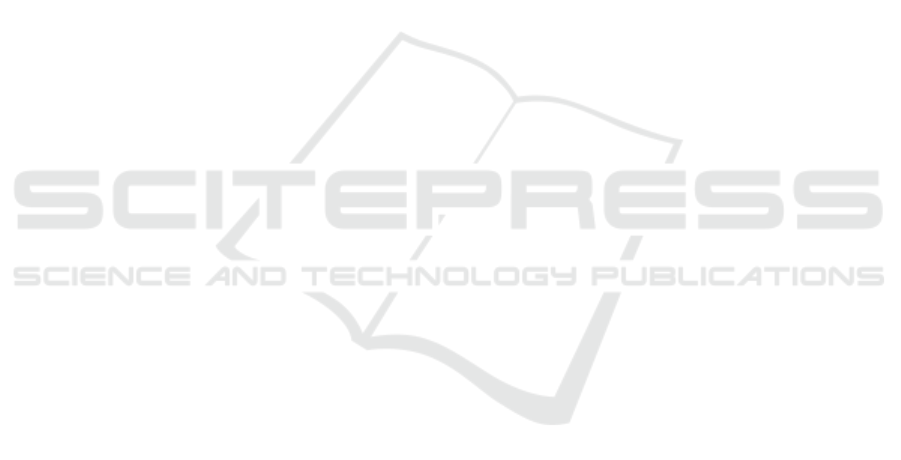
sidering absolute values is too specific because the
consumption of energy on one day may not be exactly
the same as that on the next day. Hence assigning
symbols based on absolute values at a given time may
not help infer anything useful about the data. In a time
period, if the average data consumption is considered
then it gives the approximate consumption level of the
appliance.
Overlapping window can be considered if it is
needed for the application under consideration. We
slide the window over the time duration with some
predetermined time overlapping in two consecutive
windows. This would help in maintaining the infor-
mation related to continuity of the data to some extent
depending on how much the overlap is.
Fitting Temporal Motifs Into Smart Grid
Hierarchy
We propose this model for residential type of local-
ity. This is easily scalable to other types of localities
as well, such as industrial, commercial etc and can be
extended to fit other hierarchical levels in the smart
grid as well as other complex networks. To study the
role that these motifs play in smart grid network, it
is essential that we consider the hierarchy of the par-
ticipants of the network. The hierarchy described in
(Rech and Harth, 2012) is discussed below to suit for
the requirement of our model.
• The very basic level in the hierarchy consists of
the appliances in a household. As discussed ear-
lier the motifs which are formed at this layer are
based on electricity consumption of each of the
appliances at various times.
• The layer above the layer of appliances is of
houses in a locality or a community residing at a
particular location. The motifs would consist of
the houses in the locality and the point of sup-
ply of electricity to all these houses as nodes.
The consumption of each of the houses at various
times would determine the edges and the direction
of the edges. These motifs can be defined in sim-
ilar fashion as we did earlier by determining the
suitable parameter values.
• Similarly, the next layer consists of communities
which together form a city.
• More layers can be thought of and considered on
top of the previously mentioned layers so as to
build the model of motifs that will help enable us
to study and determine various aspects of a smart
grid network.
7 CONCLUSION
Temporal motifs play an important role in characteriz-
ing networks. The change in usage of power over time
helps to study the behaviour of the consumers. We
have formally defined the Temporal Motifs in Smart
Grid network. We have also described the construc-
tion of such Temporal Motifs in Smart Grid network,
without overlapping windows, how to fit them in the
hierarchical structure of the network. In future we
will use motifs to draw inferences about participants
of the grid network. We will also look at impact of
this model on the energy distribution policies.
ACKNOWLEDGEMENTS
This research was partially supported by
NRDMS/UG/S.Mishra/Odisha/E-01/2018
REFERENCES
Aggarwal, A., Kunta, S., and Verma, P. K. (2010). A
proposed communications infrastructure for the smart
grid. In 2010 Innovative Smart Grid Technologies
(ISGT), pages 1–5, Gaithersburg, MD, USA. IEEE.
Dataport. https://dataport.pecanstreet.org/. Pecan Street
Inc. Dataport, accessed on 24 January 2020.
Funde, N., Dhabu, M., and Balande, U. (2018). Motif-
Based Pattern Detection Method for Smart Energy
Meter Data. In 2018 3rd International Conference for
Convergence in Technology (I2CT), pages 1–5, Pune.
IEEE.
Lin, J., Keogh, E., Lonardi, S., and Chiu, B. (2003). A sym-
bolic representation of time series, with implications
for streaming algorithms. In Proceedings of the 8th
ACM SIGMOD Workshop on Research Issues in Data
Mining and Knowledge Discovery, DMKD ’03, page
2–11, New York, NY, USA. Association for Comput-
ing Machinery.
Milo, R., Shen-Orr, S., Itzkovitz, S., Kashtan, N.,
Chklovskii, D., and Alon, U. (2002). Network Mo-
tifs: Simple Building Blocks of Complex Networks.
Science, 298(5594):824–827.
Mishra, S., Li, X., Pan, T., Kuhnle, A., Thai, M. T., and Seo,
J. (2016). Price modification attack and protection
scheme in smart grid. IEEE Transactions on Smart
Grid, 8(4):1864–1875.
Paranjape, A., Benson, A. R., and Leskovec, J. (2017). Mo-
tifs in temporal networks. Proceedings of the Tenth
ACM International Conference on Web Search and
Data Mining - WSDM ’17.
Rech, D. and Harth, A. (2012). Towards a decentralised hi-
erarchical architecture for smart grids. In Proceedings
of the 2012 Joint EDBT/ICDT Workshops on - EDBT-
ICDT ’12, page 111, Berlin, Germany. ACM Press.
Temporal Motifs in Smart Grid
127