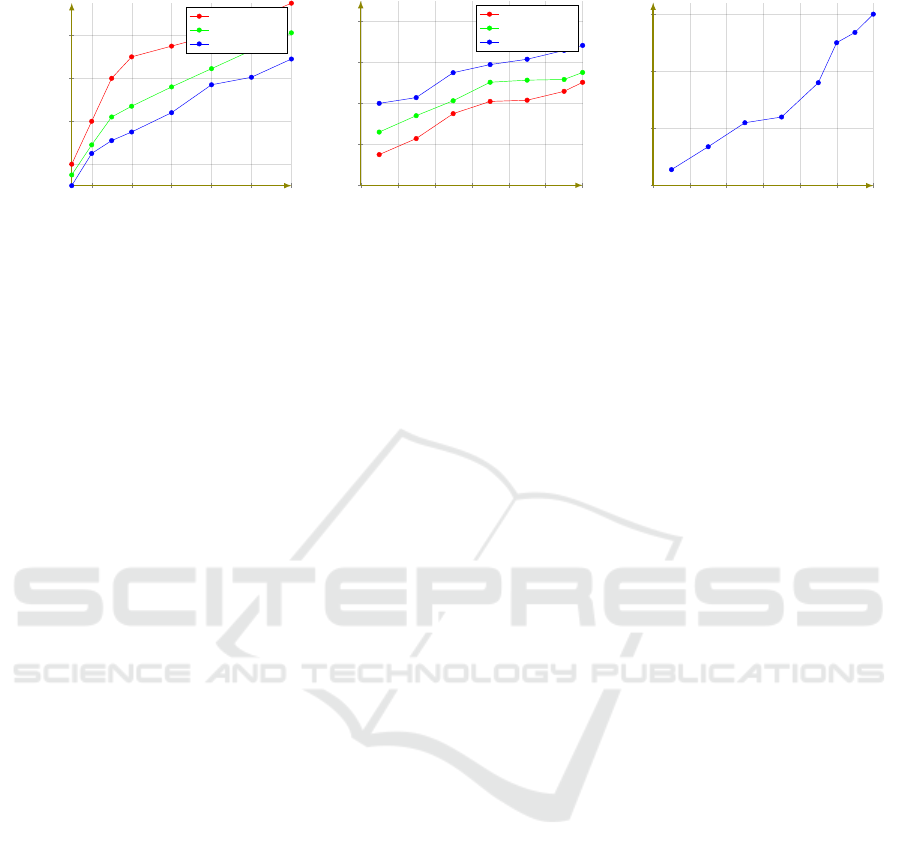
2 4
6
8 10 12
20
40
60
80
Simulation time(per Month)
Total waiting time ρ(SG)(h)
cumulative waiting time
with SGCS-RB
with FROSLR
with SGREP
(a) Total waiting time reduction.
0 2 4
6
8 10 12
20
40
60
80
100
Simulation period (per Month)
Total availability σ(SG)(%)
Total availability performance evaluation
with SGCS-RB
with FROSLR
with SGREP
(b) Total availability enhancement.
0 2 4
6
8 10 12
0
50
100
150
Simulation time(per Month)
Renewable energy (kWh)
Emergency lines performance evaluation in Tunis
(c) Emergency lines number increasing.
Figure 4: Performance Evaluation due to Reconfiguring Simulator SGREP.
ing failures recovery in SG
1
after applying different
contributions. Thus, the addition of the new num-
ber of emergency lines coming from added renewable
sources allows for increasing the recovery of failures.
Figure 4c shows the annual increase of use of renew-
able sources by integrating a new number of emer-
gency lines that allow to better enhance the recovery
of the power network. So that, the implemented sim-
ulator SGREP helps electricity markets to satisfy the
recovery and maintenance of recorded critical failures
and encourages the use of renewable sources.
Furthermore, SGREP permits to improve the per-
formed rates in term of power availability and total
waiting time thanks to implemented prediction model.
5 CONCLUSIONS
To guarantee the accurate integrated numbers of tech-
nical teams and emergency lines for best improve-
ment of failures recovery and maintenance in smart
grids, we have proposed a reconfiguration approach
that permits to predict the integrated number of ex-
pert and technician teams, besides the addition of a
new number of emergency lines which allows increas-
ing the total availability of energy during interruptions
and outages of more than 2% with the reduction of
total waiting time without energy to the half. This
approach permits to reduce economic losses during
blackouts. In the future work, we aim to secure trans-
mitted data in the smart grid proposed architecture,
beside verifying formally the proposed strategies.
REFERENCES
Abidi, M., Ben Smida, M., Khalgui, M., Li, Z., and Wu, N.
(2017). Multi agent oriented solution for forecasting
based control strategy with load priority of microgrids
in an island mode case study: Tunisian petroleum plat-
form. Electric Power Systems Research, 152:411–
423.
Ben Meskina, S., Doggaz, N., Khalgui, M., and Li, Z.
(2016). Multiagent framework for smart grids recov-
ery. IEEE SMC, 47:1284–1300.
Ben Meskina, S., Doggaz, N., Khalgui, M., and Li, Z.
(2018). Reconfiguration based methodology for im-
proving recovery performance of faults in smart grids.
Information Sciences, 454:73–95.
Bhalshankar, S. S. and Thorat, C. (2016). Integration of
smart grid with renewable energy for energy demand
management: Puducherry case study. pages 1–5.
Ding, Y., Neumann, M. A., Kehri, Ö., Ryder, G., Riedel,
T., and Beigl, M. (2014). From load forecasting to
demand response-a web of things use case. pages 28–
33.
Iqbal, M. N. and Kütt, L. (2018). End-user electricity con-
sumption modelling for power quality analysis in res-
idential building. pages 1–6.
James, J., Hou, Y., Lam, A. Y., and Li, V. O. (2017). In-
telligent fault detection scheme for microgrids with
wavelet-based deep neural networks. IEEE Transac-
tions on Smart Grid, 10(2):1694–1703.
Nefedov, E., Sierla, S., and Vyatkin, V. (2018). Internet of
energy approach for sustainable use of electric vehi-
cles as energy storage of prosumer buildings. Ener-
gies, 11:2165.
Vaidya, N. and Kasturiwale, N. (2016). Application
of quick simplex method on two phase method.
British Journal of Mathematics & Computer Science,
16(1):1–15.
Worighi, I., Maach, A., Hafid, A., Hegazy, O., and
Van Mierlo, J. (2019). Integrating renewable energy
in smart grid system. Sustainable Energy, Grids and
Networks, 18:100226.
Ziouche, L., Ben Meskina, S., Khalgui, M., and Kahloul,
L. (2019a). An optimized linear regression machine
for prediction and integration of renewable sources in
smart grid. ESM, 8(6).
Ziouche, L., Ben Meskina, S., Khalgui, M., Kahloul, L.,
and Li, Z. (2019b). Smart grid rebuilding based on
cloud computing architecture. IEEE SMC, 8(8).
Smart Grid Reconfiguration based on Prediction Model for Technical Teams Intervention Integration and Recovery Enhancement
389