, 47(11):1–26.
ˇ
Skopljanac Ma
ˇ
cina, F. and Bla
ˇ
skovi
´
c, B. (2014). Formal
concept analysis – overview and applications. Proce-
dia Engineering, 69:1258 – 1267. 24th DAAAM In-
ternational Symposium on Intelligent Manufacturing
and Automation, 2013.
Neapolitan, R. E. (2003). Learning Bayesian Networks.
Prentice-Hall, Inc., Upper Saddle River, NJ, USA.
Pearl, J. (2009). Causality: Models, Reasoning and Infer-
ence. Cambridge University Press, New York, NY,
USA, 2nd edition.
Poelmans, J., Ignatov, D. I., Kuznetsov, S. O., and Dedene,
G. (2013). Formal concept analysis in knowledge pro-
cessing: A survey on applications. Expert Systems
with Applications, 40(16):6538 – 6560.
Shpitser, I., Mohan, K., and Pearl, J. (2015). Missing data
as a causal and probabilistic problem. In Proceedings
of the Thirty-First Conference on Uncertainty in Arti-
ficial Intelligence, UAI’15, pages 802–811, Arlington,
Virginia, United States. AUAI Press.
Spirtes, P., Glymour, C., and Scheines, R. (2000). Causa-
tion, Prediction, and Search. MIT press, 2nd edition.
ICEIS 2020 - 22nd International Conference on Enterprise Information Systems
122