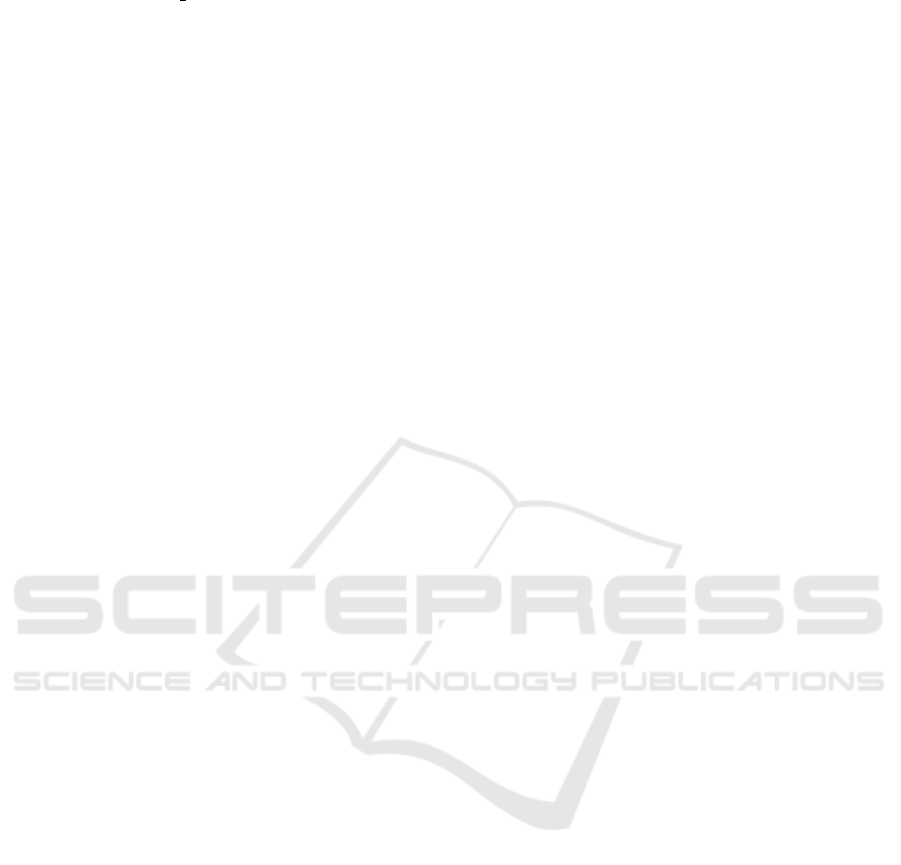
CZ.02.1.01/0.0/0.0/16 013/0001802). Access to the
CERIT-SC computing and storage facilities provided
by the CERIT-SC Center, provided under the pro-
gramme ”Projects of Large Research, Development,
and Innovations Infrastructures” (CERIT Scientific
Cloud LM2015085), is greatly appreciated.
REFERENCES
Aggarwal, C. C. (2015). Outlier analysis. In Data mining,
pages 237–263. Springer.
Ardakanian, O., Koochakzadeh, N., Singh, R. P., Golab,
L., and Keshav, S. (2014). Computing electricity con-
sumption profiles from household smart meter data. In
EDBT/ICDT Workshops, volume 14, pages 140–147.
Barker, S., Mishra, A., Irwin, D., Cecchet, E., Shenoy, P.,
Albrecht, J., et al. (2012). Smart*: An open data set
and tools for enabling research in sustainable homes.
SustKDD, August, 111(112):108.
Batra, N., Kelly, J., Parson, O., Dutta, H., Knottenbelt,
W., Rogers, A., Singh, A., and Srivastava, M. (2014).
Nilmtk: an open source toolkit for non-intrusive load
monitoring. In Proceedings of the 5th international
conference on Future energy systems, pages 265–276.
ACM.
Buzau, M. M., Tejedor-Aguilera, J., Cruz-Romero, P.,
and G
´
omez-Exp
´
osito, A. (2018). Detection of non-
technical losses using smart meter data and super-
vised learning. IEEE Transactions on Smart Grid,
10(3):2661–2670.
Caithness, N. and Wallom, D. (2018). Anomaly detection
for industrial big data. In Proceedings of the 7th In-
ternational Conference on Data Science, Technology
and Applications, DATA 2018, page 285–293, Se-
tubal, PRT. SCITEPRESS - Science and Technology
Publications, Lda.
Chandola, V., Banerjee, A., and Kumar, V. (2009).
Anomaly detection: A survey. ACM computing sur-
veys (CSUR), 41(3):15.
Chou, J.-S. and Telaga, A. S. (2014). Real-time detection of
anomalous power consumption. Renewable and Sus-
tainable Energy Reviews, 33:400–411.
Chren, S., Rossi, B., and Pitner, T. (2016). Smart grids de-
ployments within eu projects: The role of smart me-
ters. In 2016 Smart cities symposium Prague (SCSP),
pages 1–5. IEEE.
Cramer, I., Govindarajan, P., Martin, M., Savinov, A.,
Shekhawat, A., Staerk, A., and Thirugnana, A. (2018).
Detecting anomalies in device event data in the iot. In
IoTBDS, pages 52–62.
Drakontaidis, S., Stanchi, M., Glazer, G., Hussey, J., Leger,
A. S., and Matthews, S. J. (2018). Towards energy-
proportional anomaly detection in the smart grid. In
2018 IEEE High Performance extreme Computing
Conference (HPEC), pages 1–7.
Fenza, G., Gallo, M., and Loia, V. (2019). Drift-aware
methodology for anomaly detection in smart grid.
IEEE Access, 7:9645–9657.
Garc
´
ıa, J., Zamora, E., and Sossa, H. (2018). Super-
vised and unsupervised neural networks: Experimen-
tal study for anomaly detection in electrical consump-
tion. In Mexican International Conference on Artifi-
cial Intelligence, pages 98–109. Springer.
G
´
omez, O. S., Juristo, N., and Vegas, S. (2010). Replica-
tions types in experimental disciplines. In Proceed-
ings of the 2010 ACM-IEEE international symposium
on empirical software engineering and measurement,
page 3. ACM.
Jung., O., Smith., P., Magin., J., and Reuter., L. (2019).
Anomaly detection in smart grids based on software
defined networks. In Proceedings of the 8th Interna-
tional Conference on Smart Cities and Green ICT Sys-
tems - Volume 1: SMARTGREENS,, pages 157–164.
INSTICC, SciTePress.
Juristo, N. and Vegas, S. (2011). The role of non-exact repli-
cations in software engineering experiments. Empiri-
cal Software Engineering, 16(3):295–324.
Lip
ˇ
c
´
ak, P., Macak, M., and Rossi, B. (2019). Big data plat-
form for smart grids power consumption anomaly de-
tection. In 2019 Federated Conference on Computer
Science and Information Systems (FedCSIS), pages
771–780.
Liu, X., Iftikhar, N., Nielsen, P. S., and Heller, A. (2016).
Online anomaly energy consumption detection using
lambda architecture. In International Conference on
Big Data Analytics and Knowledge Discovery, pages
193–209. Springer.
Liu, X. and Nielsen, P. S. (2016). Regression-based online
anomaly detection for smart grid data. arXiv preprint
arXiv:1606.05781.
R Core Team (2018). R: A Language and Environment for
Statistical Computing. R Foundation for Statistical
Computing, Vienna, Austria.
Rossi, B. and Chren, S. (2019). Smart grids data analysis:
A systematic mapping study. IEEE Transactions on
Industrial Informatics.
Rossi, B., Chren, S., Buhnova, B., and Pitner, T. (2016).
Anomaly detection in smart grid data: An experience
report. In 2016 ieee international conference on sys-
tems, man, and cybernetics (smc), pages 2313–2318.
IEEE.
Saad, A. and Sisworahardjo, N. (2017). Data analytics-
based anomaly detection in smart distribution net-
work. In 2017 International Conference on High
Voltage Engineering and Power Systems (ICHVEPS),
pages 1–5. IEEE.
Sial, A., Singh, A., Mahanti, A., and Gong, M. (2018).
Heuristics-based detection of abnormal energy con-
sumption. In International Conference on Smart Grid
Inspired Future Technologies, pages 21–31. Springer.
Zhang, Y., Chen, W., and Black, J. (2011). Anomaly de-
tection in premise energy consumption data. In 2011
IEEE Power and Energy Society General Meeting,
pages 1–8. IEEE.
A Large-scale Replication of Smart Grids Power Consumption Anomaly Detection
295