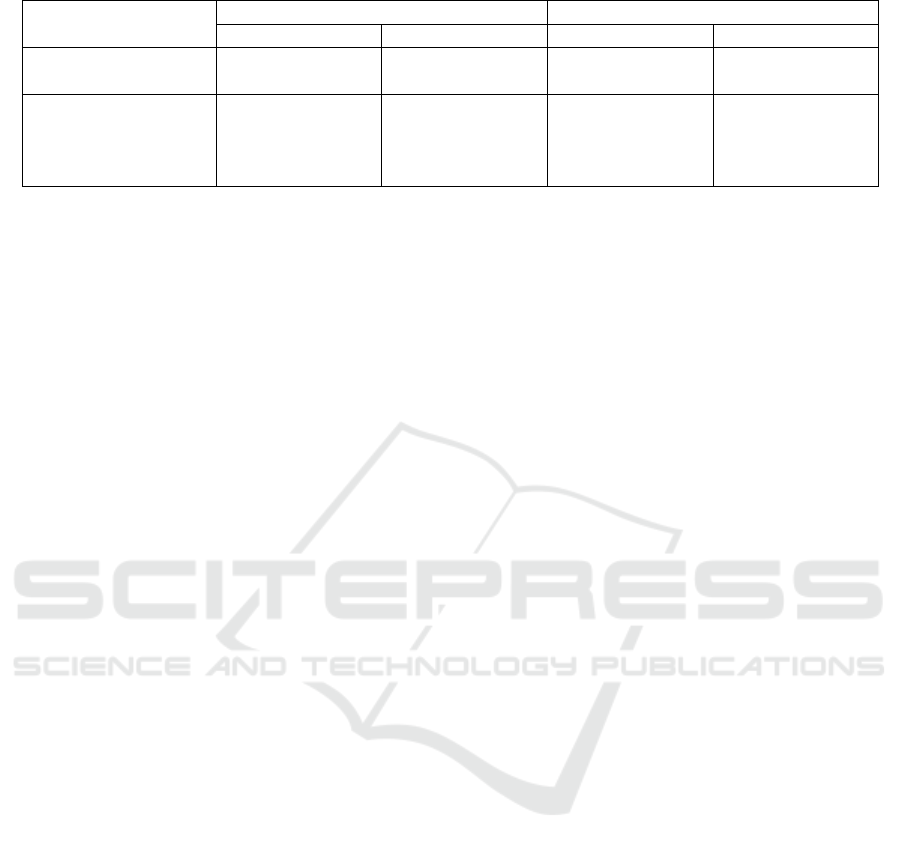
Table 5: Thirty-day Decision-making Prediction Accuracy in Production.
Model Stochastic Process model Regression Model with Xgboost
APC APD APC APD
Overall 78.1% 58.4% 39.5% 23.7%
[75.1%, 80.8%] [54.9%, 61.8%] [36.1%, 42.9%] [20.1%, 25.9%]
duration < 15 days 79.2% 40.2% 40% 20%
[75.1%, 83.2%] [35.3%, 45.0%] [35.1%, 44.8%] [16.0%, 23.9%]
duration ≥ 15 days 77.1% 75.8% 39.1% 27%
[73.0%, 81.1%] [71.6%, 79.9%] [34.2%, 43.7%] [22.6%, 31.3%]
experiments in the offline-payment business at Ant
Financial. Possible explanation of the results is also
given. The BG/NBD model has been productionized.
Production results show the effectiveness of the pro-
posed methodology. Analysis of the effect of train-
ing duration on prediction accuracy is also conducted.
This analysis is very useful to guide the operation of
controlled experiments, e.g., decide the run time of
experiments. Two possible future directions are as
follows. First, although the stochastic process mod-
els are from the marketing area, they can be used to
model any counting metric. Hence extension to other
counting metrics in Ant Financial is desired. The sec-
ond direction is to extend the stochastic process mod-
els to achieve higher prediction accuracy, e.g., relax
the lack of memory assumption, add nonstationarity,
etc.
REFERENCES
Abe, M. (2009). “counting your customers” one by one: A
hierarchical bayes extension to the pareto/nbd model.
Marketing Science, 28(3):541–553.
Bakshy, E., Eckles, D., and Bernstein, M. S. (2014). De-
signing and deploying online field experiments. In
Proceedings of the 23rd international conference on
World wide web, pages 283–292. ACM.
Dahana, W. D., Miwa, Y., and Morisada, M. (2019).
Linking lifestyle to customer lifetime value: An ex-
ploratory study in an online fashion retail market.
Journal of Business Research, 99:319–331.
Dechant, A., Spann, M., and Becker, J. U. (2019). Posi-
tive customer churn: An application to online dating.
Journal of Service Research, 22(1):90–100.
Dmitriev, P., Frasca, B., Gupta, S., Kohavi, R., and Vaz, G.
(2016). Pitfalls of long-term online controlled exper-
iments. In Big Data (Big Data), 2016 IEEE Interna-
tional Conference on, pages 1367–1376. IEEE.
Dziurzynski, L., Wadsworth, E., and McCarthy, D. (2014).
BTYD: Implementing Buy ’Til You Die Models. URL
http://CRAN.R-project.org/package=BTYD. R pack-
age version, 2.
Ehrenberg, A. S. C. (1972). Repeat-buying; theory and ap-
plications. North-Holland Pub. Co.
Fader, P. S. and Hardie, B. G. (2007). How to project
customer retention. Journal of Interactive Marketing,
21(1):76–90.
Fader, P. S., Hardie, B. G., and Lee, K. L. (2005). “counting
your customers” the easy way: An alternative to the
pareto/nbd model. Marketing science, 24(2):275–284.
Fader, P. S., Hardie, B. G., and Lee, K. L. (2008). Comput-
ing p(alive) using the bg/nbd model.
Fader, P. S., Hardie, B. G., and Shang, J. (2010). Customer-
base analysis in a discrete-time noncontractual setting.
Marketing Science, 29(6):1086–1108.
Gomez-Uribe, C. A. and Hunt, N. (2016). The netflix rec-
ommender system: Algorithms, business value, and
innovation. ACM Transactions on Management Infor-
mation Systems (TMIS), 6(4):13.
Hohnhold, H., O’Brien, D., and Tang, D. (2015). Focusing
on the long-term: It’s good for users and business. In
Proceedings of the 21th ACM SIGKDD International
Conference on Knowledge Discovery and Data Min-
ing, pages 1849–1858. ACM.
Karlin, S. (2014). A first course in stochastic processes.
Academic press.
Kohavi, R., Deng, A., Frasca, B., Longbotham, R., Walker,
T., and Xu, Y. (2012). Trustworthy online controlled
experiments: Five puzzling outcomes explained. In
Proceedings of the 18th ACM SIGKDD international
conference on Knowledge discovery and data mining,
pages 786–794. ACM.
Kohavi, R., Henne, R. M., and Sommerfield, D. (2007).
Practical guide to controlled experiments on the web:
listen to your customers not to the hippo. In Proceed-
ings of the 13th ACM SIGKDD international confer-
ence on Knowledge discovery and data mining, pages
959–967. ACM.
Lee, M. R. and Shen, M. (2018). Winner’s curse: Bias
estimation for total effects of features in online con-
trolled experiments. In Proceedings of the 24th
ACM SIGKDD International Conference on Knowl-
edge Discovery & Data Mining, pages 491–499.
ACM.
Roy, R. K. (2001). Design of experiments using the Taguchi
approach: 16 steps to product and process improve-
ment. John Wiley & Sons.
Schmittlein, D. C., Morrison, D. G., and Colombo, R.
(1987). Counting your customers: Who-are they
and what will they do next? Management science,
33(1):1–24.
Auxiliary Decision-making for Controlled Experiments based on Mid-term Treatment Effect Prediction: Applications in Ant Financial’s
Offline-payment Business
29