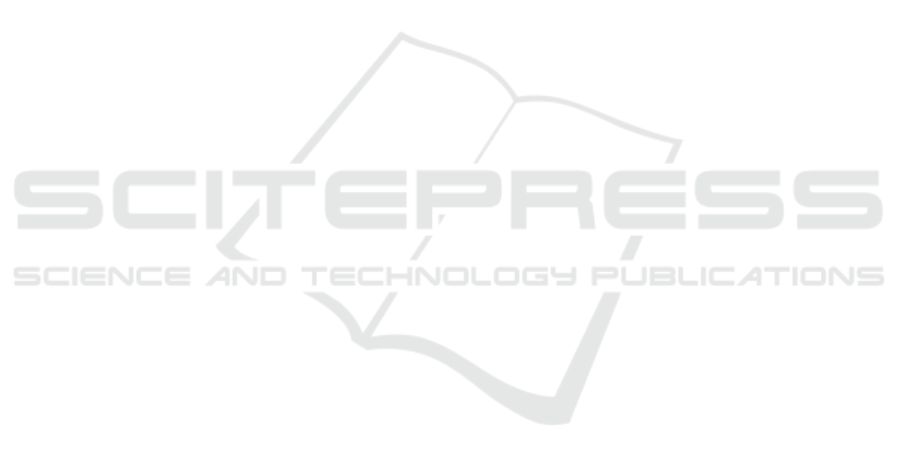
The proposed framework outperform feed forward-
based networks when the attention layer is applied.
Our future research in this context will also consider
additional architectures and data for further evalua-
tions and more general conclusions.
REFERENCES
Bahdanau, D., Cho, K., and Bengio, Y. (2014). Neural ma-
chine translation by jointly learning to align and trans-
late. arXiv preprint arXiv:1409.0473.
Bonhard, P. and Sasse, M. A. (2006). ’knowing me, know-
ing you’—using profiles and social networking to im-
prove recommender systems. BT Technology Journal,
24(3):84–98.
Chen, X., Xu, H., Zhang, Y., Tang, J., Cao, Y., Qin, Z., and
Zha, H. (2018). Sequential recommendation with user
memory networks. In Proceedings of the Eleventh
ACM International Conference on Web Search and
Data Mining, WSDM ’18, pages 108–116, New York,
NY, USA. ACM.
Dondi, R., Mauri, G., and Zoppis, I. (2016). Clique editing
to support case versus control discrimination. In Intel-
ligent Decision Technologies, pages 27–36. Springer.
Dondi, R., Mauri, G., and Zoppis, I. (2019). On the
tractability of finding disjoint clubs in a network. The-
oretical Computer Science.
Frasconi, P., Gori, M., and Sperduti, A. (1998). A general
framework for adaptive processing of data structures.
IEEE transactions on Neural Networks, 9(5):768–
786.
Gori, M., Monfardini, G., and Scarselli, F. (2005). A new
model for learning in graph domains. In Proceedings.
2005 IEEE International Joint Conference on Neural
Networks, 2005., volume 2, pages 729–734. IEEE.
Goyal, P. and Ferrara, E. (2018). Graph embedding tech-
niques, applications, and performance: A survey.
Knowledge-Based Systems, 151:78–94.
Gupta, P., Goel, A., Lin, J., Sharma, A., Wang, D., and
Zadeh, R. (2013). Wtf: The who to follow service at
twitter. In Proceedings of the 22nd international con-
ference on World Wide Web, pages 505–514. ACM.
Lee, J. B., Rossi, R. A., Kim, S., Ahmed, N. K., and Koh, E.
(2018). Attention models in graphs: A survey. arXiv
preprint arXiv:1807.07984.
Li, C., Quan, C., Peng, L., Qi, Y., Deng, Y., and Wu, L.
(2019). A capsule network for recommendation and
explaining what you like and dislike.
Lu, Y., Dong, R., and Smyth, B. (2018). Coevolutionary
recommendation model: Mutual learning between rat-
ings and reviews. In Proceedings of the 2018 World
Wide Web Conference, pages 773–782.
Scarselli, F., Gori, M., Tsoi, A. C., Hagenbuchner, M.,
and Monfardini, G. (2008). The graph neural net-
work model. IEEE Transactions on Neural Networks,
20(1):61–80.
Schafer, J. B., Frankowski, D., Herlocker, J., and Sen, S.
(2007). Collaborative filtering recommender systems.
In The adaptive web, pages 291–324. Springer.
Seo, S., Huang, J., Yang, H., and Liu, Y. (2017). Inter-
pretable convolutional neural networks with dual local
and global attention for review rating prediction. In
Proceedings of the eleventh ACM conference on rec-
ommender systems, pages 297–305.
Sperduti, A. and Starita, A. (1997). Supervised neural net-
works for the classification of structures. IEEE Trans-
actions on Neural Networks, 8(3):714–735.
Veli
ˇ
ckovi
´
c, P., Cucurull, G., Casanova, A., Romero, A., Lio,
P., and Bengio, Y. (2017). Graph attention networks.
arXiv preprint arXiv:1710.10903.
Zhang, Y. and Chen, X. (2018). Explainable recommenda-
tion: A survey and new perspectives.
Zhou, X., Xu, Y., Li, Y., Josang, A., and Cox, C. (2012).
The state-of-the-art in personalized recommender sys-
tems for social networking. Artificial Intelligence Re-
view, 37(2):119–132.
Zoppis, I., Dondi, R., Manzoni, S., Mauri, G., Marconi, L.,
and Epifania, F. (2019a). Optimized social explana-
tion for educational platforms. In Int. Conf. on Com-
puter Supported Education, volume 1, pages 85–91.
Zoppis, I., Manzoni, S., and Mauri, G. (2019b). A com-
putational model for promoting targeted communica-
tion and supplying social explainable recommenda-
tions. In 2019 IEEE 32nd International Symposium
on Computer-Based Medical Systems (CBMS), pages
429–434. IEEE.
Attentional Neural Mechanisms for Social Recommendations in Educational Platforms
117