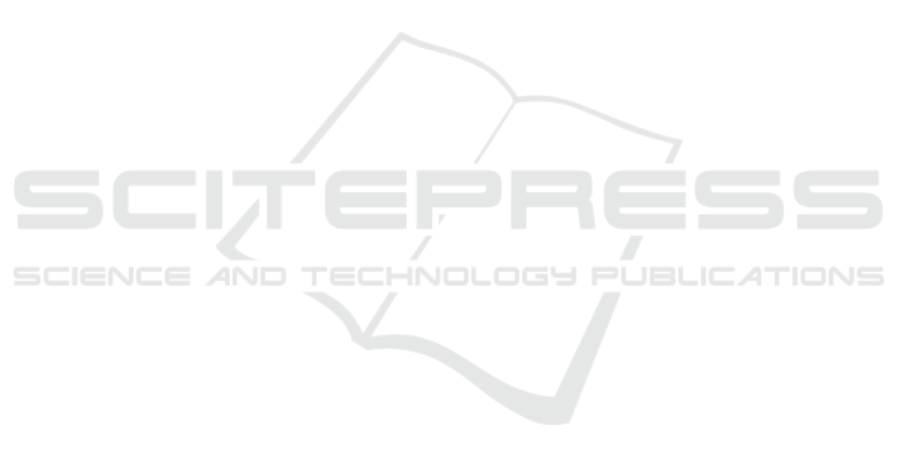
ACKNOWLEDGEMENTS
This work has been supported by the General-
itat Valenciana and the FSE through the grant
ACIF/2018/224, by the Spanish government through
the project DPI 2016-78361-R (AEI/FEDER, UE):
“Creaci
´
on de mapas mediante m
´
etodos de apariencia
visual para la navegaci
´
on de robots.” and by General-
itat Valenciana through the project AICO/2019/031:
“Creaci
´
on de modelos jer
´
arquicos y localizaci
´
on ro-
busta de robots m
´
oviles en entornos sociales”
REFERENCES
Amor
´
os, F., Pay
´
a, L., Mar
´
ın, J. M., and Reinoso, O. (2018).
Trajectory estimation and optimization through loop
closure detection, using omnidirectional imaging and
global-appearance descriptors. Expert Systems with
Applications, 102:273–290.
Bay, H., Ess, A., Tuytelaars, T., and Van Gool, L. (2008).
Speeded-up robust features (surf). Computer vision
and image understanding, 110(3):346–359.
Berenguer, Y., Pay
´
a, L., Valiente, D., Peidr
´
o, A., and
Reinoso, O. (2019). Relative altitude estimation us-
ing omnidirectional imaging and holistic descriptors.
Remote Sensing, 11(3):323.
Cebollada, S., Pay
´
a, L., Valiente, D., Jiang, X., and
Reinoso, O. (2019). An evaluation between global
appearance descriptors based on analytic methods
and deep learning techniques for localization in au-
tonomous mobile robots.
Dalal, N. and Triggs, B. (2005). Histograms of oriented gra-
dients for human detection. In 2005 IEEE Computer
Society Conference on Computer Vision and Pattern
Recognition (CVPR’05), volume 1, pages 886–893
vol. 1.
Gil, A., Valiente, D., Reinoso,
´
O., Fern
´
andez, L., and
Mar
´
ın, J. M. (2011). Building visual maps with a
single omnidirectional camera. In ICINCO (2), pages
145–154.
Hofmeister, M., Liebsch, M., and Zell, A. (2009). Vi-
sual self-localization for small mobile robots with
weighted gradient orientation histograms. In 40th In-
ternational Symposium on Robotics (ISR), pages 87–
91. Barcelona.
Lowe, D. G. (2004). Distinctive image features from scale-
invariant keypoints. International journal of computer
vision, 60(2):91–110.
Menegatti, E., Maeda, T., and Ishiguro, H. (2004). Image-
based memory for robot navigation using properties
of omnidirectional images. Robotics and Autonomous
Systems, 47(4):251 – 267.
Murillo, A. C., Guerrero, J. J., and Sagues, C. (2007). Surf
features for efficient robot localization with omnidi-
rectional images. In Robotics and Automation, 2007
IEEE International Conference on, pages 3901–3907.
IEEE.
Oliva, A. and Torralba, A. (2001). Modeling the shape
of the scene: A holistic representation of the spatial
envelope. International journal of computer vision,
42(3):145–175.
Oliva, A. and Torralba, A. (2006). Building the gist of a
scene: The role of global image features in recogni-
tion. Progress in brain research, 155:23–36.
Pay
´
a, L., Fern
´
andez, L., Reinoso,
´
O., Gil, A., and
´
Ubeda, D. (2009). Appearance-based dense maps
creation-comparison of compression techniques with
panoramic images. In ICINCO-RA, pages 250–255.
Pay
´
a, L., Gil, A., and Reinoso, O. (2017). A state-of-the-art
review on mapping and localization of mobile robots
using omnidirectional vision sensors. Journal of Sen-
sors, 2017.
Pay
´
a, L., Peidr
´
o, A., Amor
´
os, F., Valiente, D., and Reinoso,
O. (2018). Modeling environments hierarchically with
omnidirectional imaging and global-appearance de-
scriptors. Remote Sensing, 10(4):522.
Pronobis, A. and Caputo, B. (2009). COLD: COsy Lo-
calization Database. The International Journal of
Robotics Research (IJRR), 28(5):588–594.
Radon, J. (2005). 1.1
¨
uber die bestimmung von funktio-
nen durch ihre integralwerte l
¨
angs gewisser mannig-
faltigkeiten. Classic papers in modern diagnostic ra-
diology, 5:21.
Rom
´
an, V., Pay
´
a, L., Flores, M., Cebollada, S., and
Reinoso,
´
O. (2019). Performance of new global ap-
pearance description methods in localization of mo-
bile robots. In Iberian Robotics conference, pages
351–363. Springer.
Rom
´
an, V., Pay
´
a, L., and Reinoso,
´
O. (2018). Evaluating
the robustness of global appearance descriptors in a
visual localization task, under changing lighting con-
ditions. In ICINCO-RA, pages 258–265.
Siagian, C. and Itti, L. (2009). Biologically inspired mo-
bile robot vision localization. IEEE Transactions on
Robotics, 25(4):861–873.
Sturm, P., Ramalingam, S., Tardif, J.-P., Gasparini, S., Bar-
reto, J., et al. (2011). Camera models and fundamental
concepts used in geometric computer vision. Founda-
tions and Trends
R
in Computer Graphics and Vision,
6(1–2):1–183.
Su, Z., Zhou, X., Cheng, T., Zhang, H., Xu, B., and Chen,
W. (2017). Global localization of a mobile robot us-
ing lidar and visual features. In 2017 IEEE Interna-
tional Conference on Robotics and Biomimetics (RO-
BIO), pages 2377–2383. IEEE.
Valiente Garc
´
ıa, D., Fern
´
andez Rojo, L., Gil Aparicio, A.,
Pay
´
a Castell
´
o, L., and Reinoso Garc
´
ıa, O. (2012). Vi-
sual odometry through appearance-and feature-based
method with omnidirectional images. Journal of
Robotics, 2012.
Xu, S., Chou, W., and Dong, H. (2019). A robust indoor lo-
calization system integrating visual localization aided
by cnn-based image retrieval with monte carlo local-
ization. Sensors, 19(2):249.
Zhou, X., Su, Z., Huang, D., Zhang, H., Cheng, T., and Wu,
J. (2018). Robust global localization by using global
visual features and range finders data. In 2018 IEEE
International Conference on Robotics and Biomimet-
ics (ROBIO), pages 218–223. IEEE.
ICINCO 2020 - 17th International Conference on Informatics in Control, Automation and Robotics
384