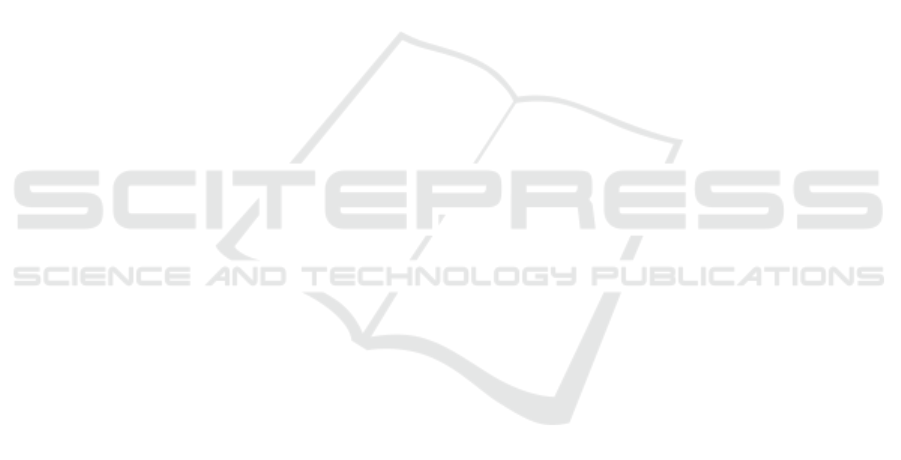
the increase in the number of features as it adds more
separability power to it. The F1-measure closely fol-
lows the accuracy in almost all cases. This means that
our models correctly classify each person correctly
without any bias towards a single class/individual.
7 CONCLUSIONS AND FUTURE
WORK
In this work, a novel multi-sensory dataset was pre-
sented with detailed demonstration of all the steps
taken for its construction. Additionally, three differ-
ent machine learning algorithms were used to eval-
uate the new dataset, and to explore the correlation
between the on-body location of sensory device -
with the model accuracy as well as, the effect of fus-
ing pairs of sensory data from different body loca-
tions on the resultant predictive performance. It turns
out that, the sensory devices mounted on the lower
body achieved better performance (up to 99% accu-
racy) than sensors worn on the wrist or on the upper
arm. However, the sensory device mounted on the
back achieved a comparable accuracy despite being
far away from the gait.
It was also shown that fusing sensory data from
two different locations help increasing the classifica-
tion accuracy above the low-accuracy single location,
and increases the accuracy significantly if fused with
a device mounted on one of the three locations in the
lower body (up to 98%).
Finally, the data collected in this work included
only walking activity. However, in the future, we
would like to explore more activities such as: brush-
ing teeth, climbing stairs, jogging, etc., and investi-
gate if they can constitute bio-metric features for dif-
ferent people. We would also like to study the impact
of sensory devices’ location on the identification per-
formance for each activity.
ACKNOWLEDGEMENTS
This work is funded by the Information Technol-
ogy Industry Development Agency (ITIDA), Infor-
mation Technology Academia Collaboration (ITAC)
Program, Egypt – Grant Number (PRP2019.R26.1 - A
Robust Wearable Activity Recognition System based
on IMU Signals).
REFERENCES
Abdu-Aguye, M. G. and Gomaa, W. (2019). Competitive
Feature Extraction for Activity Recognition based on
Wavelet Transforms and Adaptive Pooling. In 2019
International Joint Conference on Neural Networks
(IJCNN), pages 1–8. ISSN: 2161-4393.
Cola, G., Avvenuti, M., Musso, F., and Vecchio, A. (2016).
Gait-based authentication using a wrist-worn device.
In Proceedings of the 13th International Conference
on Mobile and Ubiquitous Systems: Computing, Net-
working and Services, MOBIQUITOUS 2016, page
208–217, New York, NY, USA. Association for Com-
puting Machinery.
Gomaa, W., Elbasiony, R., and Ashry, S. (2017). Adl
classification based on autocorrelation function of
inertial signals. In 2017 16th IEEE International
Conference on Machine Learning and Applications
(ICMLA), pages 833–837.
Kwapisz, J. R., Weiss, G. M., and Moore, S. A. (2010).
Cell phone-based biometric identification. In 2010
Fourth IEEE International Conference on Biometrics:
Theory, Applications and Systems (BTAS), pages 1–7.
ISSN: null.
Lara, O. D. and Labrador, M. A. (2013). A Sur-
vey on Human Activity Recognition using Wearable
Sensors. IEEE Communications Surveys Tutorials,
15(3):1192–1209.
Mondol, M. A. S., Emi, I. A., Preum, S. M., and Stankovic,
J. A. (2017). Poster Abstract: User Authentication
Using Wrist Mounted Inertial Sensors. In 2017 16th
ACM/IEEE International Conference on Information
Processing in Sensor Networks (IPSN), pages 309–
310. ISSN: null.
Murray, M., Drought, A., and Kory, R. (1964). Walking
patterns of normal men. The Journal of bone and joint
surgery. American volume, 46:335—360.
Ngo, T. T., Makihara, Y., Nagahara, H., Mukaigawa, Y.,
and Yagi, Y. (2014). The largest inertial sensor-
based gait database and performance evaluation of
gait-based personal authentication. Pattern Recogni-
tion, 47(1):228–237.
Patel, S., Park, H., Bonato, P., Chan, L., and Rodgers, M.
(2012). A review of wearable sensors and systems
with application in rehabilitation. Journal of Neuro-
Engineering and Rehabilitation, 9(1):21.
Shumway, R. H. and Stoffer, D. S. Time series analysis and
its applications : with R examples. Springer, Cham.
Tao, S., Zhang, X., Cai, H., Lv, Z., Hu, C., and Xie, H.
(2018). Gait based biometric personal authentication
by using MEMS inertial sensors. Journal of Ambient
Intelligence and Humanized Computing, 9(5):1705–
1712.
ICINCO 2020 - 17th International Conference on Informatics in Control, Automation and Robotics
628