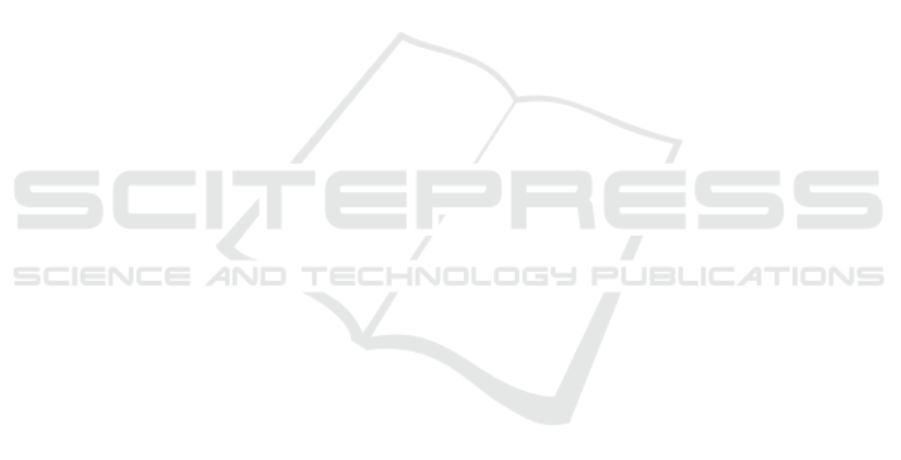
Ciolacu, M., Tehrani, A. F., Binder, L., and Svasta, P. M.
(2018). Education 4.0 - Artificial Intelligence Assisted
Higher Education: Early recognition System with Ma-
chine Learning to support Students’ Success. In 2018
IEEE 24th International Symposium for Design and
Technology in Electronic Packaging (SIITME), pages
23–30, Iasi. IEEE.
Correia, A. M. R. and Mesquita, A. (2006). Novos p
´
ublicos
no ensino superior desafios da sociedade do conheci-
mento. Edic¸
˜
oes S
´
ılabo, Lisboa. OCLC: 494458369.
Currie, E., Harvey, P. H., Daryanani, P., Augusto, J. C., Arif,
R., and Ali, A. (2016). An investigation into the effi-
cacy of avatar-based systems for student advice. EAI
Endorsed Transactions on e-Learning, 3(11):151450.
Geoffroy, F., Aimeur, E., and Gillet, D. (2002). A virtual as-
sistant for web-based training in engineering educa-
tion, volume 2363 of Lecture Notes in Computer Sci-
ence (including subseries Lecture Notes in Artificial
Intelligence and Lecture Notes in Bioinformatics).
Hanus, M. D. and Fox, J. (2015). Assessing the effects of
gamification in the classroom: A longitudinal study
on intrinsic motivation, social comparison, satisfac-
tion, effort, and academic performance. Computers
& Education, 80:152–161.
Khasianov, A., Suleymanov, D., and Marchenko, A. (2017).
Three agent platform approach for digital education
environment. pages 8580–8587, Valencia, Spain.
Laeeq, K. and Memon, Z. (2019). Scavenge: an intelligent
multi-agent based voice-enabled virtual assistant for
LMS. Interactive Learning Environments.
Lamontagne, L., Laviolette, F., Khoury, R., and Bergeron-
Guyard, A. (2014). A framework for building adap-
tive intelligent virtual assistants. Proceedings of the
IASTED International Conference on Artificial Intel-
ligence and Applications, AIA 2014, pages 342–349.
Lin, H.-C. K., Wang, C.-H., Chao, C.-J., and Chien, M.-
K. (2012). Employing Textual and Facial Emotion
Recognition to Design an Affective Tutoring System.
Turkish Online Journal of Educational Technology,
11(4):418–426. WOS:000309531600042.
Lopes, R. P., Mesquita, C., de G
´
ois, L. A., and dos San-
tos J
´
unior, G. (2019). Students’ Learning Autonomy:
A Systematic Literature Review. pages 5958–5964,
Palma, Spain.
Lopes, R. P. and Mesquita-Pires, C. (2013). Novos p
´
ublicos
no Ensino Superior Polit
´
ecnico: desafios de formac¸
˜
ao.
In Membiela, P., Casado, N., and Cebreiros, M. I.,
editors, III International Conference on Higher Ed-
ucation Teaching, pages 535–539. Educaci
´
on Editora,
Ourense, Spain.
Materla, T., Cudney, E. A., and Antony, J. (2017). The ap-
plication of Kano model in the healthcare industry: a
systematic literature review. Total Quality Manage-
ment & Business Excellence, 0(0):1–22.
Mesquita, C., Lopes, R. P., Garc
´
ıa, J. l., and de la Cruz del
R
´
ıo Rama, M. (2015). First Contact with the Word of
Work: The Competence Built in the Teaching Prac-
tices. In Peris-Ortiz, M. and Merig
´
o Lindahl, J. M.,
editors, Sustainable Learning in Higher Education SE
- 6, Innovation, Technology, and Knowledge Manage-
ment, pages 75–87. Springer International Publishing.
Mesquita, C., Lopes, R. P., Garc
´
ıa, J. l., and Rama, M. d.
l. C. d. R. (2014). Pedagogical Innovation in Higher
Education: Teachers’ Perceptions. In Peris-Ortiz, M.,
Garrig
´
os-Sim
´
on, F. J., and Gil Pechu
´
an, I., editors,
Innovation and Teaching Technologies: New Direc-
tions in Research, Practice and Policy, pages 51–60.
Springer International Publishing, Cham.
Nenkov, N., Dimitrov, G., Dyachenko, Y., and Koeva, K.
(2016). Artificial intelligence technologies for person-
nel learning management systems. pages 189–194.
Pedrazzoli, A. and dall’Acqua, L. (2009). OPUS One An
Artificial Intelligence-Multi Agent based Intelligent
Adaptive Learning Environment ( IALE ).
Pogorskiy, E., Beckmann, J., Joksimovic, S., Kovanovic,
V., and West, R. (2019). Utilising a Virtual Learning
Assistant as a Measurement and Intervention Tool for
Self-Regulation in Learning. pages 846–849.
Rodriguez, E., Burguillo, J., Rodriguez, D., Mikic Fonte,
F., Gonz
´
alez Moreno, J. C., and Novegil, J. (2008).
Developing virtual teaching assistants for open e-
learning platforms. pages 193–198.
Rosado-Pinto, P. (2008). Formac¸
˜
ao pedag
´
ogica no ensino
superior.: O caso dos docentes m
´
edicos. Revista de
Ci
ˆ
encias da Educac¸
˜
ao, (7):111–124.
Sim
˜
ao, J. V., Costa, A. d. A., and Santos, S. M. d. (2002).
Ensino superior: uma vis
˜
ao para a pr
´
oxima d
´
ecada.
Gradiva, Lisboa. OCLC: 917200006.
Subhash, S. and Cudney, E. A. (2018). Gamified learning
in higher education: A systematic review of the litera-
ture. Computers in Human Behavior, 87:192–206.
Tenorio, M., Reinaldo, F., Esperandim, R., Lopes, R., Gois,
L., and Dos Santos Junior, G. (2018). C
´
eos: A collab-
orative web-based application for improving teaching-
learning strategies. In Advances in Intelligent Systems
and Computing, volume 725, pages 107–114.
Tran, T. D., Bouchon-Meunier, B., Marsala, C., and Putois,
G.-M. (2009). An Intelligent Assistant to Support Stu-
dents and to Prevent them from Dropout. In CSEDU.
Veiga Sim
˜
ao, A. M., Flores, M. A., Fernandes, S., and
Figueira, C. (2008). Tutoria no ensino superior:
concepc¸
˜
oes e pr
´
aticas. S
´
ısifo/Revista de Ci
ˆ
encias da
Educac¸
˜
ao, (7):15.
Wong, L.-H. and Looi, C.-K. (2010). Swarm intelligence:
New techniques for adaptive systems to provide learn-
ing support. Interactive Learning Environments, 20.
Zakharova, I. (2018). Machine Learning Methods of Pro-
viding Informational Management Support for Stu-
dents’ Professional Development. The Education and
science journal, 20:91–114.
Zhang, Y., Oussena, S., Clark, T., and Kim, H. (2010). Use
Data Mining to Improve Student Retention in Higher
Education - A Case Study. In Proceedings of the
12th International Conference on Enterprise Informa-
tion Systems, pages 190–197, Funchal, Madeira, Por-
tugal. SciTePress - Science and and Technology Pub-
lications.
Virtual Assistants for Learning: A Systematic Literature Review
103