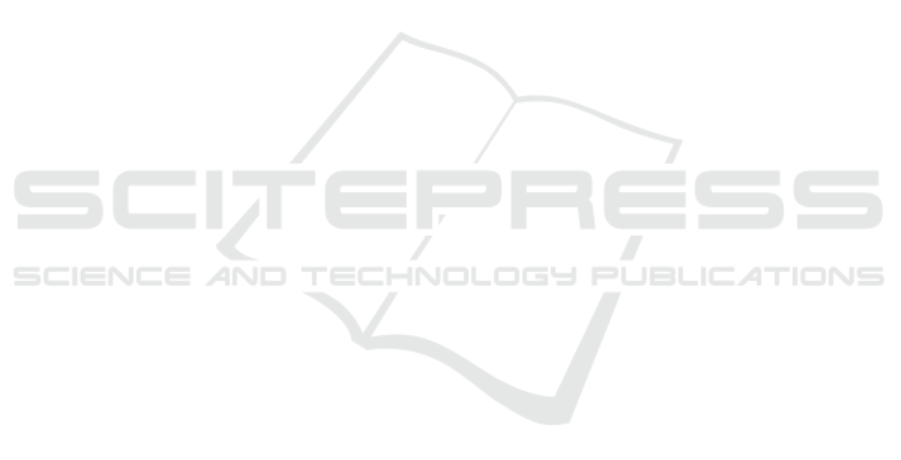
machine learning with big data in the hadoop ecosys-
tem. Journal of Big Data, 2:1–36.
Liu, X., Iftikhar, N., and Xie, X. (2014). Survey of real-time
processing systems for big data. In Proceedings of
the 18th International Database Engineering &
Applications Symposium, IDEAS ’14, pages 356–361,
New York, NY, USA. ACM.
Lopez, M. A., Lobato, A. G. P., and Duarte, O. C. M. B.
(2016). A performance comparison of open-source
stream processing platforms. In 2016 IEEE Global
Communications Conference (GLOBECOM), pages
1–6.
Lourenc¸o, J. R., Cabral, B., Carreiro, P., Vieira, M., and
Bernardino, J. (2015). Choosing the right nosql
database for the job: a quality attribute evaluation.
Journal of Big Data, 2(1):18.
Lu, J. and Thomo, A. (2016). An experimental evaluation
of giraph and graphchi. In 2016 IEEE/ACM Inter-
national Conference on Advances in Social Networks
Analysis and Mining (ASONAM), pages 993–996.
Lu, Y., Cheng, J., Yan, D., and Wu, H. (2014). Large-scale
distributed graph computing systems: An experimen-
tal evaluation. Proceedings of the VLDB Endowment,
8(3):281–292.
Makris, A., Tserpes, K., Andronikou, V., and Anagnos-
topoulos, D. (2016). A classification of nosql data
stores based on key design characteristics. Procedia
Computer Science, 97:94 – 103. 2nd International
Conference on Cloud Forward: From Distributed to
Complete Computing.
Marcu, O., Costan, A., Antoniu, G., and P
´
erez-Hern
´
andez,
M. S. (2016). Spark versus flink: Understanding per-
formance in big data analytics frameworks. In 2016
IEEE International Conference on Cluster Computing
(CLUSTER), pages 433–442.
Mazumdar, S., Seybold, D., Kritikos, K., and Verginadis,
Y. (2019). A survey on data storage and placement
methodologies for cloud-big data ecosystem. Journal
of Big Data, 6(1):15.
Oussous, A., Benjelloun, F.-Z., Lahcen, A. A., and Belfkih,
S. (2018a). Big data technologies: A survey. Journal
of King Saud University - Computer and Information
Sciences, 30(4):431 – 448.
Oussous, A., Benjelloun, F.-Z., Lahcen, A. A., and Belfkih,
S. (2018b). Big data technologies: A survey. Journal
of King Saud University - Computer and Information
Sciences, 30(4):431 – 448.
Płuciennik, E. and Zgorzałek, K. (2017). The multi-model
databases – a review. In Kozielski, S., Mrozek, D.,
Kasprowski, P., Małysiak-Mrozek, B., and Kostrzewa,
D., editors, Beyond Databases, Architectures and
Structures. Towards Efficient Solutions for Data Anal-
ysis and Knowledge Representation, pages 141–152,
Cham. Springer International Publishing.
Qian, S., Wu, G., Huang, J., and Das, T. (2016). Bench-
marking modern distributed streaming platforms. In
2016 IEEE International Conference on Industrial
Technology (ICIT), pages 592–598.
Qin, X., Chen, Y., Chen, J., Li, S., Liu, J., and Zhang,
H. (2017). The performance of sql-on-hadoop sys-
tems - an experimental study. In 2017 IEEE Inter-
national Congress on Big Data (BigData Congress),
pages 464–471.
Raghav, R. S., Pothula, S., Vengattaraman, T., and Ponnu-
rangam, D. (2016). A survey of data visualization
tools for analyzing large volume of data in big data
platform. In 2016 International Conference on Com-
munication and Electronics Systems (ICCES), pages
1–6.
Ramadan, R. (2017). Big data tools-an overview. Inter-
national Journal of Computer Science and Software
Engineering, 2.
Richter, A. N., Khoshgoftaar, T. M., Landset, S., and
Hasanin, T. (2015). A multi-dimensional comparison
of toolkits for machine learning with big data. In 2015
IEEE International Conference on Information Reuse
and Integration, pages 1–8. IEEE.
Rodrigues, M., Santos, M. Y., and Bernardino, J. (2019).
Experimental evaluation of big data analytical tools.
In Themistocleous, M. and Rupino da Cunha, P., ed-
itors, Information Systems, pages 121–127, Cham.
Springer International Publishing.
Sakr, S. (2016). Big data 2.0 processing systems: a survey.
Springer.
Samadi, Y., Zbakh, M., and Tadonki, C. (2016). Compara-
tive study between hadoop and spark based on hibench
benchmarks. In 2016 2nd International Conference
on Cloud Computing Technologies and Applications
(CloudTech), pages 267–275.
Santos, M. Y., Costa, C., Galv
˜
ao, J. a., Andrade, C., Mart-
inho, B. A., Lima, F. V., and Costa, E. (2017). Evalu-
ating sql-on-hadoop for big data warehousing on not-
so-good hardware. In Proceedings of the 21st Inter-
national Database Engineering & Applications Sym-
posium, IDEAS 2017, page 242–252, New York, NY,
USA. Association for Computing Machinery.
Shatnawi, A., Al-Bdour, G., Al-Qurran, R., and Al-Ayyoub,
M. (2018). A comparative study of open source deep
learning frameworks. In 2018 9th International Con-
ference on Information and Communication Systems
(ICICS), pages 72–77.
Siddiqa, A., Karim, A., and Gani, A. (2017). Big data stor-
age technologies: a survey. Frontiers of Information
Technology & Electronic Engineering, 18(8):1040–
1070.
Siddique, K., Akhtar, Z., Yoon, E. J., Jeong, Y., Dasgupta,
D., and Kim, Y. (2016). Apache hama: An emerging
bulk synchronous parallel computing framework for
big data applications. IEEE Access, 4:8879–8887.
Tapdiya, A. and Fabbri, D. (2017). A comparative analysis
of state-of-the-art sql-on-hadoop systems for interac-
tive analytics. In 2017 IEEE International Conference
on Big Data (Big Data), pages 1349–1356.
Turck, M. (2019). A turbulent year: The 2019 data &
ai landscape. https://mattturck.com/Data2019/. Ac-
cessed: 2019-12-20.
Ulusar, U. D., Ozcan, D. G., and Al-Turjman, F. (2020).
Open source tools for machine learning with big data
in smart cities. In Smart Cities Performability, Cogni-
tion, & Security, pages 153–168. Springer.
Veiga, J., Exp
´
osito, R. R., Pardo, X. C., Taboada, G. L.,
and Tourifio, J. (2016). Performance evaluation of
Big Data Processing Tools Navigation Diagram
311