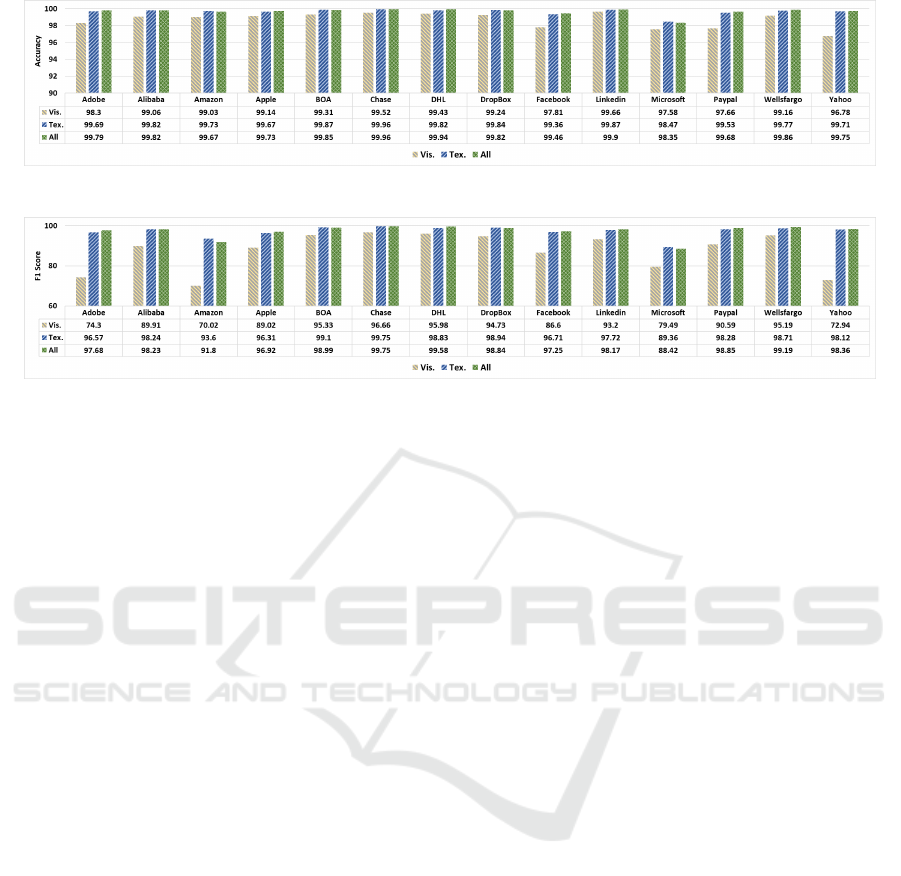
Figure 4: Accuracy of trained model for targeted websites.
Figure 5: F1 score for trained model for targeted websites.
is not biased towards more popular websites and can
be adapted for new attacks. We demonstrated our ap-
proach on 14 different target websites with varying
popularity. Our model achieved an accuracy of 99%
for all of them with cross-validated data. Further-
more, we employed a one-vs-all technique and cre-
ated an imbalanced dataset; we reported an accuracy
of more than 98% among all of the websites, which
is surprisingly high. It may possible that through ad-
versarial machine learning attackers generate phish-
ing samples that match the fingerprint of legitimate
websites. Our future work will investigate how to pro-
tect against such attacks.
REFERENCES
Abdelhamid, N., Ayesh, A., and Thabtah, F. (2014). Phish-
ing Detection Based Associative Classification Data
Mining. Expert Systems with Applications, 41(13).
Alexa (2020). Competitive Analysis, Marketing Mix and
Traffic.
Cui, Q., Jourdan, G.-V., Bochmann, G. V., Couturier, R.,
and Onut, I.-V. (2017). Tracking Phishing Attacks
Over Time. In Proc. of IW3C2.
Cui, Q., Jourdan, G.-V., Bochmann, G. V., Onut, I.-V., and
Flood, J. (2018). Phishing Attacks Modifications and
Evolutions. In Proc. of ESORICS.
Dalgic, F. C., Bozkir, A. S., and Aydos, M. (2018). Phish-
iris: A new approach for vision based brand prediction
of phishing web pages via compact visual descriptors.
In Proc. of ISMSIT.
Gutierrez, C. N., Kim, T., Della Corte, R., Avery, J., Gold-
wasser, D., Cinque, M., and Bagchi, S. (2018). Learn-
ing from the Ones That Got Away: Detecting New
Forms of Phishing Attacks. IEEE Transactions on De-
pendable and Secure Computing, 15(6).
Ho, G., Cidon, A., Gavish, L., Schweighauser, M., Pax-
son, V., Savage, S., Voelker, G. M., and Wagner, D.
(2019). Detecting and Characterizing Lateral Phish-
ing at Scale. In Proc. of USENIX.
Jain, A. K. and Gupta, B. B. (2018). Towards detec-
tion of phishing websites on client-side using machine
learning based approach. Telecommunication Systems,
68(4).
Ma, E. (2019). Secret of Google Web-Based OCR Service.
Marchal, S., Franc¸ois, J., Engel, T., et al. (2012). Proac-
tive Discovery of Phishing Related Domain Names.
In RAID.
Mohammad, R. M., Thabtah, F., and McCluskey, L. (2012).
An Assessment of Features Related to Phishing Web-
sites Using an Automated Technique. In Proc. of IC-
ITST.
Pedregosa, F., Varoquaux, G., Gramfort, A., Michel, V.,
Thirion, B., Grisel, O., Blondel, M., Prettenhofer, P.,
Weiss, R., Dubourg, V., Vanderplas, J., Passos, A.,
Cournapeau, D., Brucher, M., Perrot, M., and Duch-
esnay, E. (2011). Scikit-learn: Machine Learning in
Python. Journal of Machine Learning Research, 12.
Shirazi, H., Bezawada, B., and Ray, I. (2018). “Kn0w Thy
Doma1n Name”: Unbiased Phishing Detection Using
Domain Name Based Features. In Proc. of SACMAT.
Shirazi, H., Bezawada, B., Ray, I., and Anderson, C. (2019).
Adversarial Sampling Attacks Against Phishing De-
tection. In Proc. of DBSec.
Tan, C. L. (2018). Phishing Dataset for Machine Learning:
Feature Evaluation.
Van Der Heijden, A. and Allodi, L. (2019). Cognitive Triag-
ing of Phishing Attacks. In USENIX.
Zou, J. and Schiebinger, L. (2018). AI Can Be Sexist and
Racist—It’s Time to Make It Fair.
SECRYPT 2020 - 17th International Conference on Security and Cryptography
430