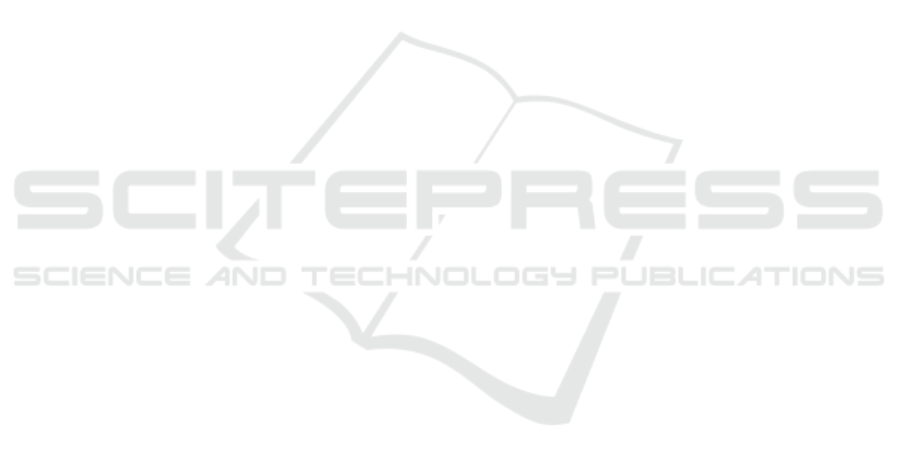
ages using circular hough transform and grow-cut al-
gorithm. PeerJ, 4:e2003.
Al-Bander, B., Williams, B. M., Al-Nuaimy, W., Al-Taee,
M. A., Pratt, H., and Zheng, Y. (2018). Dense fully
convolutional segmentation of the optic disc and cup
in colour fundus for glaucoma diagnosis. Symmetry,
10(4):87.
Aquino, A., Geg
´
undez-Arias, M. E., and Mar
´
ın, D. (2010).
Detecting the optic disc boundary in digital fundus im-
ages using morphological, edge detection, and feature
extraction techniques. IEEE transactions on medical
imaging, 29(11):1860–1869.
Bajwa, M. N., Malik, M. I., Siddiqui, S. A., Dengel, A.,
Shafait, F., Neumeier, W., and Ahmed, S. (2019).
Two-stage framework for optic disc localization and
glaucoma classification in retinal fundus images using
deep learning. BMC medical informatics and decision
making, 19(1):136.
Bourne, R. R., Flaxman, S. R., Braithwaite, T., Cicinelli,
M. V., Das, A., Jonas, J. B., Keeffe, J., Kempen, J. H.,
Leasher, J., Limburg, H., et al. (2017). Magnitude,
temporal trends, and projections of the global preva-
lence of blindness and distance and near vision im-
pairment: a systematic review and meta-analysis. The
Lancet Global Health, 5(9):e888–e897.
Carmona, E. J., Rinc
´
on, M., Garc
´
ıa-Feijo
´
o, J., and
Mart
´
ınez-de-la Casa, J. M. (2008). Identification of
the optic nerve head with genetic algorithms. Artifi-
cial Intelligence in Medicine, 43(3):243–259.
Chen, X., Xu, Y., Yan, S., Wong, D. W. K., Wong, T. Y.,
and Liu, J. (2015). Automatic feature learning for
glaucoma detection based on deep learning. In In-
ternational Conference on Medical Image Computing
and Computer-Assisted Intervention, pages 669–677.
Springer.
Civit Masot, J., Luna Perej
´
on, F., Dur
´
an L
´
opez, L.,
Dom
´
ınguez Morales, J. P., Vicente D
´
ıaz, S.,
Linares Barranco, A., and Civit Balcells, A. (2019).
Multi-dataset training for medical image segmentation
as a service. In UCCI 2019: 11th International Joint
Conference on Computational Intelligence (2019), p
542-547. ScitePress Digital Library.
Dice, L. R. (1945). Measures of the amount of ecologic
association between species. Ecology, 26(3):297–302.
Fan, Z., Rong, Y., Cai, X., Lu, J., Li, W., Lin, H., and Chen,
X. (2017). Optic disk detection in fundus image based
on structured learning. IEEE journal of biomedical
and health informatics, 22(1):224–234.
Federation, I. (2013). International diabetic federation atlas;
chapter 2 the global burden.
Forsyth, D. A. and Ponce, J. (2002). Computer vision: a
modern approach. Prentice Hall Professional Techni-
cal Reference.
Fraz, M. M., Welikala, R., Rudnicka, A. R., Owen, C. G.,
Strachan, D., and Barman, S. A. (2015). Quartz:
Quantitative analysis of retinal vessel topology and
size–an automated system for quantification of reti-
nal vessels morphology. Expert Systems with Appli-
cations, 42(20):7221–7234.
Fu, H., Cheng, J., Xu, Y., Wong, D. W. K., Liu, J., and Cao,
X. (2018). Joint optic disc and cup segmentation based
on multi-label deep network and polar transformation.
IEEE transactions on medical imaging, 37(7):1597–
1605.
Fumero, F., Alay
´
on, S., Sanchez, J. L., Sigut, J., and
Gonzalez-Hernandez, M. (2011). Rim-one: An open
retinal image database for optic nerve evaluation.
In 2011 24th international symposium on computer-
based medical systems (CBMS), pages 1–6. IEEE.
Gao, Y., Yu, X., Wu, C., Zhou, W., Wang, X., and Chu, H.
(2019). Accurate and efficient segmentation of optic
disc and optic cup in retinal images integrating multi-
view information. IEEE Access, 7:148183–148197.
He, K., Zhang, X., Ren, S., and Sun, J. (2016). Deep resid-
ual learning for image recognition. In Proceedings of
the IEEE conference on computer vision and pattern
recognition, pages 770–778.
Huang, G., Liu, Z., Van Der Maaten, L., and Weinberger,
K. Q. (2017). Densely connected convolutional net-
works. In Proceedings of the IEEE conference on
computer vision and pattern recognition, pages 4700–
4708.
Jackson, A. and Radhakrishnan, S. (2014). Understanding
and living with glaucoma. Glaucoma Research Foun-
dation.
Joshua, A. O., Nelwamondo, F. V., and Mabuza-Hocquet,
G. (2019). Segmentation of optic cup and disc
for diagnosis of glaucoma on retinal fundus im-
ages. In 2019 Southern African Universities Power
Engineering Conference/Robotics and Mechatron-
ics/Pattern Recognition Association of South Africa
(SAUPEC/RobMech/PRASA), pages 183–187. IEEE.
Long, J., Shelhamer, E., and Darrell, T. (2015). Fully con-
volutional networks for semantic segmentation. In
Proceedings of the IEEE conference on computer vi-
sion and pattern recognition, pages 3431–3440.
Maninis, K.-K., Pont-Tuset, J., Arbel
´
aez, P., and Van Gool,
L. (2016). Deep retinal image understanding. In In-
ternational conference on medical image computing
and computer-assisted intervention, pages 140–148.
Springer.
Mookiah, M. R. K., Acharya, U. R., Lim, C. M., Petznick,
A., and Suri, J. S. (2012). Data mining technique
for automated diagnosis of glaucoma using higher or-
der spectra and wavelet energy features. Knowledge-
Based Systems, 33:73–82.
Morales, S., Naranjo, V., Angulo, J., and Alca
˜
niz, M.
(2013). Automatic detection of optic disc based on
pca and mathematical morphology. IEEE transactions
on medical imaging, 32(4):786–796.
Quigley, H. A. and Broman, A. T. (2006). The number of
people with glaucoma worldwide in 2010 and 2020.
British journal of ophthalmology, 90(3):262–267.
Ramani, R. G. and Shanthamalar, J. J. (2020). Improved im-
age processing techniques for optic disc segmentation
in retinal fundus images. Biomedical Signal Process-
ing and Control, 58:101832.
Rehman, Z. U., Naqvi, S. S., Khan, T. M., Arsalan, M.,
Khan, M. A., and Khalil, M. (2019). Multi-parametric
DeLTA 2020 - 1st International Conference on Deep Learning Theory and Applications
28