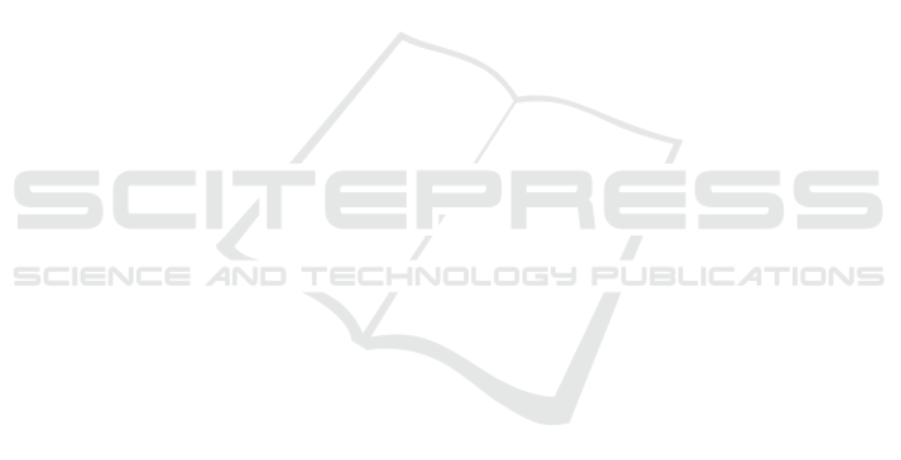
ure 4-(d) bottom), κ = 100 and l
gr
= 1 m. For the
group of friends (see Figure 4-(d) top), κ = 10 and
l
gr
= 2 m. For the first case, when the robot meets
a group of mother and son, since the spring stiffness
is very high and may cost much energy to separate
them, the robot detours from outside. However, in the
second case, when the robot meets a group of friends,
the robot passes through the group to save energy.
Switching strategy. The result for the corridor sce-
nario is showed in Figure 4-(e). All the parameters for
reactive and proactive-cooperative planner are fixed
to correctly compare the performance of these two
strategies. In real application, for reactive planning,
the robot motion should be more conservative, with
v
r
∈ (0,1] and the proxemic distance ξ
per
= 1.2 m.
For the switching parameters, the initial confidence
Con
int
= 20, Con
thres+
= 10, Con
thres+
= 7, e
thres
= 1,
lonot = 2 and losee = 1. In Figure 4-(f) on the top,
at the beginning the robot proactively communicates
with the human its willingness. During this phase, the
robot evaluates human intentions. Until 2s of simula-
tion, the confidence Con
int
is still beyond the thresh-
old Con
thres−
, thus the robot maintains a proactive and
cooperative behavior. However, in the following (see
Figure 4-(f) bottom), the real human trajectory dif-
ferentiates from the predicted one, thus Con
int
falls
below the confidence threshold Con
thres−
: the robot
switches to reactive planning to guarantee safety.
6 CONCLUSIONS
A Proactive-cooperative planning for a mobile robot
and a switching strategy to pass from a purely reac-
tive to a proactive behavior depending on the confi-
dence about human intentions is provided. Simula-
tions show the effectiveness of our method. The many
parameters introduced for modeling the human mo-
tion model in different situations are the main draw-
back of our method. Future works will be dedicated
to include some learning strategies (e.g. (Ferrer and
Sanfeliu, 2019) and (Che et al., 2020)) to determine
these parameters and automatically adapt the method
to any situation. Moreover, a simulator for taking onto
account more realistic human motions will be consid-
ered. Finally, real experiments are required to defi-
nitely validate the proposed machinery.
REFERENCES
Bevilacqua, P., Frego, M., et al. (2018). Reactive planning
for assistive robots. IEEE Robotics and Automation
Letters, 3(2):1276–1283.
Bruneau, J., Olivier, A., and Pettr
´
e, J. (2015). Going
through, going around: A study on individual avoid-
ance of groups. IEEE Trans. on Visualization and
Computer Graphics, 21(4):520–528.
Che, Y., Okamura, A. M., and Sadigh, D. (2020). Efficient
and trustworthy social navigation via explicit and im-
plicit robot–human communication. IEEE Transac-
tions on Robotics, pages 1–16.
Farina, F., Fontanelli, D., et al. (2017). Walking ahead: The
headed social force model. PLOS ONE, 12(1):1–23.
Ferrer, G. and Sanfeliu, A. (2019). Anticipative kino-
dynamic planning: Multi-objective robot navigation
in urban and dynamic environments. Autonomous
Robots, 43(6):1473–1488.
Helbing, D. and Moln
´
ar, P. (1995). Social force model for
pedestrian dynamics. Physical Review E, 51:4282–
4286.
Johansson, A., Helbing, D., and Shukla, P. K. (2007). Spec-
ification of the social force pedestrian model by evo-
lutionary adjustment to video tracking data. Advances
in Complex Systems, 10:271–288.
Khambhaita, H. and Alami, R. (2017). Assessing the social
criteria for human-robot collaborative navigation: A
comparison of human-aware navigation planners. In
Proc. IEEE ISRHIC, pages 1140–1145.
Khambhaita, H. and Alami, R. (2020). Viewing robot navi-
gation in human environment as a cooperative activity.
In Robotics Research, pages 285–300.
Mombaur, K., Truong, A., and Laumond, J.-P. (2010). From
human to humanoid locomotion—an inverse optimal
control approach. Autonomous Robots, 28(3):369–
383.
Snape, J., van den Berg, J. P., et al. (2011). The hybrid
reciprocal velocity obstacle. IEEE Transactions on
Robotics, 27:696–706.
Tamura, Y., Le, P. D., et al. (2012). Development of pedes-
trian behavior model taking account of intention. In
IEEE/RSJ IROS, pages 382–387.
Trautman, P., Ma, J., et al. (2013). Robot navigation in
dense human crowds: the case for cooperation. In
Proc. IEEE ICRA, pages 2153–2160.
Truong, X. and Ngo, T. D. (2017). Toward socially aware
robot navigation in dynamic and crowded environ-
ments: A proactive social motion model. IEEE TASE,
14(4):1743–1760.
Twitchell Hall, E. (1966). The hidden dimension. Garden
City, N.Y., Doubleday.
Zanlungo, F., Ikeda, T., and Kanda, T. (2011). Social force
model with explicit collision prediction. EPL (Euro-
physics Letters), 93:68005.
Proactive-cooperative Navigation in Human-like Environment for Autonomous Robots
419