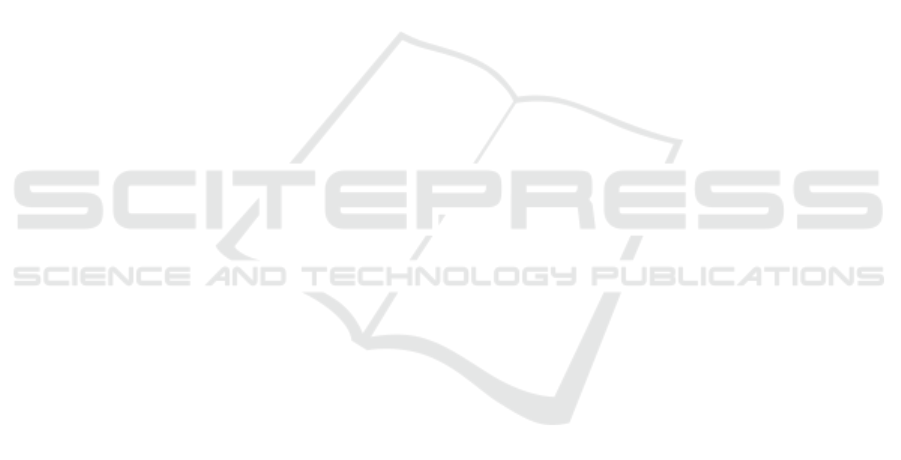
benefit companies who are offering courses to assist
high school education and companies that are par-
tially working on e-learning projects.
Moreover, there might be an economic impact on
companies that offer their employees internal training
and educational growth or learning paths, as they will
be able to provide more personalised trainings and
seminars that will reduce the lost time for overqual-
ified employees, save money for trainings within or-
ganisations, and easily and quickly verify a candi-
date’s knowledge level for a specific position.
Future educators will be able to provide spe-
cialised individual support to learners, which will in-
crease their productivity and satisfaction. On the
same page, future counsellors will be able to modify
curricula and educational systems in an easy, fast and,
most importantly, accurate way to adapt the needs of
a challenging and constantly improving the academic
and professional environment. Last but not least, stu-
dents will enjoy personalised assistance that identi-
fies their weak points and potential knowledge gaps,
and get to know where they need help in order to
reach their educational goals. Of course, they will
also learn about their strong points, which will further
push them towards pursuing their talents.
4 CONCLUSIONS
We have presented our vision of a smart learning tool
that might support students as well as teachers in their
daily life. The smart learning tool is going to be based
on a knowledge graph which connects the different
knowledge topics and also enables the tracking of
each student’s learning trajectory, making it easier to
identify a student’s knowledge gaps and plan the next
steps for a successful learning trajectory. Further, the
proposed approach helps in detecting good practices
in learning trajectories (learning paths) and method-
ologies, such as which topic should be taught first and
based on which methodology. On the practical level,
the smart learning application foresees a large pool
of assessments, which are categorised by the knowl-
edge they require in order to be addressed. This aids
learners to have a more accurate recommendation of
assessments based on their needs and profile. There-
fore, the proposed technology-enhanced smart learn-
ing environment combining knowledge graphs and
learning paths is also of great help for teachers, as it
makes the detection of a student’s knowledge gaps a
more efficient and accurate process and provides them
technology-based assistance for the personalised rec-
ommendation of assessments.
REFERENCES
Cai, J. and Nie, B. (2007). Problem Solving in Chinese
Mathematics Education: Research and Practice. ZDM
Mathematics Education, 39.
Chen, P., Lu, Y., Zheng, V. W., Chen, X., and Li, X. (2018a).
An Automatic Knowledge Graph Construction Sys-
tem for K-12 Education. In Proceedings of L@S 2018,
Annual ACM Conference on Learning at Scale, Lon-
don, UK.
Chen, P., Lu, Y., Zheng, V. W., Chen, X., and Yang, B.
(2018b). KnowEdu: A System to Construct Knowl-
edge Graph for Education. IEEE Access, 6.
Council, N. R. (2000). How People Learn. National
Academies Press.
Doignon, J.-P. and Falmagne, J.-C. (1999). Knowledge
Spaces. Springer Verlag.
Harjula, M. (2008). Mathematics Exercise System with Au-
tomatic Assessment. Master’s thesis, Helsinki Univer-
sity of Technology.
Ilkou, E. (2019). EduKnow: A Framework for Structuring
Educational Material. Master’s thesis, Vrije Univer-
siteit Brussel.
Le, N.-T. and Pinkwart, N. (2014). Towards a Classification
for Programming Exercises. In Proceedings of the 2nd
Workshop on AI-supported Education for Computer
Science, Honolulu, USA.
Lecailliez, L., Flanagan, B., and Ogata, H. (2019). Con-
struction of a Knowledge Map-based System for Per-
sonalized Second Language Learning. IPSJ SIG Tech-
nical Report, 2019-CLE-27(3).
Levav-Waynberg, A. and Leikin, R. (2006). Solving Prob-
lems in Different Ways: Teachers’ Knowledge Situ-
ated in Practice. In Proceedings of the International
Group for the Psychology of Mathematics Education,
Prague, Czech Republic.
Linn, R. L., Baker, E. L., and Dunbar, S. B. (1991).
Complex, Performance-based Assessment: Expecta-
tions and Validation Criteria. Educational Researcher,
20(8).
Rizun, M. (2019). Knowledge Graph Application in Educa-
tion: A Literature Review. Acta Universitatis Lodzien-
sis. Folia Oeconomica, 3(342).
Signer, B. and Norrie, M. C. (2007). As We May Link:
A General Metamodel for Hypermedia Systems. In
Proceedings of ER 2007, International Conference on
Conceptual Modeling, Auckland, New Zealand.
South, S. J., Haynie, D. L., and Bose, S. (2007). Student
Mobility and School Dropout. Social Science Re-
search, 36(1).
Stapel, M., Zheng, Z., and Pinkwart, N. (2016). An Ensem-
ble Method to Predict Student Performance in an On-
line Math Learning Environment. International Edu-
cational Data Mining Society.
ˇ
Zeljko
ˇ
Sundov and Gregori
´
c, M. (2014). How to Maximize
and Measure Learning’s Contribution to Business Re-
sults. Journal of Education for Entrepreneurship, 4.
CSEDU 2020 - 12th International Conference on Computer Supported Education
468