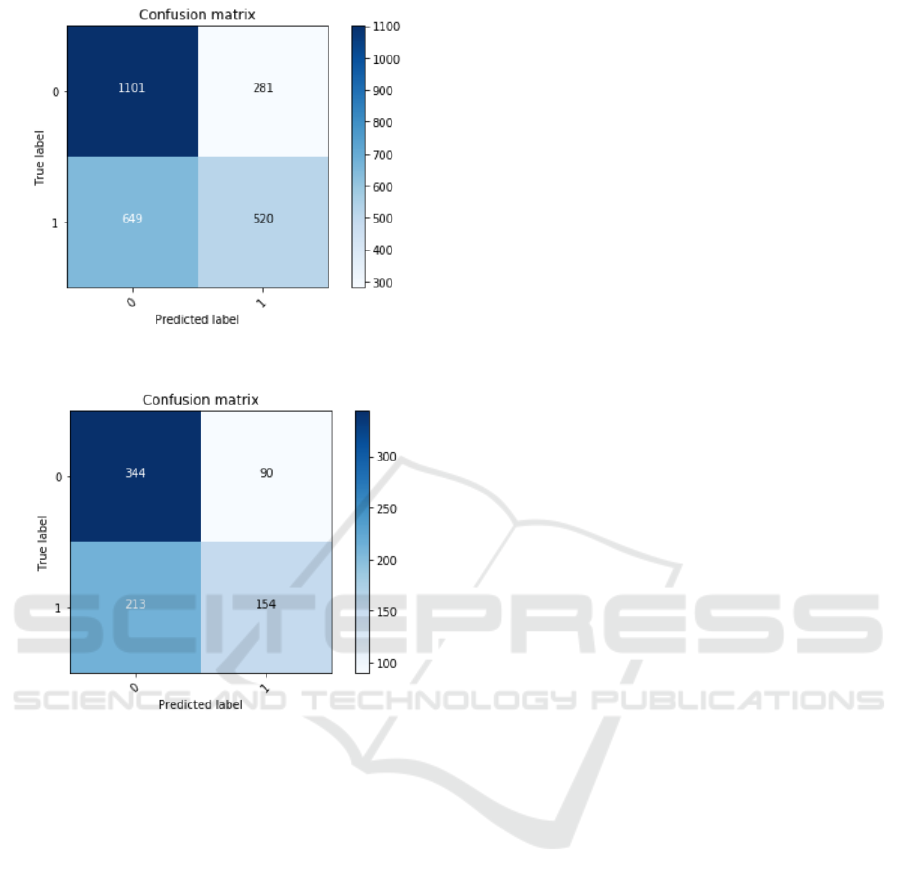
Figure 11: Confusion Matrix for LIAR dataset.
Figure 12: Confusion Matrix for Polifact dataset.
found that the most accurate results can be obtained
with logistic regression based algorithms. As a future
work, we would like to extend our analysis by better
considering also user profiles’ features and some kind
of dynamic analysis of news diffusion mechanism in
our fake news detection model.
REFERENCES
Agrawal et al., D. (2012). Challenges and opportunities
with big data. A community white paper developed
by leading researchers across the United States. Tech-
nical report, Purdue University.
Allcott, H. and Gentzkow, M. (2017). Social media and
fake news in the 2016 election. Working Paper 23089,
National Bureau of Economic Research.
Boselli, R., Cesarini, M., Mercorio, F., and Mezzanzan-
ica, M. (2018). Classifying online job advertisements
through machine learning. Future Generation Com-
puter Systems, 86:319 – 328.
Cassavia, N., Masciari, E., Pulice, C., and Sacc
`
a, D. (2017).
Discovering user behavioral features to enhance infor-
mation search on big data. TiiS, 7(2):7:1–7:33.
Culpepper, J. S., Moffat, A., Bennett, P. N., and Lerman, K.,
editors (2019). Proceedings of the Twelfth ACM Inter-
national Conference on Web Search and Data Min-
ing, WSDM 2019, Melbourne, VIC, Australia, Febru-
ary 11-15, 2019. ACM.
Flach, P. A. and Kull, M. (2015). Precision-recall-gain
curves: PR analysis done right. In Cortes, C.,
Lawrence, N. D., Lee, D. D., Sugiyama, M., and Gar-
nett, R., editors, Advances in Neural Information Pro-
cessing Systems 28: Annual Conference on Neural In-
formation Processing Systems 2015, December 7-12,
2015, Montreal, Quebec, Canada, pages 838–846.
Guo, C., Cao, J., Zhang, X., Shu, K., and Yu, M. (2019).
Exploiting emotions for fake news detection on social
media. CoRR, abs/1903.01728.
Masciari, E. (2012). SMART: stream monitoring enterprise
activities by RFID tags. Inf. Sci., 195:25–44.
Masood, R. and Aker, A. (2018). The fake news challenge:
Stance detection using traditional machine learning
approaches.
Mezzanzanica, M., Boselli, R., Cesarini, M., and Mercorio,
F. (2015). A model-based approach for developing
data cleansing solutions. Journal of Data and Infor-
mation Quality (JDIQ), 5(4):1–28.
Potthast, M., Kiesel, J., Reinartz, K., Bevendorff, J., and
Stein, B. (2017). A stylometric inquiry into hyperpar-
tisan and fake news. CoRR, abs/1702.05638.
Shabani, S. and Sokhn, M. (2018). Hybrid machine-crowd
approach for fake news detection. In 2018 IEEE 4th
International Conference on Collaboration and Inter-
net Computing (CIC), pages 299–306. IEEE.
Shu, K., Sliva, A., Wang, S., Tang, J., and Liu, H. (2017a).
Fake news detection on social media: A data mining
perspective. CoRR, abs/1708.01967.
Shu, K., Sliva, A., Wang, S., Tang, J., and Liu, H. (2017b).
Fake news detection on social media: A data mining
perspective. CoRR, abs/1708.01967.
Shu, K., Wang, S., and Liu, H. (2019). Beyond news con-
tents: The role of social context for fake news detec-
tion. In (Culpepper et al., 2019), pages 312–320.
Vosoughi, S., Roy, D., and Aral, S. (2018). The spread of
true and false news online. Science, 359(6380):1146–
1151.
Zhou, X., Zafarani, R., Shu, K., and Liu, H. (2019a). Fake
news: Fundamental theories, detection strategies and
challenges. In (Culpepper et al., 2019), pages 836–
837.
Zhou, Z., Guan, H., Bhat, M. M., and Hsu, J. (2019b). Fake
news detection via nlp is vulnerable to adversarial at-
tacks. arXiv preprint arXiv:1901.09657.
Leveraging Machine Learning for Fake News Detection
157