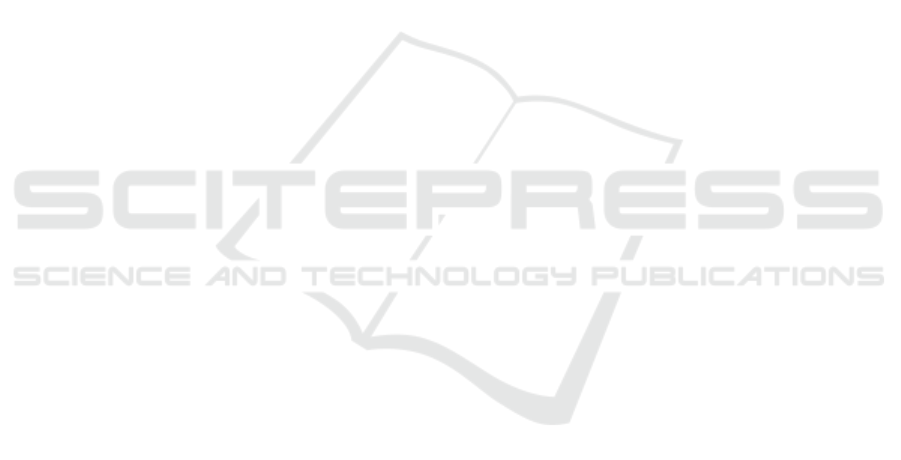
especially with respect to the incoming demands, as
well as the effect of different time steps for perform-
ing repositioning decisions.
ACKNOWLEDGEMENTS
This report and the work described were sponsored by
the U.S. Department of Energy Vehicle Technologies
Office under the Systems and Modeling for Accel-
erated Research in Transportation Mobility Labora-
tory Consortium, an initiative of the Energy Efficient
Mobility Systems Program. David Anderson, a De-
partment of Energy Office of Energy Efficiency and
Renewable Energy manager, played an important role
in establishing the project concept, advancing imple-
mentation, and providing ongoing guidance.
REFERENCES
Alonso-Mora, J., Samaranayake, S., Wallar, A., Fraz-
zoli, E., and Rus, D. (2017). On-demand high-
capacity ride-sharing via dynamic trip-vehicle assign-
ment. Proceedings of the National Academy of Sci-
ences, 114(3):462–467.
Auld, J., Hope, M., Ley, H., Sokolov, V., Xu, B., and
Zhang, K. (2016). Polaris: Agent-based modeling
framework development and implementation for inte-
grated travel demand and network and operations sim-
ulations. Transportation Research Part C: Emerging
Technologies, 64:101–116.
Auld, J. and Mohammadian, A. (2009). Framework for
the development of the agent-based dynamic activity
planning and travel scheduling (adapts) model. Trans-
portation Letters, 1(3):245–255.
Auld, J. and Mohammadian, A. K. (2012). Activity
planning processes in the agent-based dynamic ac-
tivity planning and travel scheduling (adapts) model.
Transportation Research Part A: Policy and Practice,
46(8):1386–1403.
Auld, J., Verbas, O., and Stinson, M. (2019a). Agent-based
dynamic traffic assignment with information mixing.
Procedia Computer Science, 151:864–869.
Auld, J. A., de Souza, F., Enam, A., Javanmardi, M., Stin-
son, M., Verbas, O., and Rousseau, A. (2019b). Ex-
ploring the mobility and energy implications of shared
versus private autonomous vehicles. In 2019 IEEE In-
telligent Transportation Systems Conference (ITSC),
pages 1691–1696. IEEE.
Bischoff, J. and Maciejewski, M. (2016). Simulation
of city-wide replacement of private cars with au-
tonomous taxis in berlin. Procedia computer science,
83:237–244.
Dandl, F., Hyland, M., Bogenberger, K., and Mahmas-
sani, H. S. (2019). Evaluating the impact of spatio-
temporal demand forecast aggregation on the oper-
ational performance of shared autonomous mobility
fleets. Transportation, pages 1–22.
de Souza, F., Gurumurthy, K. M., Auld, J., and Kockel-
man, K. M. (2020). A repositioning method for shared
autonomous vehicles operation. In Presented at the
9th International Workshop on Agent-based Mobil-
ity, Traffic and Transportation Models, Methodologies
and Applications (ABMTRANS), Warsaw, Poland.
de Souza, F., Verbas, O., and Auld, J. (2019). Mesoscopic
traffic flow model for agent-based simulation. Proce-
dia Computer Science, 151:858–863.
Fagnant, D. J. and Kockelman, K. (2015). Preparing a
nation for autonomous vehicles: opportunities, barri-
ers and policy recommendations. Transportation Re-
search Part A: Policy and Practice, 77:167–181.
Fagnant, D. J. and Kockelman, K. M. (2014). The travel
and environmental implications of shared autonomous
vehicles, using agent-based model scenarios. Trans-
portation Research Part C: Emerging Technologies,
40:1–13.
Gurumurthy, K. M., de Souza, F., Enam, A., and Auld, J.
(2020). Large-scale simulation of shared autonomous
vehicles: Integrating the supply and demand perspec-
tives. In Presented at the 99th Annual Meeting of
the Transportation Research Board (TRB), Washing-
ton DC.
Gurumurthy, K. M., Kockelman, K. M., and Simoni, M. D.
(2019). Benefits and costs of ride-sharing in shared
automated vehicles across austin, texas: Opportuni-
ties for congestion pricing. Transportation Research
Record, 2673(6):548–556.
Henao, A. and Marshall, W. E. (2019). The impact of ride
hailing on parking (and vice versa). Journal of Trans-
port and Land Use, 12(1).
Hyland, M. and Mahmassani, H. S. (2018). Dynamic
autonomous vehicle fleet operations: Optimization-
based strategies to assign avs to immediate traveler
demand requests. Transportation Research Part C:
Emerging Technologies, 92:278–297.
Makhorin, A. (2001). Gnu linear programming kit (glpk).
Department for Applied Informatics, Moscow Avia-
tion Institute, Moscow, Russia.
Newell, G. F. (1993). A simplified theory of kine-
matic waves in highway traffic, part i: General the-
ory. Transportation Research Part B: Methodological,
27(4):281–287.
Shladover, S. E., Su, D., and Lu, X.-Y. (2012). Impacts of
cooperative adaptive cruise control on freeway traffic
flow. Transportation Research Record, 2324(1):63–
70.
Simoni, M. D., Kockelman, K. M., Gurumurthy, K. M., and
Bischoff, J. (2019). Congestion pricing in a world of
self-driving vehicles: An analysis of different strate-
gies in alternative future scenarios. Transportation Re-
search Part C: Emerging Technologies, 98:167–185.
Spieser, K., Treleaven, K., Zhang, R., Frazzoli, E., Mor-
ton, D., and Pavone, M. (2014). Toward a systematic
approach to the design and evaluation of automated
mobility-on-demand systems: A case study in singa-
An Optimization-based Strategy for Shared Autonomous Vehicle Fleet Repositioning
375