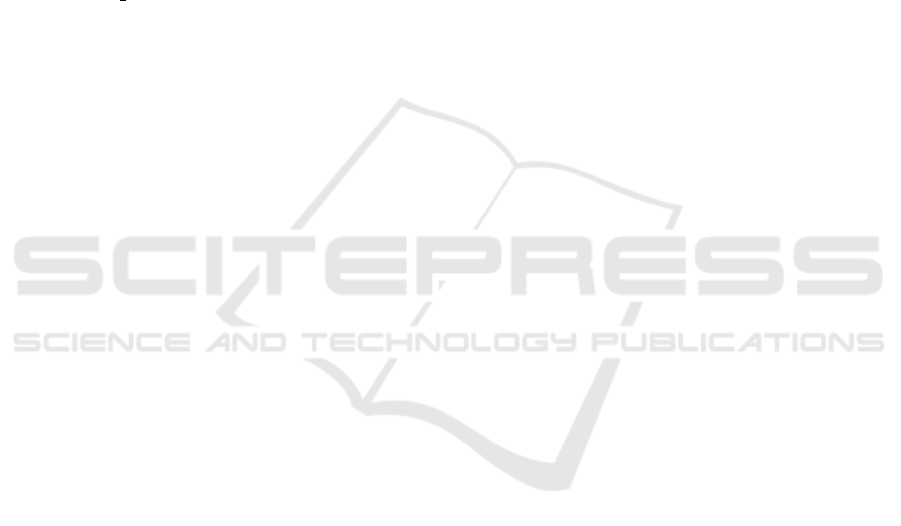
• Broaden the work done for the classification of
type of diabetes in other medical areas as well;
• Consider using a NO-SQL database(like Mon-
goDb) to manage the many data and features made
available from an electronic medical record;
ACKNOWLEDGEMENTS
The research presented in this paper was co-funded
by the activities of Gesan s.r.l. within the Tabl-
Health Project (CUP: B49J17000710008). This re-
search was also partially funded by the project ”At-
trazione e Mobilit
`
a dei Ricercatori” Italian PON Pro-
gramme (PON AIM 2018 num. AIM1878214-2).
REFERENCES
Alssema, M., Vistisen, D., Heymans, M., Nijpels, G.,
Gl
¨
umer, C., Zimmet, P. Z., Shaw, J. E., Eliasson, M.,
Stehouwer, C. D., Tab
´
ak, A. G., et al. (2011). The
evaluation of screening and early detection strategies
for type 2 diabetes and impaired glucose tolerance
(detect-2) update of the finnish diabetes risk score for
prediction of incident type 2 diabetes. Diabetologia,
54(5):1004–1012.
Bennetts, C. J., Owings, T. M., Erdemir, A., Botek, G.,
and Cavanagh, P. R. (2013). Clustering and classifi-
cation of regional peak plantar pressures of diabetic
feet. Journal of biomechanics, 46(1):19–25.
Brunese, L., Mercaldo, F., Reginelli, A., and Santone, A.
(2019). Neural networks for lung cancer detection
through radiomic features. In 2019 International Joint
Conference on Neural Networks (IJCNN), pages 1–10.
IEEE.
Brunese, L., Mercaldo, F., Reginelli, A., and Santone, A.
(2020). An ensemble learning approach for brain can-
cer detection exploiting radiomic features. Computer
methods and programs in biomedicine, 185:105134.
Contreras, I. and Veh
´
ı, J. (2018). Artificial intelligence for
diabetes management and decision support: Literature
review. Journal of Medical Internet Research, 20.
Coronato, A. and Naeem, M. (2019). A reinforcement
learning based intelligent system for the healthcare
treatment assistance of patients with disabilities. In
International Symposium on Pervasive Systems, Algo-
rithms and Networks, pages 15–28. Springer.
Dankwa-Mullan, I., Rivo, M., Sepulveda, M., Park, Y.,
Snowdon, J., and Rhee, K. (2019). Transforming dia-
betes care through artificial intelligence: the future is
here. Population health management, 22(3):229–242.
Fiorini, N., Lipman, D. J., and Lu, Z. (2017). Cutting edge:
towards pubmed 2.0. Elife, 6:e28801.
Hossin, M. and Sulaiman, M. (2015). A review on evalua-
tion metrics for data classification evaluations. Inter-
national Journal of Data Mining & Knowledge Man-
agement Process, 5(2):1.
IDF, I. (2017). International diabetes federation. idf dia-
betes atlas, 8th edn. brussels, belgium: International
diabetes federation, 2017.
Mercaldo, F., Nardone, V., and Santone, A. (2017). Dia-
betes mellitus affected patients classification and diag-
nosis through machine learning techniques. Procedia
computer science, 112:2519–2528.
Meyfroidt, G., G
¨
uiza, F., Ramon, J., and Bruynooghe, M.
(2009). Machine learning techniques to examine large
patient databases. Best Practice & Research Clinical
Anaesthesiology, 23(1):127–143.
Nanda, S., Savvidou, M., Syngelaki, A., Akolekar, R., and
Nicolaides, K. H. (2011). Prediction of gestational
diabetes mellitus by maternal factors and biomarkers
at 11 to 13 weeks. Prenatal diagnosis, 31(2):135–141.
Osman, A. H. and Aljahdali, H. M. (2017). Diabetes disease
diagnosis method based on feature extraction using k-
svm. Int J Adv Comput Sci Appl, 8(1).
Patterson, C. C., Karuranga, S., Salpea, P., Saeedi, P.,
Dahlquist, G., Soltesz, G., and Ogle, G. D. (2019).
Worldwide estimates of incidence, prevalence and
mortality of type 1 diabetes in children and adoles-
cents: Results from the international diabetes feder-
ation diabetes atlas. Diabetes Research and Clinical
Practice, 157:107842.
Ricciardi, C., Cantoni, V., Improta, G., Iuppariello, L.,
Latessa, I., Cesarelli, M., Triassi, M., and Cuocolo, A.
(2020a). Application of data mining in a cohort of ital-
ian subjects undergoing myocardial perfusion imaging
at an academic medical center. Computer Methods
and Programs in Biomedicine, page 105343.
Ricciardi, C., Edmunds, K. J., Recenti, M., Sigurdsson, S.,
Gudnason, V., Carraro, U., and Gargiulo, P. (2020b).
Assessing cardiovascular risks from a mid-thigh ct im-
age: a tree-based machine learning approach using
radiodensitometric distributions. Scientific Reports,
10(1):1–13.
Romeo, V., Cuocolo, R., Ricciardi, C., Ugga, L., Cocozza,
S., Verde, F., Stanzione, A., Napolitano, V., Russo, D.,
Improta, G., et al. (2020). Prediction of tumor grade
and nodal status in oropharyngeal and oral cavity
squamous-cell carcinoma using a radiomic approach.
Anticancer Research, 40(1):271–280.
Sahin, H. and Subasi, A. (2015). Classification of the car-
diotocogram data for anticipation of fetal risks using
machine learning techniques. Applied Soft Comput-
ing, 33:231–238.
Verde, L. and De Pietro, G. (2018). A machine learning ap-
proach for carotid diseases using heart rate variability
features. In HEALTHINF, pages 658–664.
Verde, L., De Pietro, G., and Sannino, G. (2018). Voice dis-
order identification by using machine learning tech-
niques. IEEE Access, 6:16246–16255.
Zheng, T., Xie, W., Xu, L., He, X., Zhang, Y., You, M.,
Yang, G., and Chen, Y. (2017). A machine learning-
based framework to identify type 2 diabetes through
electronic health records. International journal of
medical informatics, 97:120–127.
Zhou, H. and Myrzashova, R. (2020). Ai based systems for
diabetes treatment: a brief overview of the past and
plans for the future. Journal of Physics: Conference
Series, 1453:012063.
AI4EIoTs 2020 - Special Session on Artificial Intelligence for Emerging IoT Systems: Open Challenges and Novel Perspectives
540