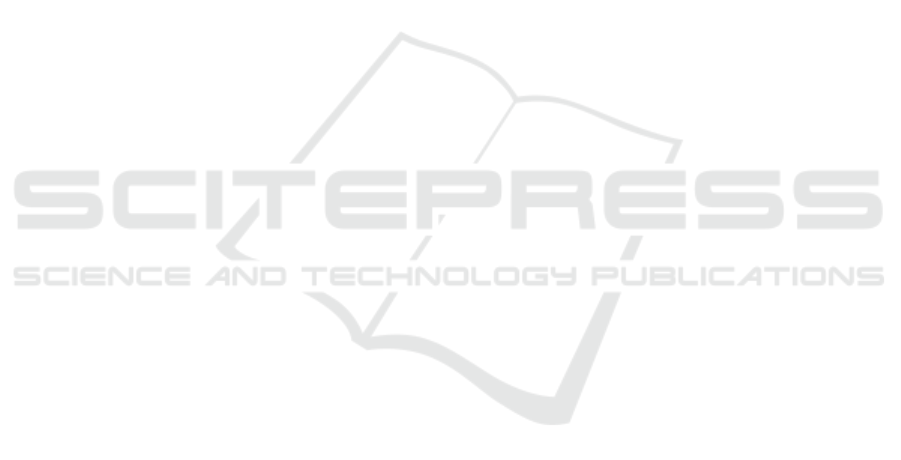
achieved at the second CRISP-DM iteration, which
involved outlier removal, while the best weft test re-
sults were obtained at the third CRISP-DM iteration,
which included the final fabric composition as an ex-
tra input feature. The best predictive results were an-
alyzed as valuable by the textile company experts. In
future work, we intend to apply a similar approach
in the prediction of other fabric quality tests, such as
pilling. Moreover, we intend to apply the best predic-
tion models in a real textile environment, aiming to
reduce the number of fabric sample creation attempts.
ACKNOWLEDGMENTS
This work was carried out within the project “Tex-
Boost: less Commodities more Specialities” ref-
erence POCI-01-0247-FEDER-024523, co-funded
by Fundo Europeu de Desenvolvimento Regional
(FEDER), through Portugal 2020 (P2020).
REFERENCES
Beltran, R., Wang, L., and Wang, X. (2006). Predicting the
pilling tendency of wool knits. The Journal of The
Textile Institute, 97(2):129–136.
Bi, J. and Bennett, K. P. (2003). Regression error char-
acteristic curves. In Proceedings of the 20th inter-
national conference on machine learning (ICML-03),
pages 43–50.
Cortez, P. (2010). Data mining with neural networks and
support vector machines using the r/rminer tool. In In-
dustrial Conference on Data Mining, pages 572–583.
Springer.
Dimitrovski, K., Gabrijel
ˇ
ci
´
c, H., Kova
ˇ
cevi
´
c, S., and
Nikoli
´
c, M. (2004). The influence of weft yarn char-
acteristics on tensile strength of woven fabrics in warp
direction. In Magic World of Textiles.
Eltayib, H. E., Ali, A. H., and Ishag, I. A. (2016). The
prediction of tear strength of plain weave fabric us-
ing linear regression models. International Journal of
Advanced Engineering Research and Science, 3(11).
Feurer, M., Klein, A., Eggensperger, K., Springenberg, J.,
Blum, M., and Hutter, F. (2015). Efficient and robust
automated machine learning. In Advances in neural
information processing systems, pages 2962–2970.
Gibert, K., Izquierdo, J., S
`
anchez-Marr
`
e, M., Hamilton,
S. H., Rodr
´
ıguez-Roda, I., and Holmes, G. (2018).
Which method to use? an assessment of data mining
methods in environmental data science. Environmen-
tal modelling & software, 110:3–27.
Gibert, K., S
`
anchez-Marr
`
e, M., and Izquierdo, J. (2016). A
survey on pre-processing techniques: Relevant issues
in the context of environmental data mining. AI Com-
munications, 29(6):627–663.
Hu, J. (2008). Fabric testing. Elsevier.
Kotb, N. (2009). Engineering of tearing strength for pile
fabrics. Journal of Textile and Apparel, Technology
and Management, 6(1).
Landry, M., Bartz, A., Aiello, S., Eckstrand, E., Fu, A., and
Aboyoun, P. (2018). Machine Learning with R and
H2O: Seventh Edition. Technical Report September.
Lasi, H., Fettke, P., Kemper, H.-G., Feld, T., and Hoffmann,
M. (2014). Industry 4.0. Business & information sys-
tems engineering, 6(4):239–242.
Le, T. T., Fu, W., and Moore, J. H. (2019). Scaling
tree-based automated machine learning to biomedical
big data with a feature set selector. Bioinformatics.
btz470.
Maher, M. M. M. Z. A. and Sakr, S. (2019). SmartML:
A Meta Learning-Based Framework for Automated
Selection and Hyperparameter Tuning for Machine
Learning Algorithms. In EDBT: 22nd International
Conference on Extending Database Technology, Lis-
bon, Portugal.
Malik, Z. A., Malik, M. H., Hussain, T., and Arain, F. A.
(2011). Development of models to predict tensile
strength of cotton woven fabrics. Journal of engi-
neered fibers and fabrics, 6(4):155892501100600407.
Mozafary, V. and Payvandy, P. (2014). Application of
data mining technique in predicting worsted spun
yarn quality. The Journal of The Textile Institute,
105(1):100–108.
Shishoo, R. (2012). The global textile and clothing indus-
try: technological advances and future challenges. El-
sevier.
Teli, M., Khare, A., and Chakrabarti, R. (2008). Depen-
dence of yarn and fabric strength on the structural pa-
rameters. AUTEX Research Journal, 8(3):63–67.
Wirth, R. and Hipp, J. (2000). Crisp-dm: Towards a stan-
dard process model for data mining. In Proceedings of
the 4th international conference on the practical ap-
plications of knowledge discovery and data mining,
pages 29–39. Citeseer.
Witten, I. H., Frank, E., Hall, M. A., and Pal, C. J. (2016).
Data Mining: Practical machine learning tools and
techniques. Morgan Kaufmann.
Yildirim, P., Birant, D., and Alpyildiz, T. (2018). Data min-
ing and machine learning in textile industry. Wiley In-
terdisciplinary Reviews: Data Mining and Knowledge
Discovery, 8(1).
Zeydan, M. (2010). Prediction of fabric tensile strength by
modelling the woven fabric. Woven Fabric Engineer-
ing, page 155.
Predicting the Tear Strength of Woven Fabrics Via Automated Machine Learning: An Application of the CRISP-DM Methodology
555