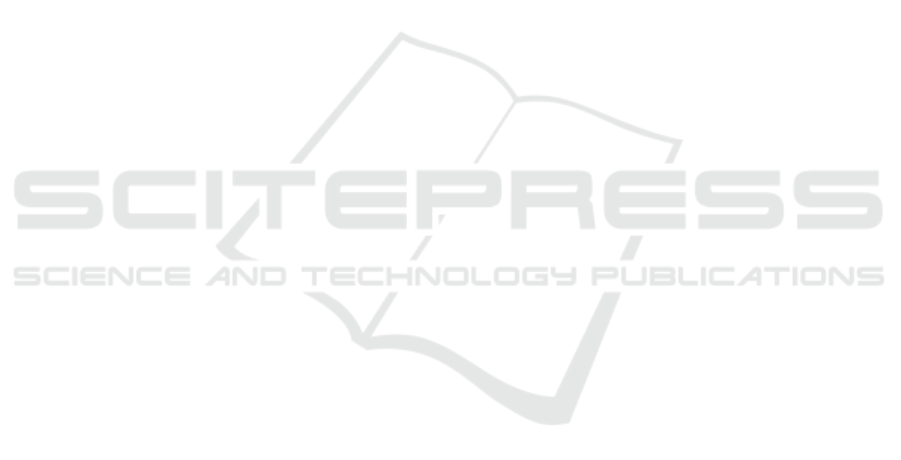
Krebs, F., B
¨
ock, S., and Widmer, G. (2015). An efficient
state-space model for joint tempo and meter tracking.
In Proc. of the Int. Society for Music Information Re-
trieval Conference, pages 72–78.
Lerch, A., Arthur, C., Pati, A., and Gururani, S. (2019). Mu-
sic Performance Analysis: A Survey. In Proc. of the
Int. Society for Music Information Retrieval Confer-
ence, pages 33–43.
Luo, Y. J., Su, L., Yang, Y. H., and Chi, T. S. (2015). De-
tection of common mistakes in novice violin playing.
In Proc. of the Int. Society for Music Information Re-
trieval Conference, pages 316–322.
Maestre, E. and G
´
omez, E. (2005). Automatic characteriza-
tion of dynamics and articulation of expressive mono-
phonic recordings. In Proc. of the Audio Engineering
Society Convention, volume 1, pages 26–33.
Mantie, R. (2019). The Philosophy of Assessment in Mu-
sic Education. In The Oxford handbook of assessment
policy and practice in music education, Volume 1 (pp.
32–55). Oxford University Press.
Mauch, M. and Dixon, S. (2014). PYIN: A fundamental fre-
quency estimator using probabilistic threshold distri-
butions. In Proc. of the IEEE Int. Conf. on Acoustics,
Speech and Signal, number 1, pages 659–663.
Mazur, Z. and Łaguna, M. (2017). Assessment of instru-
mental music performance: definitions, criteria, mea-
surement. Edukacja, pages 115–128,
Molina, E., Barbancho, I., G
´
omez, E., Barbancho,
A. M., and Tard
´
on, L. J. (2013). Fundamental fre-
quency alignment vs. note-based melodic similarity
for singing voice assessment. In 2013 IEEE Int. Conf.
on Acoustics, Speech and Signal Processing, pages
744–748.
Pati, K. A., Gururani, S., and Lerch, A. (2018). Assess-
ment of student music performances using Deep Neu-
ral Networks. Applied Sciences (Switzerland), 8(4),
Pauwels, J., O’hanlon, K., G
´
omez, E., and Sandler, M. B.
(2019). 20 Years of Automatic Chord Recognition
From Audio. In Proc. of the Int. Society for Music
Information Retrieval Conference, pages 54–63.
Pellegrino, K., Conway, C. M., and Russell, J. A. (2015).
Assessment in Performance-Based Secondary Music
Classes. Music Educators Journal, 102(1):48–55,
Ramirez, R., Volpe, G., Canepa, C., Ghisio, S.,
Kolykhalova, K., Giraldo, S., Mayor, O., Perez, A.,
Mancini, M., Volta, E., Waddell, G., and Williamon,
A. (2018). Enhancing music learning with smart tech-
nologies. In ACM Int. Conf. Proceeding Series, num-
ber June, pages 1–4.
Reboursi
`
ere, L., L
¨
ahdeoja, O., Drugman, T., Dupont, S.,
Picard, C., and Riche, N. (2012). Left and right-hand
guitar playing techniques detection. In Proc. of the
Int. Conf. on New Interfaces for Musical Expression,
pages 1–4.
Romani Picas, O., Rodriguez, H. P., Dabiri, D., Tokuda,
H., Hariya, W., Oishi, K., and Serra, X. (2015). A
real-time system for measuring sound goodness in in-
strumental sounds. In Proc. of the Audio Engineering
Society Convention, volume 2, pages 1106–1111.
Ros
´
e, C. P., McLaughlin, E. A., Liu, R., and Koedinger,
K. R. (2019). Explanatory learner models: Why ma-
chine learning (alone) is not the answer. British Jour-
nal of Educational Technology, 50(6):2943–2958,
Rudin, C. (2019). Stop explaining black box machine learn-
ing models for high stakes decisions and use inter-
pretable models instead. Nature Machine Intelligence,
1(5):206–215.
Russell, B. E. (2010). The Development of a Guitar Perfor-
mance Rating Scale using a Facet-Factorial Approach.
Bulletin of the Council for Research in Music Educa-
tion, (184):21–34.
Salamon, J., G
´
omez, E., Ellis, D. P., and Richard, G. (2014).
Melody extraction from polyphonic music signals:
Approaches, applications, and challenges. IEEE Sig-
nal Processing Magazine, 31(2):118–134,
Schedl, M., G
´
omez, E., and Urbano, J. (2014). Music in-
formation retrieval: Recent developments and appli-
cations. Foundations and Trends in Information Re-
trieval, 8(2-3):127–261.
Schneider, M. C., McDonel, J. S., and DePascale, C. A.
(2019). Performance Assessment and Rubric Design,
pages 628–650.
Schramm, R., de Souza Nunes, H., and Jung, C. R. (2015).
Automatic solf
`
ege assessment. In Proc. of the Int.
Society for Music Information Retrieval Conference,
pages 183–189.
Schreiber, H. and M
¨
uller, M. (2018). A single-step ap-
proach to musical tempo estimation using a convolu-
tional neural network. In Proc. of the Int. Society for
Music Information Retrieval Conference, pages 98–
105.
Serra, X. (2011). A Multicultural Approach in Music Infor-
mation Research. In Proc. of the Int. Society for Music
Information Retrieval Conference, pages 151–156.
Tomoyasu, N., Goto, M., and Hiraga, Y. (2006). An Auto-
matic Singing Skill Evaluation Method for Unknown
Melodies Using Pitch Interval Accuracy and Vibrato
Features. In Int. Conf. on Spoken Language Process-
ing, volume 8, pages 2–4.
van den Boogaart, K. G. and Tolosana-Delgado, R. (2013).
Fundamental Concepts of Compositional Data Anal-
ysis, pages 13–50. Springer Berlin Heidelberg,
Vidwans, A., Gururani, S., Wu, C. W., Subramanian, V.,
Swaminathan, R. V., and Lerch, A. (2017). Objective
descriptors for the assessment of student music per-
formances. In Proc. of the AES Int. Conference, pages
116–123.
Wesolowski, B. C. (2012). Understanding and Developing
Rubrics for Music Performance Assessment. Music
Educators Journal, 98(3):36–42,
Wesolowski, B. C. (2014). Documenting Student Learn-
ing in Music Performance. Music Educators Journal,
101(1):77–85,
Wesolowski, B. C. and Wind, S. A. (2019). Validity, Reli-
ability, and Fairness in Music Testing. In The Oxford
handbook of assessment policy and practice in music
education (pp. 437–460).
Widmer, G. (1998). Applications of Machine Learning
to Music Research: Empirical Investigations into the
Phenomenon of Musical Expression. Machine Learn-
ing, Data Mining, and Knowledge Discovery: Meth-
ods and Applications, (March):269–293.
Wu, C.-W. and Lerch, A. (2018). Learned features for
the assessment of percussive music performances. In
Proc. of the Int. Conf. on Semantic Computing, pages
93–99.
CSME 2020 - Special Session on Computer Supported Music Education
640