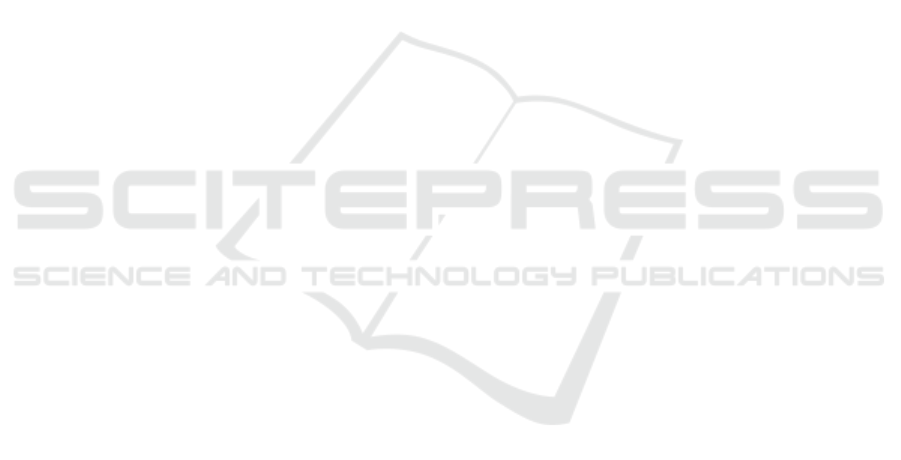
and technologies. OntoDIVE ontology, proposed in
this study, explains relationships among these terms
and concepts in the context of a data science initiative
upon big data. Proposed ontology may also be used
to confirm interdisciplinary nature of data science ini-
tiatives upon big data technologies. Another potential
usage for OntoDIVE is to make organizations capable
of drawing parallels between data science results for a
particular domain to their own domain. The ontology
may be used as a tool for comparison of both data sci-
ence frameworks and big data technologies and could
be used as basis of a framework to support organiza-
tions in their data science initiatives upon big data.
This work has several limitations. Although On-
toDIVE was designed to be a comprehensive arti-
fact capable of explaining most part of data science
initiatives upon big data, it was built based on con-
siderations from specialized professionals that work
for a single organization. Furthermore, while sev-
eral ontologies within the same domain are developed
independently by different communities, this study
was never focused on merging OntoDIVE with exist-
ing ontologies and no method was used for ontology
alignment, as proposed by (Idoudi et al., 2016). Ad-
ditionally, while OntoDIVE was validated by descrip-
tion logics queries it has not been applied in produc-
tive system. Therefore, OntoDIVE should be consid-
ered as an initial version.
Future works could create a friendly graphical
user interface to allow interaction with OntoDIVE,
since Proteg
´
e interface is not understood by many
who are not knowledgeable about ontologies and their
editing tools. Future works coud also apply Onto-
DIVE on productive systems in order to collect in-
sights and thoughts of more people and organizations
focusing on evolution of the ontology.
REFERENCES
Abdullah, M. F., Ibrahim, M., and Zulkifli, H. (2017). Re-
solving the misconceptions on big data analytics im-
plementation through government research institute in
malaysia. In International Conference on Internet of
Things, Big Data and Security, volume 2, pages 261–
266. SCITEPRESS.
Dietrich, D., Heller, B., and Yang, B. (2015). Data Science
and Big Data Analytics: Discovering, Analyzing, Vi-
sualizing and Presenting. Wiley, [s.l].
Hurwitz, J., Kaufman, M., and Bowles, A. (2015). Cogni-
tive Computing and Big Data Analytics. Wiley Pub-
lishing, [s.l], 1st edition.
Idoudi, R., Ettabaa, K. S., Hamrouni, K., and Solaiman, B.
(2016). Fuzzy clustering based approach for ontology
alignment. In ICEIS (1), pages 594–599.
Liu, J., Li, J., Li, W., and Wu, J. (2016). Rethinking big
data: A review on the data quality and usage issues.
ISPRS Journal of Photogrammetry and Remote Sens-
ing, 115:134 – 142. Theme issue ’State-of-the-art in
photogrammetry, remote sensing and spatial informa-
tion science’.
Mizoguchi, R. and Ikeda, M. (1998). Towards ontology
engineering. Journal-Japanese Society for Artificial
Intelligence, 13:9–10.
Musen, M. A. (2015). The prot
´
Eg
´
E project: A look back
and a look forward. AI Matters, 1(4):4–12.
Osterwalder, A. (2004). The business model ontology a
proposition in a design science approach. PhD the-
sis, Universit
´
e de Lausanne, Facult
´
e des hautes
´
etudes
commerciales.
Parreiras, F. S. (2011). Marrying model-driven engineering
and ontology technologies: the twouse approach.
Spyns, P., Meersman, R., and Jarrar, M. (2002). Data mod-
elling versus ontology engineering. SIGMOD Rec.,
31(4):12–17.
Staab, S. and M
¨
adche, A. (2000). Axioms are Objects, too
- Ontology Engineering beyond the Modeling of Con-
cepts and Relations. In Benjamins, V., Gomez-Perez,
A., and Guarino, N., editors, Proceedings of the Work-
shop on Applications of Ontologies and Problem-
solving Methods, 14th European Conference on Ar-
tificial Intelligence ECAI 2000, Berlin, Germany.
Staab, S., Walter, T., Gr
¨
oner, G., and Parreiras, F. S. (2010).
Model Driven Engineering with Ontology Technolo-
gies, pages 62–98. Springer Berlin Heidelberg, Berlin,
Heidelberg.
Sure, Y., Staab, S., and Studer, R. (2009). Ontology en-
gineering methodology. In Handbook on ontologies,
pages 135–152. Springer.
Vera-Baquero, A., Colomo-Palacios, R., and Molloy, O.
(2016). Real-time business activity monitoring and
analysis of process performance on big-data domains.
Telematics and Informatics, 33(3):793 – 807.
OntoDIVE: An Ontology for Representing Data Science Initiatives upon Big Data Technologies
51