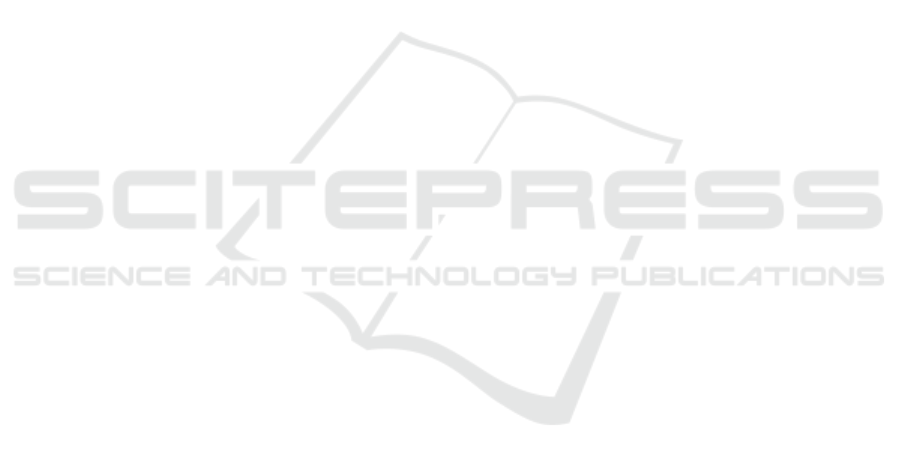
Hermoso, R., Ilarri, S., and Trillo-Lado, R. (2019). Re-
CoSKQ: Towards POIs recommendation using collec-
tive spatial keyword queries. In ACM RecSys Work-
shop on Recommenders in Tourism (RecTour 2019),
in conjunction with the 13th ACM Conference on Rec-
ommender Systems (RecSys 2019), Copenhagen (Den-
mark), volume 2435, pages 42–45. CEUR Workshop
Proceedings, ISSN 1613-0073.
Ho, S.-S., Lieberman, M., Wang, P., and Samet, H. (2012).
Mining future spatiotemporal events and their senti-
ment from online news articles for location-aware rec-
ommendation system. In First ACM SIGSPATIAL In-
ternational Workshop on Mobile Geographic Infor-
mation Systems (MobiGIS 2012), pages 25–32, New
York, NY, USA. ACM.
Jakob, N., Weber, S. H., M
¨
uller, M. C., and Gurevych, I.
(2009). Beyond the stars: Exploiting free-text user
reviews to improve the accuracy of movie recom-
mendations. In First International CIKM Workshop
on Topic-Sentiment Analysis for Mass Opinion (TSA
2009), pages 57–64, New York, NY, USA. ACM.
Kim, E.-G. and Chun, S.-H. (2019). Analyzing online car
reviews using text mining. Sustainability, 11(6):1611.
Krestel, R., Fankhauser, P., and Nejdl, W. (2009). Latent
Dirichlet Allocation for tag recommendation. In Third
ACM Conference on Recommender Systems (RecSys
2009), pages 61–68. ACM.
Li, Y., Nie, J., Zhang, Y., Wang, B., Yan, B., and Weng,
F. (2010). Contextual recommendation based on text
mining. In 23rd International Conference on Compu-
tational Linguistics (COLING 2010): Posters, pages
692–700. Association for Computational Linguistics.
Lin, J., Sugiyama, K., Kan, M.-Y., and Chua, T.-S. (2014).
New and improved: Modeling versions to improve
app recommendation. In 37th International ACM SI-
GIR Conference on Research & Development in Infor-
mation Retrieval (SIGIR 2014), pages 647–656, New
York, NY, USA. ACM.
Liu, B. and Zhang, L. (2012). A Survey of Opinion Mining
and Sentiment Analysis, pages 415–463. Springer US,
Boston, MA.
Loh, S., Lorenzi, F., Salda
˜
na, R., and Licthnow, D. (2003).
A tourism recommender system based on collabora-
tion and text analysis. Information Technology &
Tourism, 6(3):157–165.
Miao, D. and Lang, F. (2017). A recommendation system
based on text mining. In 2017 International Confer-
ence on Cyber-Enabled Distributed Computing and
Knowledge Discovery (CyberC), pages 318–321.
Mizzaro, S., Pavan, M., Scagnetto, I., and Zanello, I.
(2014). A context-aware retrieval system for mo-
bile applications. In 4th Workshop on Context-
Awareness in Retrieval and Recommendation (CARR
2014), pages 18–25. ACM.
Musto, C., Lops, P., de Gemmis, M., and Semeraro, G.
(2019). Justifying recommendations through aspect-
based sentiment analysis of users reviews. In 27th
ACM Conference on User Modeling, Adaptation and
Personalization, UMAP ’19, pages 4–12, New York,
NY, USA. ACM.
Musto, C., Narducci, F., Lops, P., De Gemmis, M., and Se-
meraro, G. (2016). ExpLOD: A framework for ex-
plaining recommendations based on the Linked Open
Data Cloud. In 10th ACM Conference on Rec-
ommender Systems (RecSys 2016), pages 151–154.
ACM.
Piao, G. and Breslin, J. G. (2018). Inferring user interests in
microblogging social networks: a survey. User Mod-
eling and User-Adapted Interaction, 28(3):277–329.
Ricci, F., Rokach, L., and Shapira, B. (2011). Recommender
Systems Handbook, chapter Introduction to Recom-
mender Systems Handbook, pages 1–35. Springer US,
Boston, MA.
Ricci, F., Rokach, L., and Shapira, B. (2015). Recommender
Systems Handbook, chapter Recommender Systems:
Introduction and Challenges, pages 1–34. Springer
US, Boston, MA.
Rosa, R. L., Schwartz, G. M., Ruggiero, W. V., and
Rodr
´
ıguez, D. Z. (2019). A knowledge-based rec-
ommendation system that includes sentiment analysis
and deep learning. IEEE Transactions on Industrial
Informatics, 15(4):2124–2135.
Roul, R. K. and Arora, K. (2019). A nifty review to
text summarization-based recommendation system for
electronic products. Soft Computing, 23(24):13183–
13204.
Shi, Y., Larson, M., and Hanjalic, A. (2014). Collaborative
filtering beyond the user-item matrix: A survey of the
state of the art and future challenges. ACM Computing
Surveys, 47(1).
Subramaniyaswamy, V., Manogaran, G., Logesh, R., Vi-
jayakumar, V., Chilamkurti, N., Malathi, D., and
Senthilselvan, N. (2019). An ontology-driven person-
alized food recommendation in IoT-based healthcare
system. The Journal of Supercomputing, 75(6):3184–
3216.
Tarus, J. K., Niu, Z., and Mustafa, G. (2018). Knowledge-
based recommendation: a review of ontology-based
recommender systems for e-learning. Artificial intel-
ligence review, 50(1):21–48.
Xu, Z., Ru, L., Xiang, L., and Yang, Q. (2011). Discov-
ering user interest on Twitter with a modified author-
topic model. In 2011 IEEE/WIC/ACM International
Conferences on Web Intelligence and Intelligent Agent
Technology, volume 1, pages 422–429.
Zarrinkalam, F., Fani, H., Bagheri, E., Kahani, M., and Du,
W. (2015). Semantics-enabled user interest detection
from twitter. In 2015 IEEE/WIC/ACM International
Conference on Web Intelligence and Intelligent Agent
Technology (WI-IAT), volume 1, pages 469–476.
Use of Text Mining Techniques for Recommender Systems
787