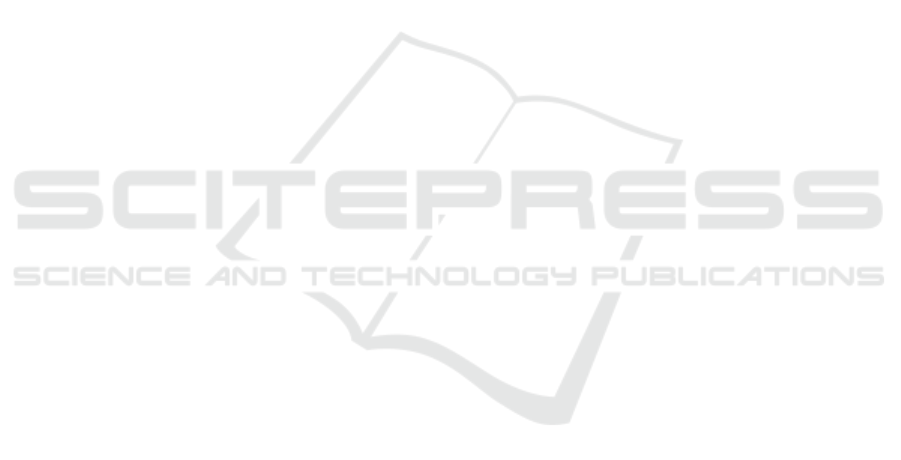
Ling, Y., Hasan, S. A., Datla, V. V., Qadir, A., Lee, K.,
Liu, J., and Farri, O. (2017). Learning to diagnose:
Assimilating clinical narratives using deep reinforce-
ment learning. In IJCNLP.
Llorca, D. F., Parra, I., Sotelo, M.
´
A., and Lacey, G. (2011).
A vision-based system for automatic hand washing
quality assessment. Machine Vision and Applications,
22(2):219–234.
M.A.Sc., A. M., P.Eng., Ph.D., G. R. F., P.Eng., Ph.D., J.
C. B., and C.Eng. (2001). The use of artificial intel-
ligence in the design of an intelligent cognitive ortho-
sis for people with dementia. Assistive Technology,
13(1):23–39.
Mozer, M. C. (2005). Lessons from an Adaptive Home,
chapter 12, pages 271–294. John Wiley Sons Ltd.
Muddasar Naeem, Antonio Coronato, G. P. G. D. P. (2020).
A cnn based monitoring system to minimize medica-
tion errors during treatment process at home.
Murphy, S. A., Oslin, D. W., Rush, A. J., and Zhu, J. (2006).
Methodological challenges in constructing effective
treatment sequences for chronic psychiatric disorders.
Neuropsychopharmacology, 32(2):257–262.
Mynatt, E. D., Essa, I., and Rogers, W. (2000). Increasing
the opportunities for aging in place. In Proceedings
on the 2000 Conference on Universal Usability, CUU
’00, pages 65–71, New York, NY, USA. ACM.
Oliver, N., Garg, A., and Horvitz, E. (2004). Layered rep-
resentations for learning and inferring office activity
from multiple sensory channels. volume 96, pages
163–180. Elsevier.
Patel, V. L., Shortliffe, E. H., Stefanelli, M., Szolovits, P.,
Berthold, M. R., Bellazzi, R., and Abu-Hanna, A.
(2009). The coming of age of artificial intelligence in
medicine. Artificial Intelligence in Medicine, 46(1):5
– 17. Artificial Intelligence in Medicine AIME’ 07.
Petersen, B. K., Yang, J., Grathwohl, W. S., Cockrell, C.,
Santiago, C., An, G., and Faissol, D. M. (2018). Preci-
sion medicine as a control problem: Using simulation
and deep reinforcement learning to discover adaptive,
personalized multi-cytokine therapy for sepsis. CoRR,
abs/1802.10440.
Pineau, J., Montemerlo, M., Pollack, M., Roy, N., and
Thrun, S. (2003). Towards robotic assistants in nurs-
ing homes: Challenges and results.
Prasad, N., Cheng, L., Chivers, C., Draugelis, M., and En-
gelhardt, B. E. (2017). A reinforcement learning ap-
proach to weaning of mechanical ventilation in inten-
sive care units. CoRR, abs/1704.06300.
Reinkensmeyer, D. J., Guigon, E., and Maier, M. A. (2012).
A computational model of use-dependent motor re-
covery following a stroke: Optimizing corticospinal
activations via reinforcement learning can explain
residual capacity and other strength recovery dynam-
ics. Neural networks : the official journal of the Inter-
national Neural Network Society, 29-30:60–9.
Ross, C. K., Steward, C. A., and Sinacore, J. M. (1993). The
importance of patient preferences in the measurement
of health care satisfaction. Medical care, pages 1138–
1149.
Russell, S. and Norvig, P. (2009). Artificial Intelligence: A
Modern Approach. Prentice Hall Press, Upper Saddle
River, NJ, USA, 3rd edition.
Sahba, F., Tizhoosh, H. R., and Salama, M. M. A. (2006). A
reinforcement learning framework for medical image
segmentation. In The 2006 IEEE International Joint
Conference on Neural Network Proceedings, pages
511–517.
Strens, M. J. A. (2000). A bayesian framework for re-
inforcement learning. In Proceedings of the Seven-
teenth International Conference on Machine Learn-
ing, ICML ’00, pages 943–950, San Francisco, CA,
USA. Morgan Kaufmann Publishers Inc.
Suresh, H., Hunt, N., Johnson, A. E. W., Celi, L. A.,
Szolovits, P., and Ghassemi, M. (2017). Clinical inter-
vention prediction and understanding using deep net-
works. CoRR, abs/1705.08498.
Sutton, R. S. and Barto, A. G. (1998). Reinforcement Learn-
ing: An Introduction. MIT Press.
Thall, P. F. and Wathen, J. K. (2005). Covariate-
adjusted adaptive randomization in a sarcoma trial
with multi-stage treatments. Statistics in Medicine,
24(13):1947–1964.
Welling, M. and Teh, Y. W. (2011). Bayesian learning via
stochastic gradient langevin dynamics. In Proceed-
ings of the 28th International Conference on Inter-
national Conference on Machine Learning, ICML’11,
pages 681–688, USA. Omnipress.
Wren, C. R., Azarbayejani, A., Darrell, T., and Pentland,
A. (1997). Pfinder: Real-time tracking of the hu-
man body. IEEE Trans. Pattern Anal. Mach. Intell.,
19:780–785.
AI4EIoTs 2020 - Special Session on Artificial Intelligence for Emerging IoT Systems: Open Challenges and Novel Perspectives
498