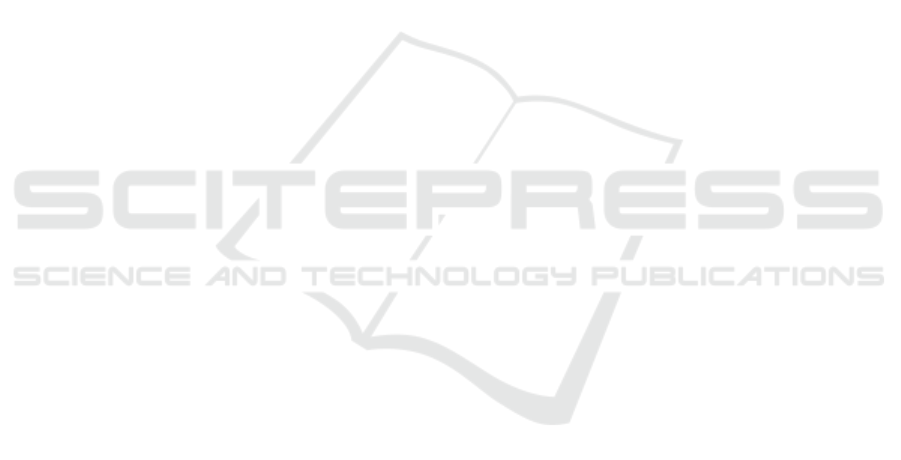
late temperature distributions over time in 2 or 3 di-
mensional problems. Since large system scales can
arise from finite element analysis, model order re-
duction is applied to reduce computation time. This
computation-efficient description is required for solv-
ing optimization problems with a high amount of iter-
ations or meeting real-time demands. However, basic
model order reduction methods are only valid for lin-
ear models. If the system can not be linearized prop-
erly, temperature dependent boundary conditions as
well as parameter uncertainties have to be accounted
for. Parametric reduction algorithms are either based
on system snapshots or entail higher reduced orders
and larger projection matrices. Thus, a method to pre-
serve physically interpretable parameters, while using
rational Krylov model order reduction algorithms is
proposed. This is especially applicable for small vari-
ations around a well defined initial operating point.
Hence, neither a validation of the full order model be-
fore formulating the reduced model is required nor
many time consuming experiments to get measure-
ment data. Instead system parameters are identi-
fied and validated with a reduced system formulation.
Moreover, temperature dependencies during the pro-
cess can be modeled and a parameterizable balancing
between computation time and accuracy is possible.
Thus, online process adaptions according to (stochas-
tic) parameter variations are possible without costly
recalculation of model order reduction. The approach
is demonstrated for two sample systems, which mate-
rial and heat transition parameters are identified with
reduced-order models. Therefore, particle swarm op-
timization can be used to find the global minimum of
a formulated cost-function. Moreover, computation
times are within real-time restrictions and thus, pre-
sented models are used for model-based temperature
control, process predictions and state estimation.
REFERENCES
Aleksendri
´
c, D., Carlone, P., and
´
Cirovi
´
c, V. (2016). Op-
timization of the temperature-time curve for the cur-
ing process of thermoset matrix composites. Applied
Composite Materials, 23(5):1047–1063.
Astrid, P. (2004). Reduction of process simulation models
: a proper orthogonal decomposition approach. PhD
thesis, Department of Electrical Engineering.
Beattie, C., Gugercin, S., and Mehrmann, V. (2017). Model
reduction for systems with inhomogeneous initial con-
ditions. Systems & Control Letters, 99:99 – 106.
Benner, P., Gugercin, S., and Willcox, K. (2015). A
survey of projection-based model reduction methods
for parametric dynamical systems. SIAM Review,
57(4):483–531.
Benner, P., Herzog, R., Lang, N., Riedel, I., and Saak, J.
(2019). Comparison of model order reduction meth-
ods for optimal sensor placement for thermo-elastic
models. Engineering Optimization, 51(3):465–483.
Bosselmann, S., Frank, T., Wielitzka, M., Dagen, M., and
Ortmaier, T. (2017). Thermal modeling and decen-
tralized control of mold temperature for a vulcaniza-
tion test bench. In 2017 IEEE Conference on Control
Technology and Applications (CCTA), pages 377–382.
Bosselmann, S., Frank, T., Wielitzka, M., and Ortmaier, T.
(2018). Optimization of process parameters for rub-
ber curing in relation to vulcanization requirements
and energy consumption. In 2018 IEEE/ASME Inter-
national Conference on Advanced Intelligent Mecha-
tronics (AIM), pages 804–809.
Bruzelius, F. (2004). Linear parameter-varying systems: An
approach to gain scheduling: Zugl.: Goteborg, Univ.,
Diss., 2004. PhD thesis, School of Electrical Engi-
neering.
Chaturantabut, S. and Sorensen, D. C. (2010). Nonlinear
model reduction via discrete empirical interpolation.
SIAM Journal on Scientific Computing, 32(5):2737–
2764.
Feng, L., Yue, Y., Banagaaya, N., Meuris, P., Schoenmaker,
W., and Benner, P. (2016). Parametric modeling and
model order reduction for (electro-)thermal analysis
of nanoelectronic structures. Journal of Mathematics
in Industry, 6(1):10.
Frank, T., Bosselmann, S., Wielitzka, M., and Ortmaier, T.
(2018). Computation-efficient simulation of nonlinear
thermal boundary conditions for large-scale models.
IEEE Control Systems Letters, 2(3):351–356.
Frank, T., Wieting, S., Wielitzka, M., Bosselmann, S., and
Ortmaier, T. (2019). Identification of temperature-
dependent boundary conditions using mor. Interna-
tional Journal of Numerical Methods for Heat & Fluid
Flow, ahead-of-print.
Grimme, E. (1997). Krylov Projection Methods for Model
Reduction. PhD thesis, Department of Electrical and
Computer Engineering.
Huang, H.-C. and Usmani, A. S. (1994). Finite Element
Analysis for Heat Transfer. Springer London, London.
Li, H.-X. and Qi, C. (2010). Modeling of distributed pa-
rameter systems for applications - a synthesized re-
view from time & space separation. Journal of Pro-
cess Control, 20(8):891 – 901.
Speicher, K., Steinboeck, A., Kugi, A., Wild, D., and
Kiefer, T. (2014). Analysis and design of an extended
kalman filter for the plate temperature in heavy plate
rolling. Journal of Process Control, 24(9):1371 –
1381.
Sun, C. and Hahn, J. (2006). Model reduction in the pres-
ence of uncertainty in model parameters. Journal of
Process Control, 16(6):645 – 649.
Wang, D.-h., Dong, Q., and Jia, Y.-x. (2015). Mathemat-
ical modelling and numerical simulation of the non-
isothermal in-mold vulcanization of natural rubber.
Chinese Journal of Polymer Science, 33(3):395–403.
Yuan, C. D., Rudnyi, E. B., Baumgartl, H., and Bechtold,
T. (2017). Model order reduction and system simula-
tion of a machine tool for real-time compensation of
thermally induced deformations. In 2017 IEEE Inter-
national Conference on Advanced Intelligent Mecha-
tronics (AIM), pages 1071–1076.
ICINCO 2020 - 17th International Conference on Informatics in Control, Automation and Robotics
666