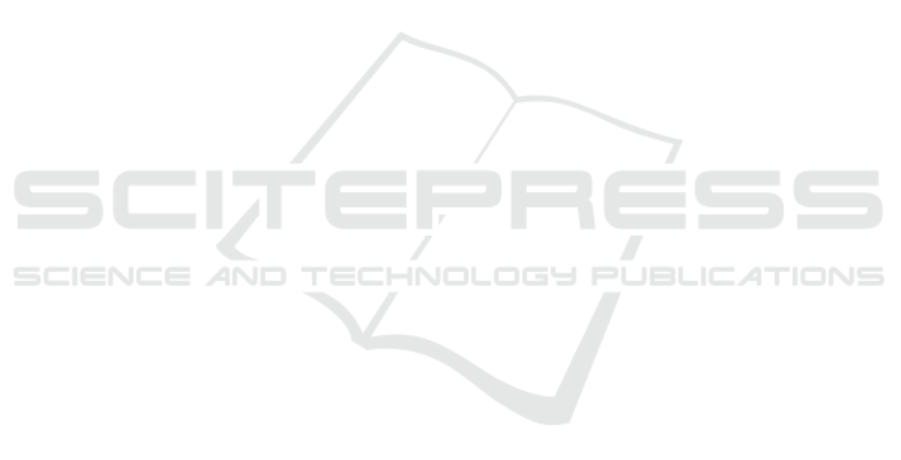
alyzed under different environment conditions: day-
time, night time and fog. This helps to identify which
specific use-cases can be transferred from the real to
the virtual domain.
Results show that complex environment condition
models, such as fog, still need to be further developed
for this kind of test, since the predictions in the virtual
scenario differs tremendously from the real one. For
daytime and night time conditions, the simulation-
generated results can be sufficient for testing pur-
poses.
The divergence in the performance results under
adverse environment conditions reinforce that algo-
rithms for automated driving systems have to be de-
veloped and tested beyond ideal conditions, such as
daytime and sunny weather. The datasets used for
training machine learning algorithms must be bal-
anced also with data acquired under non-ideal envi-
ronment conditions, so that these systems become ro-
bust enough and safety in usage is ensured.
In this work, only night time and fog in one en-
vironment simulation software were considered, but
rain and snow may also degrade the algorithms per-
formance. Therefore, further experiments can be re-
alized in these mentioned cases and with other envi-
ronment simulation software as well. In addition, this
study can be expanded to other algorithms and even
other sensors, such as radar and lidar, which are also
focus of research at the CARISSMA test center.
ACKNOWLEDGEMENTS
We applied the SDC approach for the sequence of au-
thors. This work is supported under the Ingenieur-
Nachwuchs program of the German Federal Ministry
of Education and Research (BMBF) under Grant No.
13FH578IX6.
REFERENCES
Bock, T., Maurer, M., and Farber, G. (2007). Validation
of the vehicle in the loop (vil); a milestone for the
simulation of driver assistance systems. In 2007 IEEE
Intelligent Vehicles Symposium, pages 612–617.
Corso, A., Du, P., Driggs-Campbell, K., and Kochenderfer,
M. (2019). Adaptive stress testing with reward aug-
mentation for autonomous vehicle validation.
Demers, S., Gopalakrishnan, P., and Kant, L. (2007). A
generic solution to software-in-the-loop. In MILCOM
2007 - IEEE Military Communications Conference,
pages 1–6.
Isermann, R., Schaffnit, J., and Sinsel, S. (1999). Hardware-
in-the-loop simulation for the design and testing of
engine-control systems. Control Engineering Prac-
tice, 7(5):643 – 653.
Kubota, S., Nakano, T., and Okamoto, Y. (2007). A global
optimization algorithm for real-time on-board stereo
obstacle detection systems. In 2007 IEEE Intelligent
Vehicles Symposium, pages 7–12.
Maurer, M., Gerdes, J., Lenz, B., and Winner, H. (2016).
Autonomous Driving. Technical, Legal and Social As-
pects.
Reway, F., Huber, W., and Ribeiro, E. P. (2018). Test
methodology for vision-based adas algorithms with
an automotive camera-in-the-loop. In 2018 IEEE In-
ternational Conference on Vehicular Electronics and
Safety (ICVES), pages 1–7.
Richter, S. R., Vineet, V., Roth, S., and Koltun, V. (2016).
Playing for data: Ground truth from computer games.
In Leibe, B., Matas, J., Sebe, N., and Welling, M.,
editors, Computer Vision – ECCV 2016, pages 102–
118, Cham. Springer International Publishing.
Saleem, N. H. and Klette, R. (2016). Accuracy of free-
space detection: Monocular versus binocular vision.
In 2016 International Conference on Image and Vision
Computing New Zealand (IVCNZ), pages 1–6.
Sun, Z., Bebis, G., and Miller, R. (2006). On-road vehicle
detection: a review. IEEE Transactions on Pattern
Analysis and Machine Intelligence, 28(5):694–711.
Tuncali, C. E., Fainekos, G., Prokhorov, D. V., Ito, H., and
Kapinski, J. (2019). Requirements-driven test gener-
ation for autonomous vehicles with machine learning
components. ArXiv, abs/1908.01094.
Tzomakas, C. and von Seelen, W. (1998). Vehicle de-
tection in traffic scenes using shadows. Techni-
cal report, IR-INI, INSTITUT FUR NUEROINFOR-
MATIK, RUHR-UNIVERSITAT.
Wedel, A., Badino, H., Rabe, C., Loose, H., Franke, U., and
Cremers, D. (2010). B-spline modeling of road sur-
faces with an application to free-space estimation. In-
telligent Transportation Systems, IEEE Transactions
on, 10:572 – 583.
Winner, H., Hakuli, S., Lotz, F., and Singer, C. (2015).
Handbook of driver assistance systems: Basic infor-
mation, components and systems for active safety and
comfort.
Wissing, C., Nattermann, T., Glander, K., Seewald, A., and
Bertram, T. (2016). Environment simulation for the
development, evaluation and verification of underly-
ing algorithms for automated driving. In AmE 2016 -
Automotive meets Electronics; 7th GMM-Symposium,
pages 1–6.
Yao, J., Ramalingam, S., Taguchi, Y., Miki, Y., and Urtasun,
R. (2015). Estimating drivable collision-free space
from monocular video. In 2015 IEEE Winter Confer-
ence on Applications of Computer Vision, pages 420–
427.
VEHITS 2020 - 6th International Conference on Vehicle Technology and Intelligent Transport Systems
558