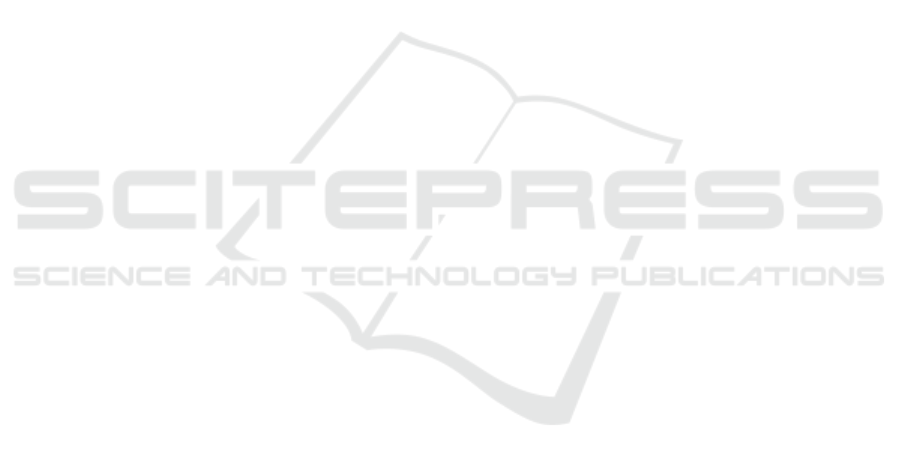
ACKNOWLEDGMENTS
This work is funded by the Information Technol-
ogy Industry Development Agency (ITIDA), Infor-
mation Technology Academia Collaboration (ITAC)
Program, Egypt – Grant Number (PRP2019.R26.1 - A
Robust Wearable Activity Recognition System based
on IMU Signals).
We also would like to thank all the students who
participated in collecting the dataset.
REFERENCES
Abdu-Aguye, M. G. and Gomaa, W. (2019). Competi-
tive feature extraction for activity recognition based
on wavelet transforms and adaptive pooling. In 2019
International Joint Conference on Neural Networks
(IJCNN), pages 1–8.
Assam, R. and Seidl, T. (2014). Activity recognition from
sensors using dyadic wavelets and hidden markov
model. In 2014 IEEE 10th International Conference
on Wireless and Mobile Computing, Networking and
Communications (WiMob), pages 442–448.
Casale, P., Pujol, O., and Radeva, P. (2011). Human activity
recognition from accelerometer data using a wearable
device. In Iberian Conference on Pattern Recognition
and Image Analysis, pages 289–296. Springer.
Feng, Z., Mo, L., and Li, M. (2015). A random forest-based
ensemble method for activity recognition. In 2015
37th Annual International Conference of the IEEE En-
gineering in Medicine and Biology Society (EMBC),
pages 5074–5077. IEEE.
Garofalo, G., Argones R
´
ua, E., Preuveneers, D., Joosen, W.,
et al. (2019). A systematic comparison of age and gen-
der prediction on imu sensor-based gait traces. Sen-
sors, 19(13):2945.
Jain, A. and Kanhangad, V. (2016). Investigating gen-
der recognition in smartphones using accelerometer
and gyroscope sensor readings. In 2016 International
Conference on Computational Techniques in Infor-
mation and Communication Technologies (ICCTICT),
pages 597–602.
Lu, J., Wang, G., and Moulin, P. (2014). Human identity
and gender recognition from gait sequences with arbi-
trary walking directions. IEEE Transactions on Infor-
mation Forensics and Security, 9(1):51–61.
MBIENTLAB (2018). https://mbientlab.com.
Mehrang, S., Pietil
¨
a, J., and Korhonen, I. (2018). An activ-
ity recognition framework deploying the random for-
est classifier and a single optical heart rate monitor-
ing and triaxial accelerometer wrist-band. Sensors,
18(2):613.
Ngo, T. T., Ahad, M. A. R., Antar, A. D., Ahmed, M., Mura-
matsu, D., Makihara, Y., Yagi, Y., Inoue, S., Hossain,
T., and Hattori, Y. (2019). Ou-isir wearable sensor-
based gait challenge: Age and gender. In Proceedings
of the 12th IAPR International Conference on Biomet-
rics, ICB.
Ngo, T. T., Makihara, Y., Nagahara, H., Mukaigawa, Y.,
and Yagi, Y. (2014). The largest inertial sensor-
based gait database and performance evaluation of
gait-based personal authentication. Pattern Recogni-
tion, 47(1):228–237.
Rosli, N. A. I. M., Rahman, M. A. A., Balakrishnan, M.,
Komeda, T., Mazlan, S. A., and Zamzuri, H. (2017).
Improved gender recognition during stepping activity
for rehab application using the combinatorial fusion
approach of emg and hrv. Applied Sciences, 7(4):348.
Shepstone, S. E., Tan, Z.-H., and Jensen, S. H. (2013). De-
mographic recommendation by means of group profile
elicitation using speaker age and gender recognition.
In INTERSPEECH, pages 2827–2831.
Taspinar, A. (2018). A guide for using the
wavelet transform in machine learning.
http://ataspinar.com/2018/12/21/a-guide-for-using-
the-wavelet-transform-in-machine-learning.
Yuchimiuk, J. (2007). System and method for gender iden-
tification in a speech application environment. US
Patent App. 10/186,049.
Zhang, K., Gao, C., Guo, L., Sun, M., Yuan, X., Han, T. X.,
Zhao, Z., and Li, B. (2017). Age group and gender
estimation in the wild with deep ror architecture. IEEE
Access, 5:22492–22503.
Zhenyu He (2010). Activity recognition from accelerome-
ter signals based on wavelet-ar model. In 2010 IEEE
International Conference on Progress in Informatics
and Computing, volume 1, pages 499–502.
ICINCO 2020 - 17th International Conference on Informatics in Control, Automation and Robotics
636