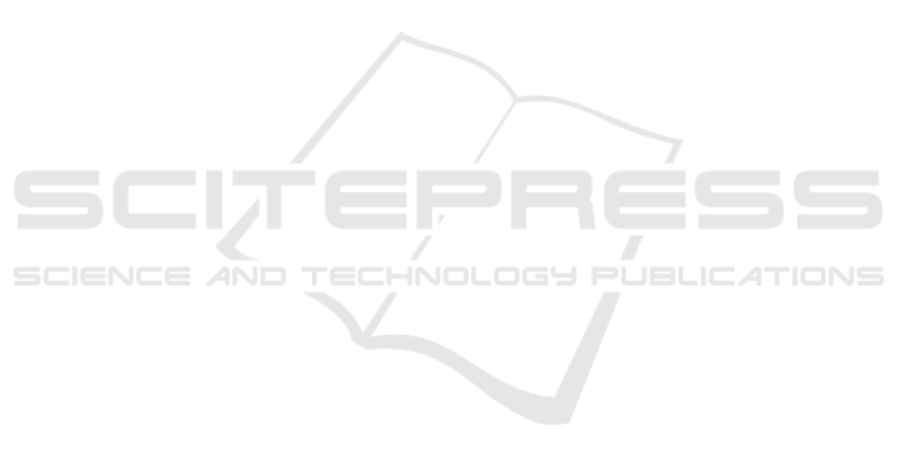
ACKNOWLEDGEMENTS
This work was partially supported by the Swedish Re-
search Council (Vetenskapsr
˚
adet) project DRIAT (VR
2016-03346), the Spanish Government under grants
RTI2018-095094-B-C22 ”CONSENT”, and the UOC
postdoctoral fellowship program.
REFERENCES
Aiello, W., Chung, F., and Lu, L. (2001). A random graph
model for power law graphs. Experimental Mathemat-
ics, 10(1):53–66.
Blocki, J., Blum, A., Datta, A., and Sheffet, O. (2013). Dif-
ferentially private data analysis of social networks via
restricted sensitivity. In Proceedings of the 4th Con-
ference on Innovations in Theoretical Computer Sci-
ence, ITCS ’13, pages 87–96.
Desfontaines, D. and Pej
´
o, B. (2019). Sok: Differential
privacies.
Dwork, C., Kenthapadi, K., McSherry, F., Mironov, I., and
Naor, M. (2006). Our data, ourselves: Privacy via
distributed noise generation. In Vaudenay, S., editor,
Advances in Cryptology - EUROCRYPT 2006, pages
486–503.
Dwork, C. and Roth, A. (2014). The algorithmic foun-
dations of differential privacy. Found. Trends Theor.
Comput. Sci., 9(3—4):211–407.
Goldberg, L. R., Johnson, J. A., Eber, H. W., Hogan,
R., Ashton, M. C., Cloninger, C. R., and Gough,
H. G. (2006). The international personality item pool
and the future of public-domain personality measures.
Journal of Research in Personality, 40(1):84 – 96.
Proceedings of the 2005 Meeting of the Association
of Research in Personality.
Harper, F. M. and Konstan, J. A. (2015). The movielens
datasets: History and context. ACM Trans. Interact.
Intell. Syst., 5(4):19:1–19:19.
Hay, M., Li, C., Miklau, G., and Jensen, D. (2009). Ac-
curate estimation of the degree distribution of private
networks. In 2009 Ninth IEEE International Confer-
ence on Data Mining, pages 169–178.
Holohan, N., Leith, D. J., and Mason, O. (2017). Optimal
differentially private mechanisms for randomised re-
sponse. IEEE Transactions on Information Forensics
and Security, 12(11):2726–2735.
Klir, G. J. and Yuan, B. (1995). Fuzzy Sets and Fuzzy Logic:
Theory and Applications. Prentice-Hall, Inc., USA.
Koren, Y., Bell, R., and Volinsky, C. (2009). Matrix factor-
ization techniques for recommender systems. Com-
puter, 42(8):30–37.
Kosinski, M., Stillwell, D., and Graepel, T. (2013). Pri-
vate traits and attributes are predictable from digital
records of human behavior. Proceedings of the Na-
tional Academy of Sciences, 110(15):5802–5805.
Kosinski, M., Wang, Y., Lakkaraju, H., and Leskovec,
J. (2016). Mining big data to extract patterns and
predict real-life outcomes. Psychological Methods,
21(4):493–506.
Leskovec, J., Rajaraman, A., and Ullman, J. D. (2014). Min-
ing of Massive Datasets. Cambridge University Press,
2 edition.
Li, N., Li, T., and Venkatasubramanian, S. (2007).
t-closeness: Privacy beyond k-anonymity and l-
diversity. In 2007 IEEE 23rd International Confer-
ence on Data Engineering, pages 106–115.
Liu, X., Liu, A., Zhang, X., Li, Z., Liu, G., Zhao, L., and
Zhou, X. (2017). When differential privacy meets ran-
domized perturbation: A hybrid approach for privacy-
preserving recommender system. In Database Sys-
tems for Advanced Applications, pages 576–591.
Machanavajjhala, A., Kifer, D., Gehrke, J., and Venkitasub-
ramaniam, M. (2007). L-diversity: Privacy beyond
k-anonymity. ACM Trans. Knowl. Discov. Data, 1(1).
Mironov, I. and McSherry, F. (2009). Differentially pri-
vate recommender systems: Building privacy into the
netflix prize contenders. In Proceedings of the 15th
ACM SIGKDD International Conference on Knowl-
edge Discovery and Data Mining (KDD), pages 627–
636.
Narayanan, A. and Shmatikov, V. (2008). Robust de-
anonymization of large sparse datasets. In 2008 IEEE
Symposium on Security and Privacy (sp 2008), pages
111–125.
Polat, H. and Du, W. (2006). Achieving private recom-
mendations using randomized response techniques. In
Advances in Knowledge Discovery and Data Mining,
pages 637–646.
Salas, J. (2019). Sanitizing and measuring privacy of large
sparse datasets for recommender systems. Journal of
Ambient Intelligence and Humanized Computing.
Salas, J. and Domingo-Ferrer, J. (2018). Some basics
on privacy techniques, anonymization and their big
data challenges. Mathematics in Computer Science,
12(3):263–274.
Samarati, P. (2001). Protecting respondents identities in mi-
crodata release. IEEE Transactions on Knowledge and
Data Engineering, 13(6):1010–1027.
Sweeney, L. (2002). k-anonymity: A model for protecting
privacy. International Journal of Uncertainty, Fuzzi-
ness and Knowledge-Based Systems, 10(05):557–570.
Torra, V. (2017). Data privacy: Foundations, new develop-
ments and the big data challenge. Springer.
Torra, V. and Salas, J. (2019). Graph perturbation as
noise graph addition: A new perspective for graph
anonymization. In Data Privacy Management, Cryp-
tocurrencies and Blockchain Technology, pages 121–
137.
Wang, Y., Wu, X., and Hu, D. (2016). Using randomized
response for differential privacy preserving data col-
lection. In EDBT/ICDT2016WS.
Warner, S. L. (1965). Randomized response: A survey tech-
nique for eliminating evasive answer bias. Journal of
the American Statistical Association, 60(309):63–69.
SECRYPT 2020 - 17th International Conference on Security and Cryptography
422