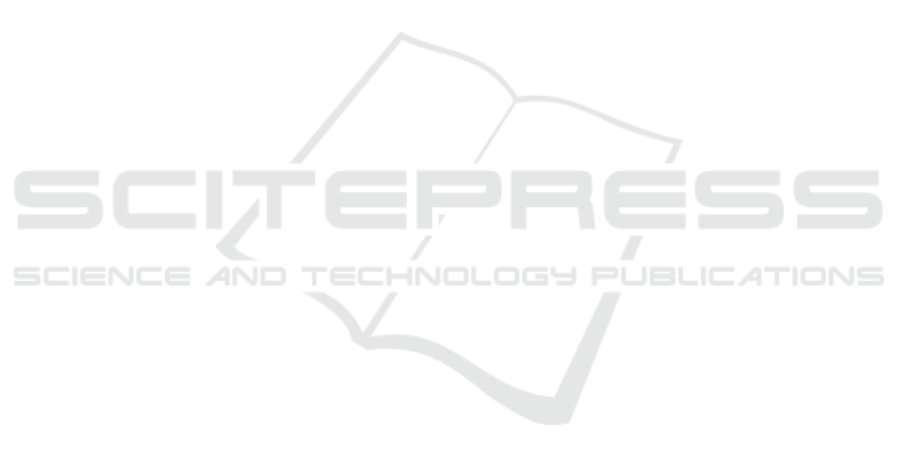
would make it possible to reach a large part of the
students on the university campus.
During this current period of the experiment, 110
students registered in the application.The inputs gen-
erated from the interactions where they were mon-
itored periodically through the evaluation question-
naire and the database. After this period the access
to the application has been restricted and the data ma-
nipulation and analysis phase has started.
For the process of evaluating the AONDE appli-
cation and its recommender system, the metrics of
accuracy of the Recommender System (RS) and user
satisfaction regarding the use of the application were
established. So that such metrics could be analyzed,
two instruments were created. The first is related to
the formalism of precision and recall used to calcu-
late the accuracy of an RS, which is combined with
a feedback system (like and dislike) inserted in each
recommended POI. While the second is a question-
naire created to collect evidence related to the usabil-
ity of the system and user satisfaction.
The analysis of the data from this experimental
mechanism is based on the calculation of precision
and recall. In order to precision and recall to be calcu-
lated in a Recommender System, the top − k items of
greatest relevance delivered by the system to the user
are used. In the context of our system, it was defined
that the 8 items with the highest recommended simi-
larity rate would represent this set. Precisely because
this is the number of POIs shown on the first page.
Therefore, the accuracy and recall were calculated for
each user who interacted with the system.
As an example, imagine that a student named Bob
accessed the system and received his recommenda-
tions after creating his profile. Bob interacts with the
system, navigating between pages, give “likes” and
“dislikes” in some of the suggested locations. This
interaction provides the necessary data to assess the
accuracy of the recommendations generated for Bob.
Suppose he liked 5 of the first 8 POIs presented, that
is, those on the first page, and that in total he liked
13 of all 86 recommended items, including those on
other pages. In this case, the precision would be
5/8 ≈ 0.63, that is, 63%, where 5 corresponds to the
number of items defined as relevant selected by the
user, and 8 the total number of items considered rele-
vant.In this case the recall would result in the propor-
tion 5/13 ≈ 0.38, that is, 38%, where 5 corresponds
to the number of items defined as relevant selected by
the user, and 13 the total number of items selected by
the user.
Applying this logic and analyzing the data col-
lected through the interactions of users with the items
recommended by AONDE, we can measure an accu-
racy of 61% and a recall equal to 66%, this having
limited to 8 the number of items considered relevant.
An accuracy of 61% represents that on average, for
every 10 items recommended, 6 met the expectations
of users, were in line with the profile and aligned with
the context. Therefore, it can be said that 61% of the
suggestions through AONDE were in fact assertive.
Thus, the probability that a selected item is the one
considered relevant for a student who interacted with
AONDE is 61%.
The RS recall was 66%, this represents the aver-
age number of times a relevant item is selected from
the total number of items selected. Thus, the greater
the recall, the greater the number of items considered
relevant to have been selected from the total. In this
context, with a 66% recall, it can be said that of all
items selected by the student during use, 66% were
among those considered relevant (top-8).
In short, the metrics collected through the data
samples point to a system with good recommendation
accuracy, considering the values of 61% for precision
and 66% for recall. The numbers indicate that in at
least more than 60% of the recommendations gener-
ated by the RS were relevant.
Of the 110 students who used AONDE applica-
tion and received recommendations, 63 students (i.e.
57%) answered the satisfaction questionnaire. Of
these students, 52.4% are male and 49.2% are fresh-
men. Students from 16 different courses participated
in the assessment (from 21 courses available in the
campus).
Regarding some usability issues from the ques-
tionnaire, they were presented in a 5-point likert scale.
Figure 3 presents the results. As Figure shows, most
students strongly agreed with the usability issues of
the app.
Regarding the question in which the student is in-
vited to say how useful for the academic journey of
a university student is to know the Points of Interest
(POI) recommended by the system, using a scale from
0 to 10, where 0 represents a lot useless and 10 very
useful, the Figure 4 presents the results. It can be seen
that the vast majority of academics agree that know-
ing such places is of great use, when voting 41.3% for
option 9 and 38.1% for option 10, totaling 50 of the
63 users who participated in the evaluation.
Students were also asked how satisfied they were
with the recommendations they received. As can be
seen in the Figure 5, the vast majority of those who
used the system and participated in the evaluation said
they were satisfied or very satisfied with the recom-
mendations received. Together these two groups rep-
resent 57 students, 90.5% of those who responded
to the evaluation. Finally, the students had the op-
POI-based Recommender System for the Support of Academics in a Smart Campus
403