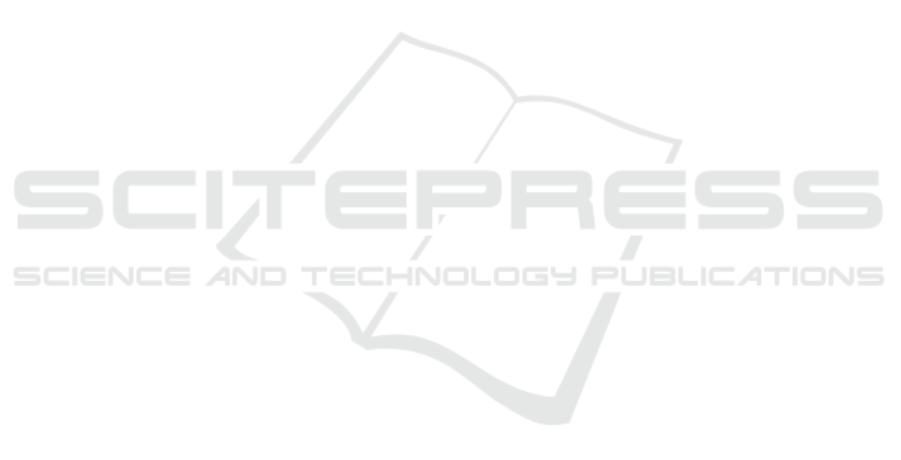
ies around vulnerabilities and weaknesses (MITRE,
2019a) (MITRE, 2019b), a knowledge bases of ad-
versary tactics and techniques (ATT&CK) (MITRE,
2020a), and a similar classification of attack pat-
terns, more focused on application security (CAPEC)
(MITRE, 2020b). Other organisations, including
NIST (NIST, 2020b) and NVD (NVD, 2020), also
maintain repositories of standards, and threat and vul-
nerability management data. There are also initiatives
around standardising the description and sharing of
cyber threat information and sources, such as STIX
(OASIS, 2020a) and TAXII (OASIS, 2020b).
These taxonomies focus on information about
system observables, such as vulnerabilities, security
events, and Indicators of Compromise (IoCs), for the
purpose of presenting and sharing specific informa-
tion that provide enhanced vulnerability and threat de-
tection and protection. In contrast, a conceptual ontol-
ogy is expressed at a higher level of abstraction and
focuses on design-level assessments of a cybersecu-
rity operation. This is similar to the use of abstrac-
tions to help thinking about risk when doing threat
modelling (Shostack, 2014). It is necessary because
IT systems and deployments are widely different to
each other and abstracting away details will provide
a better look at the big picture. Additionally, the
taxonomies focus on independent system and threat
representation, but provide no means for representing
controls and defence capabilities.
In the literature, few cybersecurity and Vulnera-
bility Management (VM) ontologies have been pro-
posed. Blanco et al. were among the first to con-
duct a review study of security ontologies (Blanco
et al., 2008). Following studies in (Fenz and Ekelhart,
2009) and (Mavroeidis and Bromander, 2017) agree
with the observations made by Blanco et al. that a
general cybersecurity ontology is yet to be defined by
the community due to a number of reasons, including
the lack of structure in the knowledge coming from
domain experts for advanced reasoning. A study by
Syed et al. (Syed and Zhong, 2018) has integrated
an intelligence element to their VM ontology, but it
has only considered Twitter data. Also, to the best
of our knowledge, none of the existing ontologies in-
cludes context as a concept, which is the main driver
of trade-offs when it comes to the interplay between
security requirements, and vulnerabilities and threats,
and their mitigation.
In (Syed et al., 2016), the authors introduced a
Unified Cybersecurity Ontology (UCO) as an exten-
sion to a previously developed Intrusion Detection
System ontology. They built UCO by semantically
linking various aspects of STIX, CVE, CCE (Martin,
2008), CVSS, CAPEC, STUCCO (Iannacone et al.,
2015), and the kill chain. The STUCCO ontology it-
self had initially incorporated data from 13 different
sources. In (Wang and Guo, 2009), the Ontology for
Vulnerability Management and Analysis (OVM) was
also built on existing standards and taxonomies such
as CVE, CWE, CPE, CVSS, and CAPEC.
For aspects more related to threat modelling, the
Attack Surface Reasoning (ASR) ontologies, pro-
posed in (Fusun et al., 2016), gives a cyber defender
the possibility to explore trade-offs between cost and
security when deciding on the composition of their
cyber defence. Ontologies created include those of
attacks, systems, defences, missions, and metrics.
The huge diversity of the theory and practice of
cybersecurity also accounts for the large variety of
underlying concepts and principles used in previous
studies, and is the main reason (and cause) of the con-
tinued effort in this area. In (Syed et al., 2016), for in-
stance, all concepts link back to the attack, and should
be more referred to as a cyber attack ontology. We
strongly believe that a common language, which ab-
stracts out low-level system observables into a set of
basic concepts is essential in developing a shared un-
derstanding of the cyber security ecosystem, and fur-
ther expand it into an ontology. This was one of our
primary motivations to propose a more comprehen-
sive foundational conceptual representation.
On the other hand, in the area of measuring and
managing information risk, various frameworks have
been proposed, some of which are ontology-based.
The FAIR institute (FAIR, 2020), for instance, pro-
poses standards, best practices, and also a risk ontol-
ogy (or rather a taxonomy). It starts from the top-
level concept risk and steps down to key factors that
derive risk in FAIR, including loss frequency, magni-
tude, and exposure, and vulnerability and threat. The
modelling is centered around quantifying the threat
factors for the risk associated with a given scenario,
while other important concepts such as controls, poli-
cies, assets, or intelligence are not explicitly defined
(Freund and Jones, 2014). Note that most risk man-
agement frameworks rely on flat terminologies and
lack the richness and flexibility that can be provided
by relationships in an ontological representation.
There are multiple other frameworks, proposed by
standard bodies and researchers, to understand, mea-
sure, and assess risk. These include CIRA (Con-
flicting Incentives Risk Analysis) (Rajbhandari, 2013;
Snekkenes, 2013), in which risks are modelled in
terms of conflicting incentives between risk owner
and other stakeholders. CIRA does not directly
conduct vulnerability and control identification, but
threats and stakeholders are at the core of the method.
Another risk framework is CORAS (Den Braber et al.,
Defender-centric Conceptual Cyber Exposure Ontology for Adaptive Cyber Risk Assessment
581