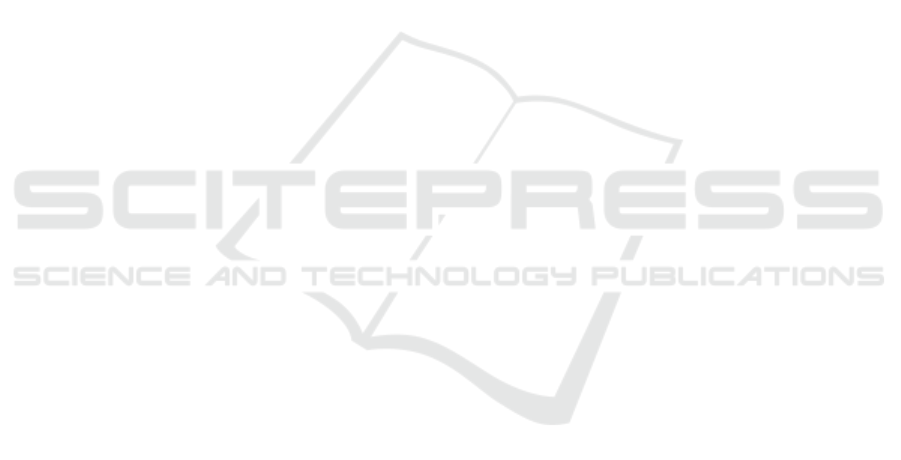
Data and facilitates dissemination and usage of Big
Data across research fields. Additionally, this work
helps to establish a common ground for all parts in-
volved in the whole Big Data technological ecosys-
tem. In this regard, knowing the Big Data taxonomy
proposed in this study may direct attention for each
required aspect. The results of this study can con-
tribute to reduce the lack of vocabulary related to Big
Data and help companies to leverage Big Data initia-
tives. Taxonomy proposed in this study may be used
to help organizations to choose technologies that best
suit their own interest as it can be used as a reference
for comparison of Big Data technologies.
This study has limits as it described Big Data
ecosystem from a technological perspective only. In
this context, no managerial, social or organizational
aspect was considered. In this regard, word ”ecosys-
tem” was used to explain only the technological as-
pects of Big Data. Thus, management processes that
oversight availability, usability, integrity and security
of data were not discussed here. Additionally, only
publications written in English had been considered.
It is important to highlight that during the ”collect
terms and concepts” stage, it was necessary to inter-
pret subjective information provided by publications
as they did not present objective details regarding the
topics analyzed. Future works could expand the pro-
posed taxonomy, creating a Big Data ontology or the-
saurus, extending this classification.
REFERENCES
1010Data (2019). Self-service platform for data manage-
ment, analytics and application building.
23andMe (2019). Dna genetic testing & analysis.
3Scan (2019). Discover in 3d with 3scan whole tissue digi-
tization and exploration.
6Sense (2019). Abm orchestration platform.
Actifio (2019). Enterprise cloud data management.
ActionIQ (2019). Enterprise customer data platform - cdp.
Active.Ai (2019). Enterprise ai platform for financial ser-
vices.
Acxiom (2019). Identity resolution & people-based market-
ing.
Aerospike (2019). Aerospike.
Affirm (2019). Affirm.
Ahlemeyer-Stubbe, A. and Coleman, S. (2014). A practical
guide to data mining for business and industry. John
Wiley & Sons.
AiCure (2019). Intelligent observation. better care.
Airobotics (2019). Automated industrial drones.
Airtable (2019). Organize anything you can imagine.
Apache (2019a). Apache drill - schema-free sql for hadoop,
nosql and cloud storage.
Apache (2019b). Apache hive tm.
Apache (2019c). Apache mesos.
Apache (2019d). Stateful computations over data streams.
Augury (2019). Machines talk, we listen.
Austerlitz, H. (2003). Data acquisition techniques using
PCs. Academic Press, San Diego, CA.
Bari, A., Chaouchi, M., and Jung, T. (2014). Predictive
Analytics for Dummies. Wiley, [s.l].
Birst (2019). Business intelligence & analytics, bi software.
Cady, F. (2017). The data science handbook. John Wiley &
Sons, Inc., Hoboken, NJ.
Chaqfeh, M. A. and Mohamed, N. (2012). Challenges in
middleware solutions for the internet of things. In
Collaboration Technologies and Systems (CTS), 2012
International Conference on, pages 21–26. IEEE.
Cignifi (2019). Cignifi transforms mobile behavior into fi-
nancial opportunity.
Cloudera (2019). The enterprise data cloud company.
CyberX (2019). Ics, scada & ot security for the industrial
network.
Darktrace (2019). Darktrace.
Demchenko, Y., de Laat, C., and Membrey, P. (2014).
Defining architecture components of the big data
ecosystem. In 2014 International Conference on Col-
laboration Technologies and Systems (CTS), pages
104–112.
Dietrich, D., Heller, B., and Yang, B. (2015). Data Science
and Big Data Analytics: Discovering, Analyzing, Vi-
sualizing and Presenting. Wiley, [s.l].
Duckett, G. (2016). Data Science: Questions and Answers.
CreateSpace Independent Publishing Platform, [s.l].
Elastic (2019). Open source log management.
Emilio, M. D. P. (2013). Data Acquisition Systems From
Fundamentals to Applied Design. Springer, New
York, NY.
Estimote (2019). Indoor location with bluetooth beacons
and mesh.
Fabbri, S., Silva, C., Hernandes, E., Octaviano, F.,
Di Thommazo, A., and Belgamo, A. (2016). Improve-
ments in the start tool to better support the systematic
review process. In Proceedings of the 20th Interna-
tional Conference on Evaluation and Assessment in
Software Engineering, pages 1–5.
Forte, R. M. (2015). Mastering predictive analytics with R:
master the craft of predictive modeling by developing
strategy, intuition, and a solid foundation in essential
concepts. Packt Publishing, Birmingham, UK.
Garcia, S., Herrera, F., and Luengo, J. (2015). Data prepro-
cessing in data mining. Springer International Pub-
lishing, Cham.
Garmin (2019). Garmin international.
Gartner (2015). Descriptive analytics.
Gass, S. I. and Harris, C. M. (2001). Encyclopedia of op-
erations research and management science. Kluwer
Academic, Boston.
Google (2019). Cloud dataflow - stream & batch data pro-
cessing — cloud dataflow — google cloud.
ICEIS 2020 - 22nd International Conference on Enterprise Information Systems
304