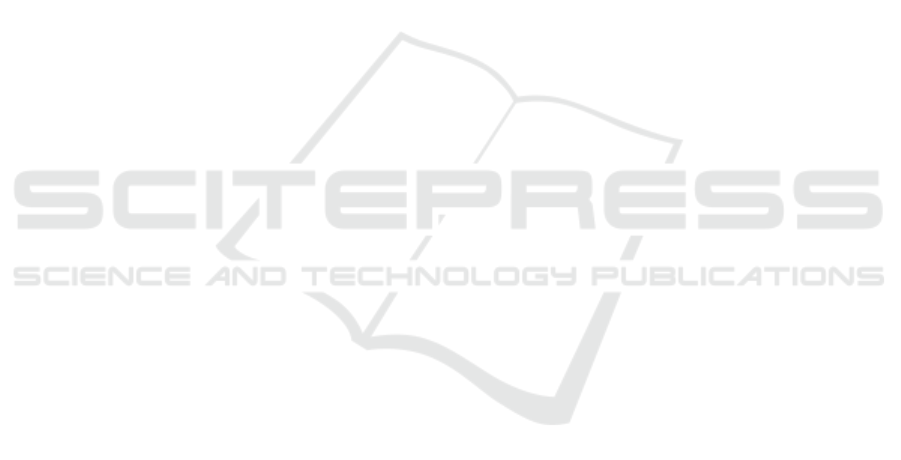
mender systems. ACM Transactions on Information
Systems (TOIS), 22(1):5–53.
Johns, J., Mahadevan, S., and Woolf, B. P. (2006). Estimat-
ing student proficiency using an item response theory
model. In Ikeda, M., Ashley, K. D., and Chan, T., ed-
itors, Intelligent Tutoring Systems, 8th International
Conference, ITS 2006, Jhongli, Taiwan, June 26-30,
2006, Proceedings, volume 4053 of Lecture Notes in
Computer Science, pages 473–480. Springer.
Lee, Y. and Cho, J. (2015). Personalized item genera-
tion method for adaptive testing systems. Multimedia
Tools Appl., 74(19):8571–8591.
Lee, Y., Cho, J., Han, S., and Choi, B. (2010). A person-
alized assessment system based on item response the-
ory. In Luo, X., Spaniol, M., Wang, L., Li, Q., Nejdl,
W., and Zhang, W., editors, Advances in Web-Based
Learning - ICWL 2010 - 9th International Conference,
Shanghai, China, December 8-10, 2010. Proceedings,
volume 6483 of Lecture Notes in Computer Science,
pages 381–386. Springer.
Lieberman, D. A. (1990). Learning: Behavior and Cogni-
tion. International Student Edition. Wadsworth Pub-
lishing Company, Belmont, CA.
Mangaroska, K., Vesin, B., and Giannakos, M. N. (2019).
Cross-platform analytics: A step towards personaliza-
tion and adaptation in education. In Proceedings of
the 9th International Conference on Learning Analyt-
ics & Knowledge, LAK 2019, Tempe, AZ, USA, March
4-8, 2019, pages 71–75. ACM.
Manouselis, N., Drachsler, H., Vuorikari, R., Hummel, H.,
and Koper, R. (2011). Recommender systems in tech-
nology enhanced learning. In Recommender systems
handbook, pages 387–415. Springer.
Mayo, M. and Mitrovic, A. (2001). Optimising its be-
haviour with bayesian networks and decision theory.
International Artificial Intelligence Education Soci-
ety, 12:124–153.
Oliveira, M. G., Marques Ciarelli, P., and Oliveira, E.
(2013). Recommendation of programming activities
by multi-label classification for a formative assess-
ment of students. Expert Systems with Applications,
40(16):6641–6651.
Pazzani, M. J. and Billsus, D. (2007). Content-based rec-
ommendation systems. In Brusilovsky, P., Kobsa, A.,
and Nejdl, W., editors, The Adaptive Web, Methods
and Strategies of Web Personalization, volume 4321
of Lecture Notes in Computer Science, pages 325–
341. Springer.
Perrenoud, P. (1998). From formative evaluation to a
controlled regulation of learning processes. towards
a wider conceptual field. Assessment in Education:
Principles, Policy & Practice, 5(1):85–102.
Roijers, D. M., Jeuring, J., and Feelders, A. (2012). Prob-
ability estimation and a competence model for rule
based e-tutoring systems. In Proceedings of the 2nd
International Conference on Learning Analytics and
Knowledge, LAK ’12, page 255–258, New York, NY,
USA. Association for Computing Machinery.
Romero, C., Ventura, S., Delgado, J. A., and Bra, P. D.
(2007). Personalized links recommendation based on
data mining in adaptive educational hypermedia sys-
tems. In Duval, E., Klamma, R., and Wolpers, M., edi-
tors, Creating New Learning Experiences on a Global
Scale, Second European Conference on Technology
Enhanced Learning, EC-TEL 2007, Crete, Greece,
September 17-20, 2007, Proceedings, volume 4753 of
Lecture Notes in Computer Science, pages 292–306.
Springer.
Sen, S. and Cohen, A. S. (2019). Applications of mixture irt
models: A literature review. Measurement: Interdis-
ciplinary Research and Perspectives, 17(4):177–191.
Shishehchi, S., Banihashem, S. Y., and Zin, N. A. M.
(2010). A proposed semantic recommendation system
for e-learning: A rule and ontology based e-learning
recommendation system. In 2010 International Sym-
posium on Information Technology, volume 1, pages
1–5.
Sinharay, S., Johnson, M. S., and Stern, H. S. (2006).
Posterior predictive assessment of item response the-
ory models. Applied Psychological Measurement,
30(4):298–321.
Spalenza, M. A., Nogueira, M. A., de Andrade,
L. B., and Oliveira, E. (2018). Uma Fer-
ramenta para Minerac¸
˜
ao de Dados Educacionais:
Extrac¸
˜
ao de Informac¸
˜
ao em Ambientes Virtuais
de Aprendizagem. In Anais do Computer on
the Beach, pages 741–750, Florian
´
opolis, Brazil.
https://computeronthebeach.com.br/.
Timms, M. J. (2007). Using item response theory (IRT)
to select hints in an ITS. In Luckin, R., Koedinger,
K. R., and Greer, J. E., editors, Artificial Intelli-
gence in Education, Building Technology Rich Learn-
ing Contexts That Work, Proceedings of the 13th Inter-
national Conference on Artificial Intelligence in Ed-
ucation, AIED 2007, July 9-13, 2007, Los Angeles,
California, USA, volume 158 of Frontiers in Artificial
Intelligence and Applications, pages 213–221. IOS
Press.
Traub, R. E. (1997). Classical test theory in historical per-
spective. Educational Measurement, 16:8–13.
van der Linden, W. J. and Hambleton, R. K. (2013). Hand-
book of modern item response theory. Springer Sci-
ence & Business Media.
Wauters, K., Desmet, P., and Van Den Noortgate, W. (2010).
Adaptive item-based learning environments based on
the item response theory: possibilities and challenges.
Journal of Computer Assisted Learning, 26(6):549–
562.
Yeung, C. (2019). Deep-irt: Make deep learning based
knowledge tracing explainable using item response
theory. In Desmarais, M. C., Lynch, C. F., Merceron,
A., and Nkambou, R., editors, Proceedings of the 12th
International Conference on Educational Data Min-
ing, EDM’19, Montr
´
eal, Canada, July 2-5, 2019. In-
ternational Educational Data Mining Society.
CSEDU 2020 - 12th International Conference on Computer Supported Education
268