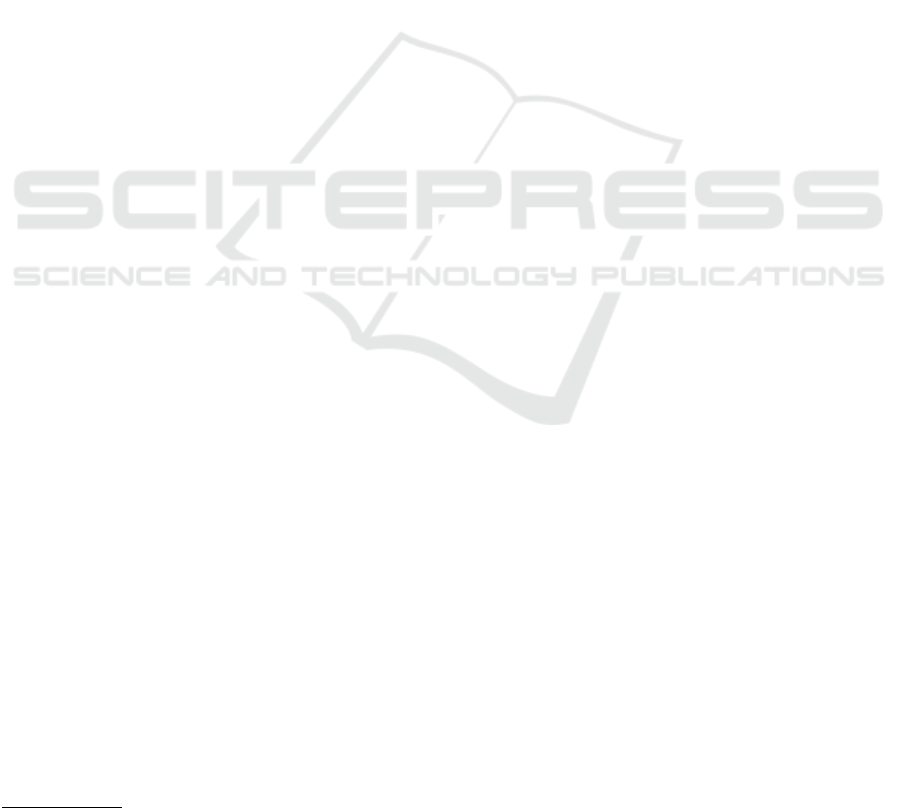
(iii) Cognitive Computing and Joint Cognitive sys-
tems to allow properly human-computer sym-
biosis;
(iv) Process automation and software/component
composition to allow the combination of tools
functionalities to automate user work;
(v) Human-Computer Interaction (HCI) which is
a research area that investigates and explores
human-computer symbiosis;
(vi) Visualization techniques and tools in order to
provide the learned knowledge to the data sci-
entist so she can properly understand what was
learned and how it was learned, and be able to
give feedback;
(vii) Recommendation systems or recommender sys-
tems which are software tools and techniques
that provide suggestions to support users’ deci-
sions (Adomavicius and Tuzhilin, 2005).
HCI and AI expertise are been combined for some
time (Grudin, 2009). The combination of HCI and
AI research is been a concerned of major tech com-
panies like Google
7
, which launched an initiative to
study and redesign the ways people interact with AI
systems (Holbrook, 2017).
As future work, we are going to further evaluate
solutions for the main challenges EW faces and im-
plement the proposed architecture considering: the
use of provenance data to improve process mining;
extracting relevant process fragments from a pool of
process instances; diminishing the usage obstacles -
in particular those related with monitoring; connect-
ing ideas of Cognitive System Engineering methods
(Elm et al., 2008)(Bonaceto and Burns, 2006) to find
points in the interaction that can be improved and how
they could be improved.
We also aiming at performing case studies to eval-
uate the tool in O&G area. The evaluation is not an
easy tasks due to the several challenges Experiment
Workbench has to deal with. We believe study case
fits this work because it is an empirical research in a
real context and there are variables in the investiga-
tive pheomenon we do not know or we do not want
to control. Evaluation in real scenario will allow ob-
servation of how data scientist engage in their tasks
using Experiment Workbench. A case study approach
suggests the use of several data sources to deep the in-
vestigation, which meet Experiment Workbench eval-
uation mainly because the disparate characteristics of
its components (Pimentel, 2011)(Yin, 2015).
7
https://ai.google/pair/
REFERENCES
Adomavicius, G. and Tuzhilin, A. (2005). Toward the
next generation of recommender systems: A sur-
vey of the state-of-the-art and possible extensions.
IEEE transactions on knowledge and data engineer-
ing, 17(6):734–749.
Bonaceto, C. and Burns, K. (2006). Using cognitive
engineering to improve systems engineering. In
Manuscript submitted for presentation at the 2006 In-
ternational Council on Systems Engineering Confer-
ence.
Davenport, T. (2005). Thinking for a living.
Davenport, T. H. and Patil, D. (2012). Data scientist. Har-
vard business review, 90:70–76.
Di Ciccio, C., Marrella, A., and Russo, A. (2012).
Knowledge-intensive processes: an overview of con-
temporary approaches. Knowledge-intensive Business
Processes, page 33.
Di Ciccio, C., Marrella, A., and Russo, A. (2015).
Knowledge-intensive processes: characteristics, re-
quirements and analysis of contemporary approaches.
Journal on Data Semantics, 4(1):29–57.
Dumas, M., Rosa, M. L., Mendling, J., and Reijers, H. A.
(2013). Fundamentals of Business Process Manage-
ment, volume 1. Springer.
Elm, W. C., Gualtieri, J. W., McKenna, B. P., Tittle, J. S.,
Peffer, J. E., Szymczak, S. S., and Grossman, J. B.
(2008). Integrating cognitive systems engineering
throughout the systems engineering process. Jour-
nal of Cognitive Engineering and Decision Making,
2(3):249–273.
Eppler, M. J., Seifried, P. M., and R
¨
opnack, A. (1999). Im-
proving knowledge intensive processes through an en-
terprise knowledge medium. In Proceedings of the
1999 ACM SIGCPR Conference on Computer Per-
sonnel Research, SIGCPR ’99, pages 222–230, New
York, NY, USA. ACM.
Fayyad, U., Piatetsky-Shapiro, G., and Smyth, P.
(1996). From data mining to knowledge discovery in
databases. AI magazine, 17(3):37.
Gaignard, A., Belhajjame, K., and Skaf-Molli, H. (2017).
SHARP: Harmonizing and bridging cross-workflow
provenance. In European Semantic Web Conference,
pages 219–234. Springer.
Gil, Y., Miles, S., Belhajjame, K., Deus, H., Garijo, D.,
Klyne, G., Missier, P., Soiland-Reyes, S., and Zednik,
S. (2013). Prov model primer. https://www.w3.org/
TR/2013/NOTE-prov-primer-20130430/.
Grudin, J. (2009). Ai and hci: Two fields divided by a com-
mon focus. Ai Magazine, 30(4):48–48.
Holbrook, J. (2017). Human-centered machine learning.
https://medium.com/google-design/human-centered-
machine-learning-a770d10562cd. Accessed in
February 14th, 2020.
Hollnagel, E. and Woods, D. D. (2005). Joint cognitive sys-
tems: Foundations of cognitive systems engineering.
CRC Press.
Kelly, J. E. (2015). Computing, cognition and the future of
knowing. Whitepaper, IBM Research, 2.
ICEIS 2020 - 22nd International Conference on Enterprise Information Systems
594