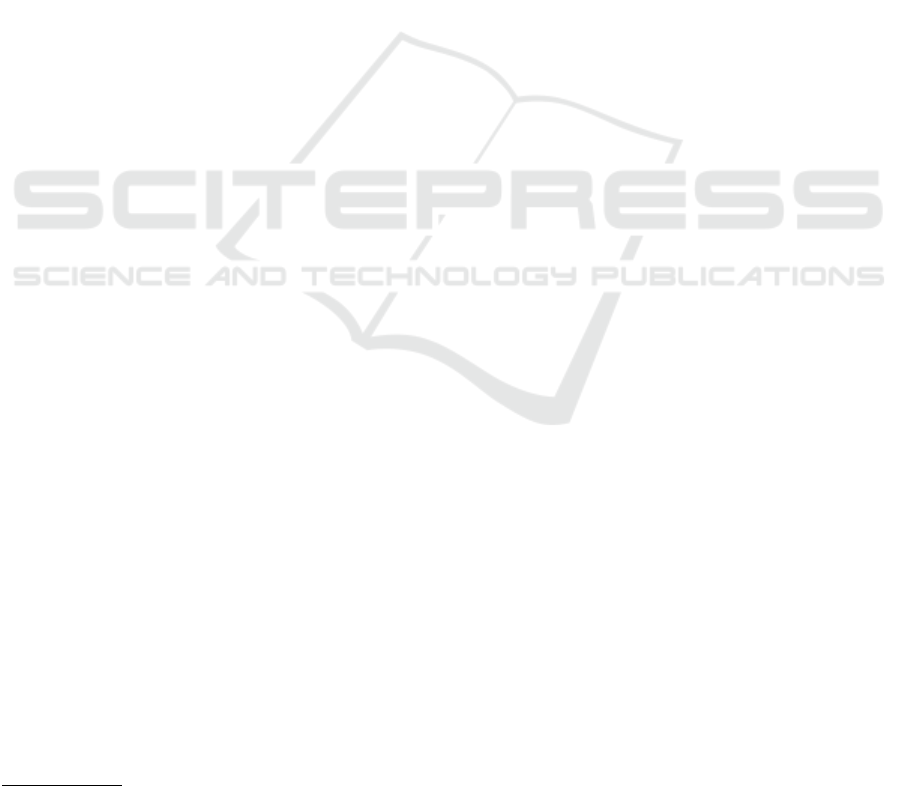
ers and other objects that will be incorporated on the
database.
We also intend to address other industry problems,
such as the verification of the load from the extin-
guisher, and if its labeling panel is preserved and read-
able. More up-to-date topologies will also be tested,
aiming to obtain better results for mobile devices.
Further results will be reported eventually.
ACKNOWLEDGEMENTS
The authors would like to thank the Edge laboratory
1
at the Federal University of Alagoas
2
which funded
this research through an agreement regulated by the
Information Technology Law.
REFERENCES
Aggarwal, C. C. (2018). Neural Networks and Deep Learn-
ing. Springer International Publishing, 1 edition. 1
Chen, L.-C., Papandreou, G., Kokkinos, I., Murphy, K., and
Yuille, A. L. (2017). Deeplab: Semantic image seg-
mentation with deep convolutional nets, atrous convo-
lution, and fully connected crfs. IEEE transactions on
pattern analysis and machine intelligence, 40(4):834–
848. 4
Choi, Y., Park, J.-H., and Jang, B. (2019). A risk estima-
tion approach based on deep learning in shipbuilding
industry. In 2019 International Conference on Infor-
mation and Communication Technology Convergence
(ICTC), pages 1438–1441. IEEE. 2
Chou, Y.-C., Kuo, C.-J., Chen, T.-T., Horng, G.-J., Pai, M.-
Y., Wu, M.-E., Lin, Y.-C., Hung, M.-H., Su, W.-T.,
Chen, Y.-C., et al. (2019). Deep-learning-based de-
fective bean inspection with gan-structured automated
labeled data augmentation in coffee industry. Applied
Sciences, 9(19):4166. 2
Dargan, S., Kumar, M., Ayyagari, M. R., and Kumar, G.
(2019). A Survey of Deep Learning and Its Ap-
plications: A New Paradigm to Machine Learning.
Archives of Computational Methods in Engineering.
1
Hocenski,
ˇ
Z., Mati
´
c, T., and Vidovi
´
c, I. (2016). Technol-
ogy transfer of computer vision defect detection to ce-
ramic tiles industry. In 2016 International Conference
on Smart Systems and Technologies (SST), pages 301–
305. IEEE. 1
Huang, J., Rathod, V., Sun, C., Zhu, M., Korattikara, A.,
Fathi, A., Fischer, I., Wojna, Z., Song, Y., Guadar-
rama, S., et al. (2017). Speed/accuracy trade-offs for
modern convolutional object detectors. In Proceed-
ings of the IEEE conference on computer vision and
pattern recognition, pages 7310–7311. 4
1
http://edgebr.org/
2
https://ufal.br/
Li, L., Ota, K., and Dong, M. (2018). Deep learning for
smart industry: Efficient manufacture inspection sys-
tem with fog computing. IEEE Transactions on In-
dustrial Informatics, 14(10):4665–4673. 2
Lin, T.-Y., Doll
´
ar, P., Girshick, R., He, K., Hariharan, B.,
and Belongie, S. (2017). Feature pyramid networks
for object detection. In Proceedings of the IEEE con-
ference on computer vision and pattern recognition,
pages 2117–2125. 3
Lin, T.-Y., Maire, M., Belongie, S., Hays, J., Perona, P.,
Ramanan, D., Doll
´
ar, P., and Zitnick, C. L. (2014).
Microsoft coco: Common objects in context. In Euro-
pean conference on computer vision, pages 740–755.
Springer. 4
Liu, W., Anguelov, D., Erhan, D., Szegedy, C., Reed, S.,
Fu, C.-Y., and Berg, A. C. (2016). Ssd: Single shot
multibox detector. In European conference on com-
puter vision, pages 21–37. Springer. 3
Rao, Q. and Frtunikj, J. (2018). Deep learning for self-
driving cars: chances and challenges. In Proceedings
of the 1st International Workshop on Software Engi-
neering for AI in Autonomous Systems, pages 35–38.
2
Sandler, M., Howard, A., Zhu, M., Zhmoginov, A., and
Chen, L.-C. (2018). Mobilenetv2: Inverted residu-
als and linear bottlenecks. In Proceedings of the IEEE
conference on computer vision and pattern recogni-
tion, pages 4510–4520. 3
Szegedy, C., Ioffe, S., Vanhoucke, V., and Alemi, A. A.
(2017). Inception-v4, inception-resnet and the impact
of residual connections on learning. In Thirty-first
AAAI conference on artificial intelligence. 3, 4
Veeraraghavan, A., Adithya, V., Bhave, A., and Akella, S.
(2017). Battery aging estimation with deep learning.
In 2017 IEEE Transportation Electrification Confer-
ence (ITEC-India), pages 1–4. IEEE. 2
Zhang, N., Liu, Y., Zou, L., Zhao, H., Dong, W., Zhou, H.,
Zhou, H., and Huang, M. (2018). Automatic recog-
nition of oil industry facilities based on deep learning.
In IGARSS 2018-2018 IEEE International Geoscience
and Remote Sensing Symposium, pages 2519–2522.
IEEE. 2
Visual Inspection of Collective Protection Equipment Conditions with Mobile Deep Learning Models
83